InconSeg: Residual-Guided Fusion With Inconsistent Multi-Modal Data for Negative and Positive Road Obstacles Segmentation
IEEE Robotics and Automation Letters(2023)
摘要
Segmentation of road obstacles, including negative and positive obstacles, is critical to the safe navigation of autonomous vehicles. Recent methods have witnessed an increasing interest in using multi-modal data fusion (e.g., RGB and depth/disparity images). Although improved segmentation accuracy has been achieved by these methods, we still find that their performance could be easily degraded if the two modalities have inconsistent information, for example, distant obstacles that can be viewed in RGB images but cannot be viewed in depth/disparity images. To address this issue, we propose a novel two-encoder-two-decoder RGB-depth/disparity multi-modal network with Residual-Guided Fusion modules. Different from most existing networks that fuse feature maps in encoders, we fuse feature maps in decoder. We also release a large-scale RGB-depth/disparity dataset recorded in both urban and rural environments with manually-labeled ground truth for both negative- and positive-obstacles segmentation. Extensive experimental results demonstrate that our network achieves state-of-the-art performance compared with other networks.
更多查看译文
关键词
obstacles,segmentation,fusion,road,residual-guided,multi-modal
AI 理解论文
溯源树
样例
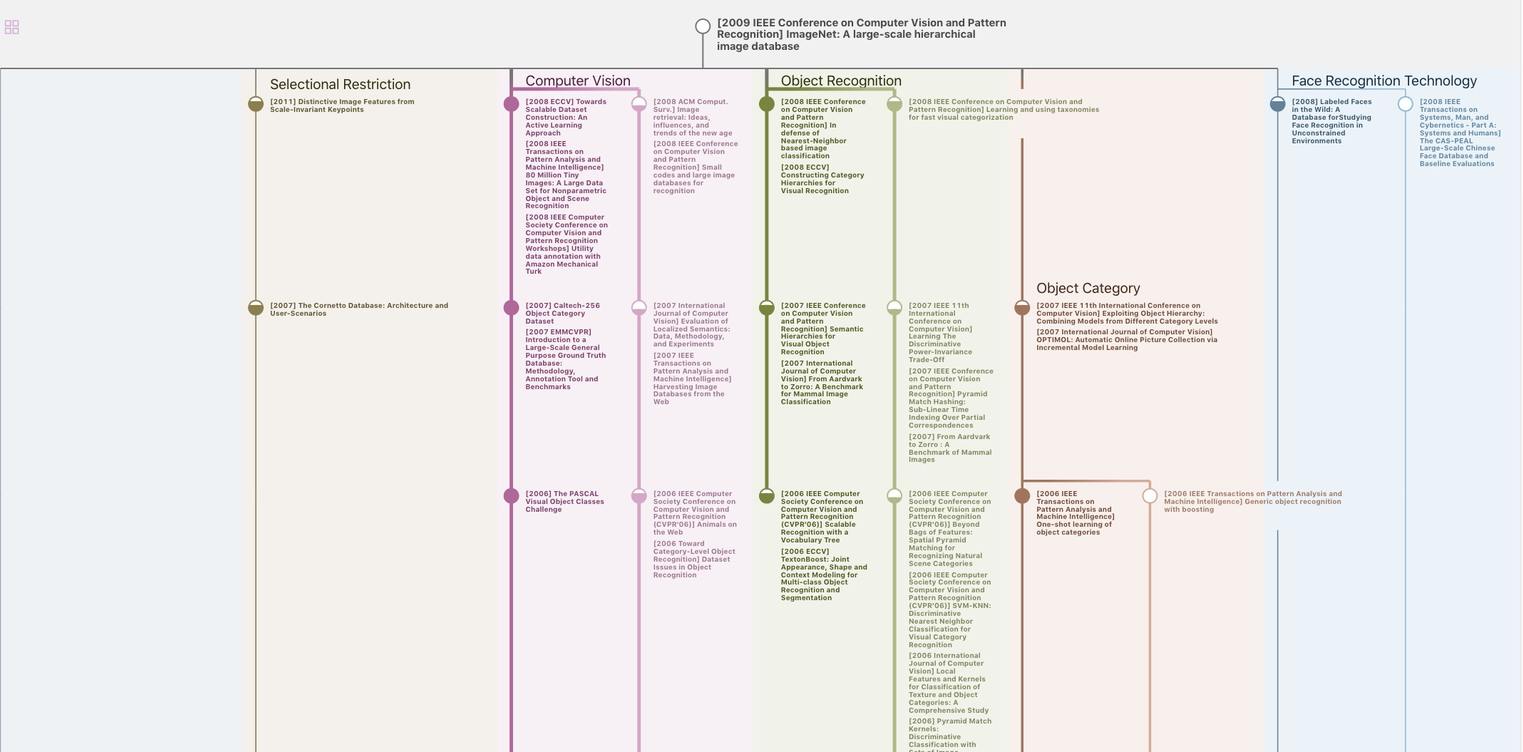
生成溯源树,研究论文发展脉络
Chat Paper
正在生成论文摘要