Monitoring of multivariate calibration models in the absence of new reference values: The regression case
Chemometrics and Intelligent Laboratory Systems(2023)
摘要
Multivariate calibration models are one of the most predominant tools in analytical chemistry for the non-destructive quantification of constituents. While pipelines, methods, and software are widely available for training calibration models, the monitoring strategies to track the prediction performance in time are still limited and are one of the emerging research topics in chemometrics and machine learning. Moreover, for the sake of efficiency, the development of modern monitoring strategies requires tools that do not require new reference values at each time point. The current study addresses the problem of monitoring calibration models for regression. We present a monitoring paradigm and propose two methods to monitor the performance of the model. The two methods focus on bias drift and spectral variation drift. We present the derivation of the methods and their application to two real case studies from the agrofood industry involving milk composition and apple harvesting. Conclusions and recommendations are presented for practitioners, as well as views for future work in this domain of research.
更多查看译文
关键词
Multivariate calibration,Calibration monitoring,Bias drift,Spectral variation drift,Unsupervised monitoring
AI 理解论文
溯源树
样例
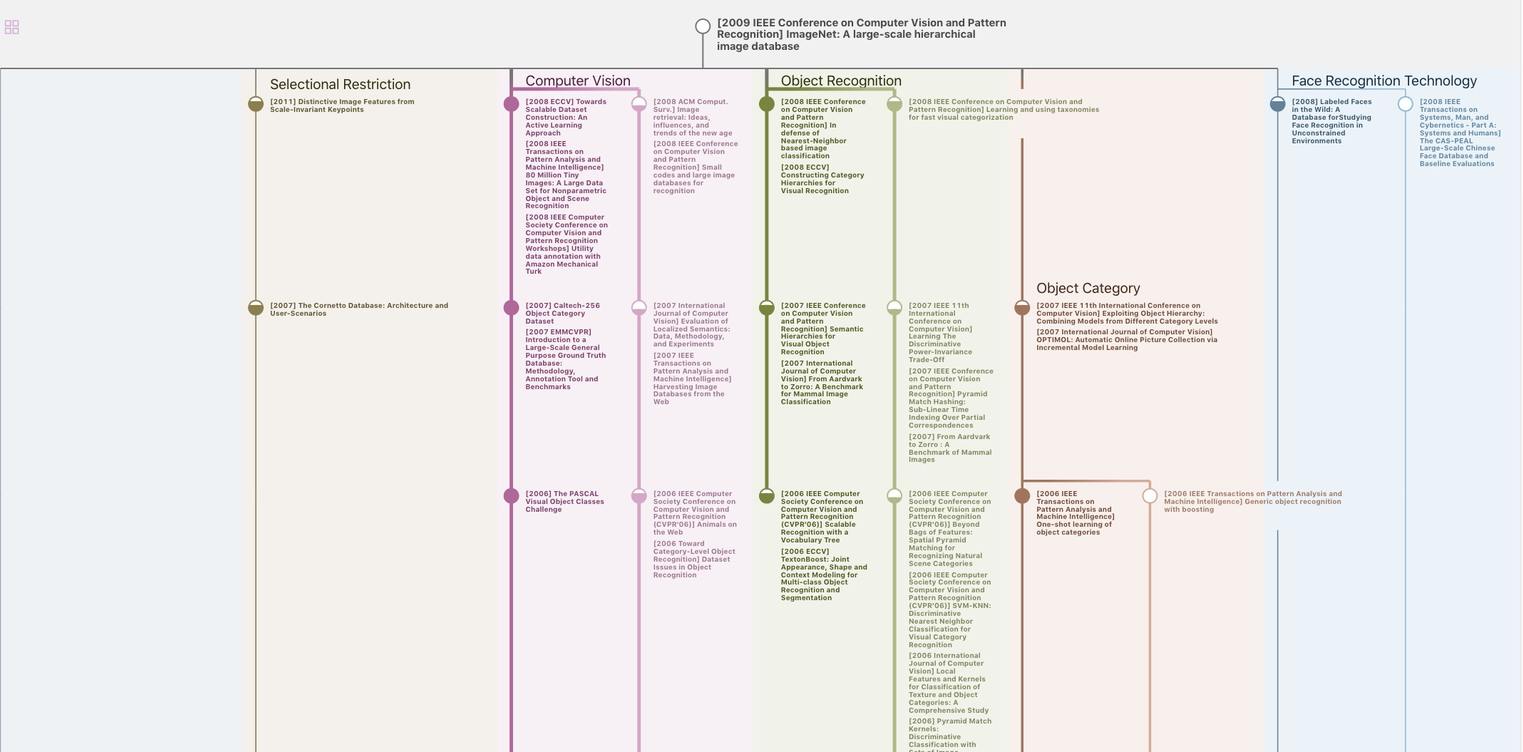
生成溯源树,研究论文发展脉络
Chat Paper
正在生成论文摘要