Does optimal estimation perform adequately for hyperspectral surface reflectance retrieval?
crossref(2020)
摘要
<p>The current and coming imaging spectroscopy missions (EMIT, ECOSTRESS, AVIRIS-NG), and observables for potential future missions studying Surface Biology and Geology (SBG) observe a wide range of spectral bands, which can be used to infer about surface properties. The current state of the art approach for performing the retrieval of surface reflectance is optimal estimation (OE), which amounts to finding the maximum a posteriori estimate of the surface reflectance, after which the posterior covariance is approximated by linearizing the forward model (Rodgers, 2001). While this method has a principled basis and often performs well, with challenging atmospheres the optimization may fall into local minima, or the estimated posterior mean and covariance may be wrong.  Addressing these failures under realistic observing conditions is particularly important to realize the full potential of upcoming global observations.                                                                                                                                                                        </p><p><br>As a preparation to improving the quality of future retrievals, we evaluate the performance of OE against posteriors generated with advanced Bayesian techniques.  We present results from comparing the OE posterior mean and covariance to the true posterior, as computed by MCMC, for moderately challenging atmospheric conditions, and an instrument configuration consistent with AVIRIS-NG. </p>
更多查看译文
AI 理解论文
溯源树
样例
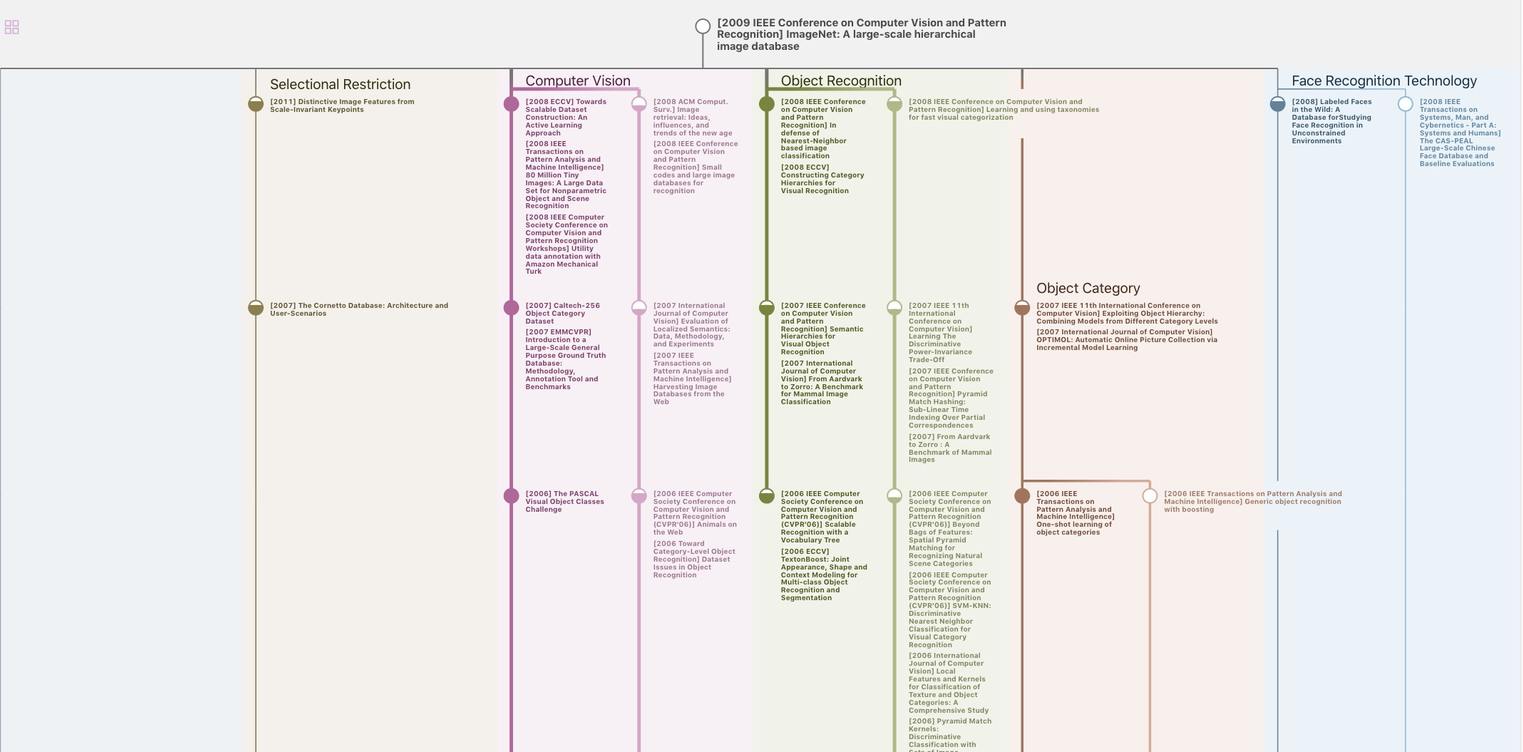
生成溯源树,研究论文发展脉络
Chat Paper
正在生成论文摘要