Effective sample pairs based contrastive learning for clustering
Information Fusion(2023)
摘要
As an indispensable branch of unsupervised learning, deep clustering is rapidly emerging along with the growth of deep neural networks. Recently, contrastive learning paradigm has been combined with deep clustering to achieve more competitive performance. However, previous works mostly employ random augmentations to construct sample pairs for contrastive clustering. Different augmentations of a sample are treated as positive sample pairs, which may result in false positives and ignore the semantic variations of different samples. To address these limitations, we present a novel end-to-end contrastive clustering framework termed Contrastive Clustering with Effective Sample pairs construction (CCES), which obtains more semantic information by jointly leveraging an effective data augmentation method ContrastiveCrop and constructing positive sample pairs based on nearest-neighbor mining. Specifically, we augment original samples by adopting ContrastiveCrop, which explicitly reduces false positives and enlarges the variance of samples. Further, with the extracted feature representations, we provide a strategy to construct positive sample pairs via a sample and its nearest neighbor for instance-wise and cluster-wise contrastive learning. Experimental results on four challenging datasets demonstrate the effectiveness of CCES for clustering, which surpasses the state-of-the-art deep clustering methods.
更多查看译文
关键词
Representation learning,Contrastive learning,Deep clustering,Nearest neighbor
AI 理解论文
溯源树
样例
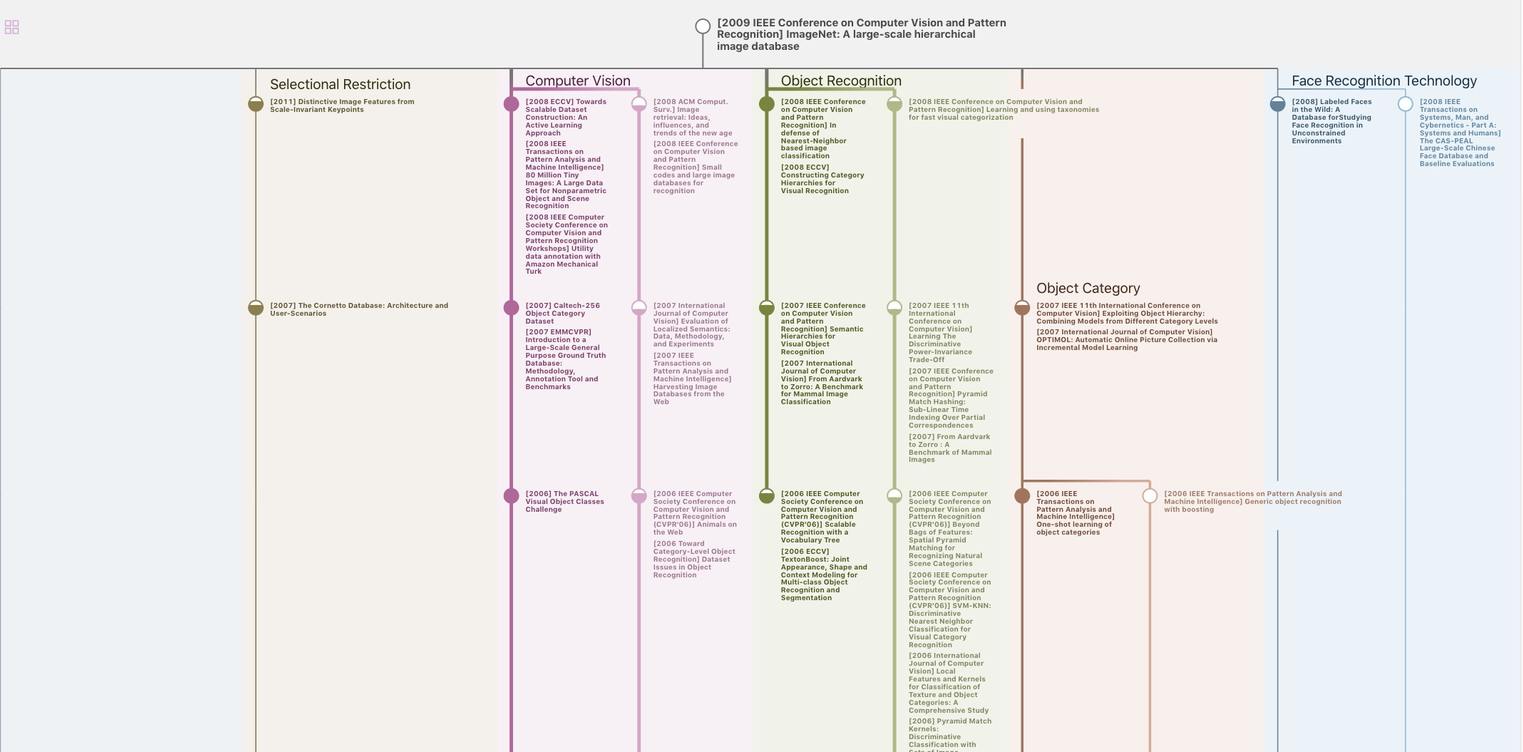
生成溯源树,研究论文发展脉络
Chat Paper
正在生成论文摘要