Boosting performance in Machine Learning of Turbulent and Geophysical Flows via scale separation
crossref(2020)
摘要
<p>Recent advances in statistical learning have opened the possibility to forecast the behavior of chaotic systems using recurrent neural networks. In this letter we investigate the applicability of this framework to geophysical flows, known to be intermittent and turbulent.  We show that both turbulence and intermittency introduce severe limitations on the applicability of recurrent neural networks, both for short term forecasts as well as for the reconstruction of the underlying attractor. We test these ideas on global sea-level pressure data for the past 40 years, issued from the NCEP reanalysis datase, a proxy of the atmospheric circulation dynamics.  The performance of recurrent neural network in predicting both short and long term behaviors rapidly drops when the systems are perturbed with noise. However, we found that a good predictability is partially recovered when scale separation is performed via a moving average filter. We suggest that possible strategies to overcome limitations  should be based on separating the smooth large-scale dynamics, from the intermittent/turbulent features. </p>
更多查看译文
AI 理解论文
溯源树
样例
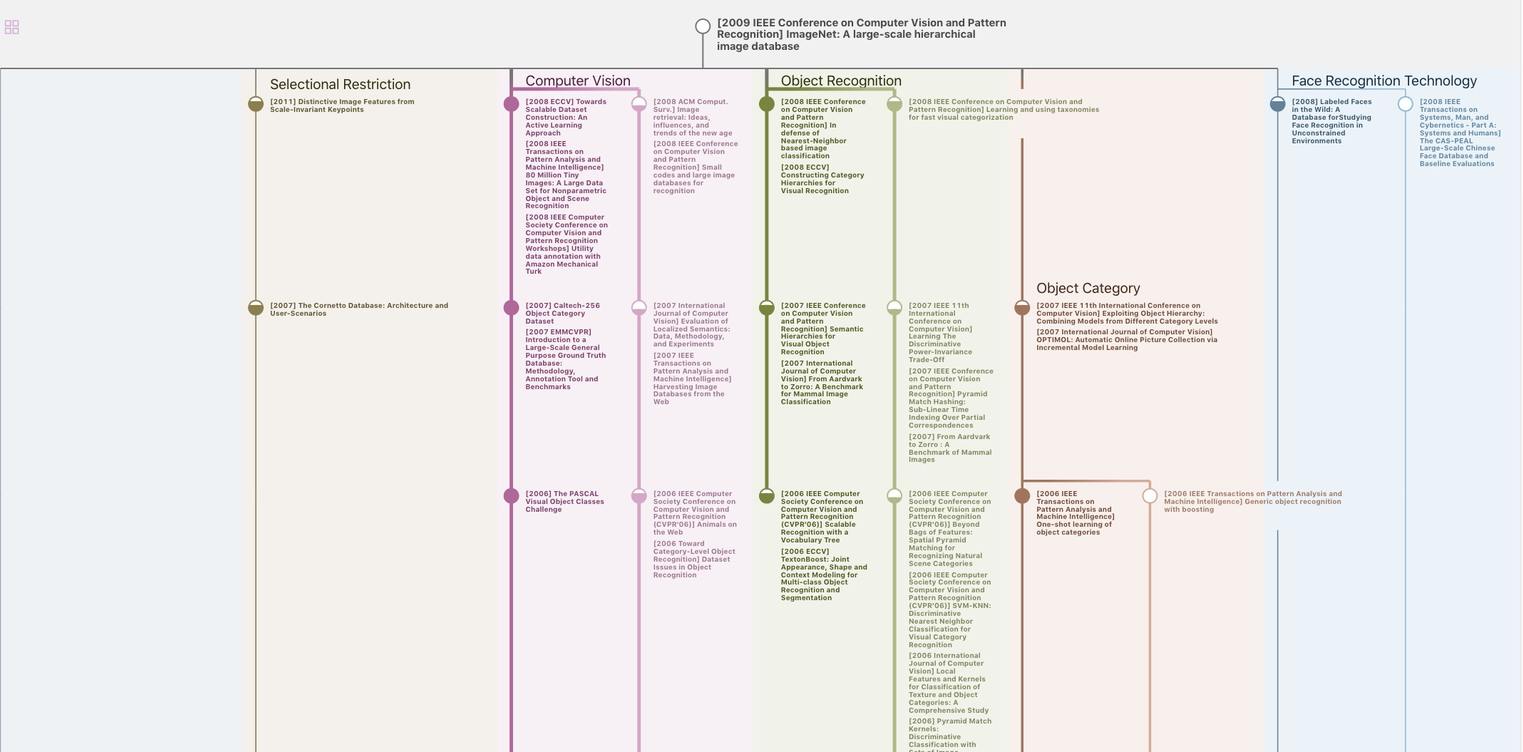
生成溯源树,研究论文发展脉络
Chat Paper
正在生成论文摘要