Exploratory mean-variance portfolio selection with Choquet regularizers
arXiv (Cornell University)(2023)
摘要
In this paper, we study a continuous-time exploratory mean-variance (EMV) problem under the framework of reinforcement learning (RL), and the Choquet regularizers are used to measure the level of exploration. By applying the classical Bellman principle of optimality, the Hamilton-Jacobi-Bellman equation of the EMV problem is derived and solved explicitly via maximizing statically a mean-variance constrained Choquet regularizer. In particular, the optimal distributions form a location-scale family, whose shape depends on the choices of the Choquet regularizer. We further reformulate the continuous-time Choquet-regularized EMV problem using a variant of the Choquet regularizer. Several examples are given under specific Choquet regularizers that generate broadly used exploratory samplers such as exponential, uniform and Gaussian. Finally, we design a RL algorithm to simulate and compare results under the two different forms of regularizers.
更多查看译文
关键词
selection,mean-variance
AI 理解论文
溯源树
样例
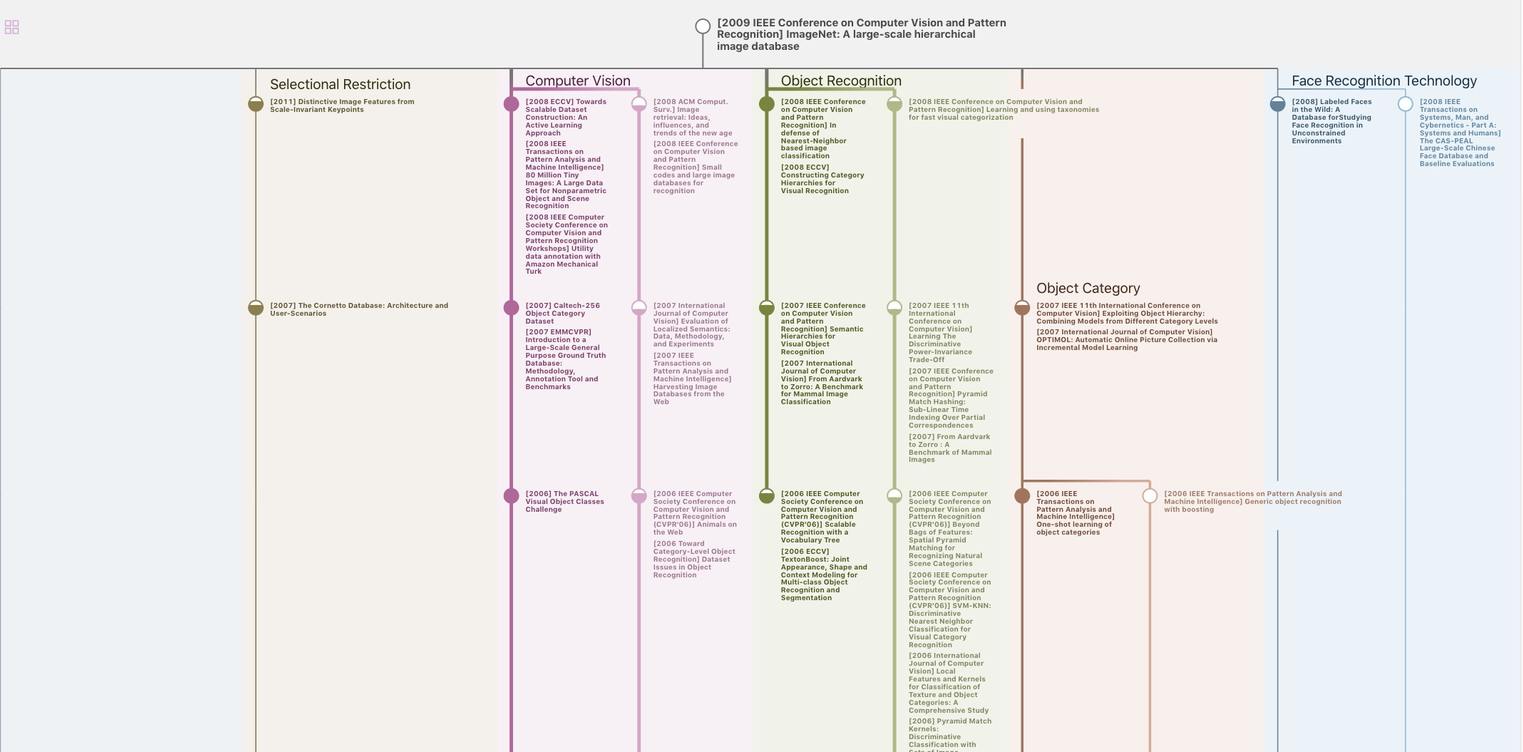
生成溯源树,研究论文发展脉络
Chat Paper
正在生成论文摘要