Geo-Localization With Transformer-Based 2D-3D Match Network
IEEE Robotics and Automation Letters(2023)
摘要
This letter presents a novel method for geographical localization by registering satellite maps with LiDAR point clouds. This method includes a Transformer-based 2D-3D matching network called D-GLSNet that directly matches the LiDAR point clouds and satellite images through end-to-end learning. Without the need for feature point detection, D-GLSNet provides accurate pixel-to-point association between the LiDAR point clouds and satellite images. And then, we can easily calculate the horizontal offset
$(\Delta x, \Delta y)$
and angular deviation
$\Delta \theta _{yaw}$
between them, thereby achieving accurate registration. To demonstrate our network's localization potential, we have designed a Geo-localization Node (GLN) that implements geographical localization and is plug-and-play in the SLAM system. Compared to GPS, GLN is less susceptible to external interference, such as building occlusion. In urban scenarios, our proposed D-GLSNet can output high-quality matching, enabling GLN to function stably and deliver more accurate localization results. Extensive experiments on the KITTI dataset show that our D-GLSNet method achieves a mean Relative Translation Error (RTE) of 1.43 m. Furthermore, our method outperforms state-of-the-art LiDAR-based geospatial localization methods when combined with odometry.
更多查看译文
关键词
Laser radar, Point cloud compression, Feature extraction, Three-dimensional displays, Satellites, Location awareness, Global Positioning System, Geo-localization, 2D-3D match, SLAM, Index Terms
AI 理解论文
溯源树
样例
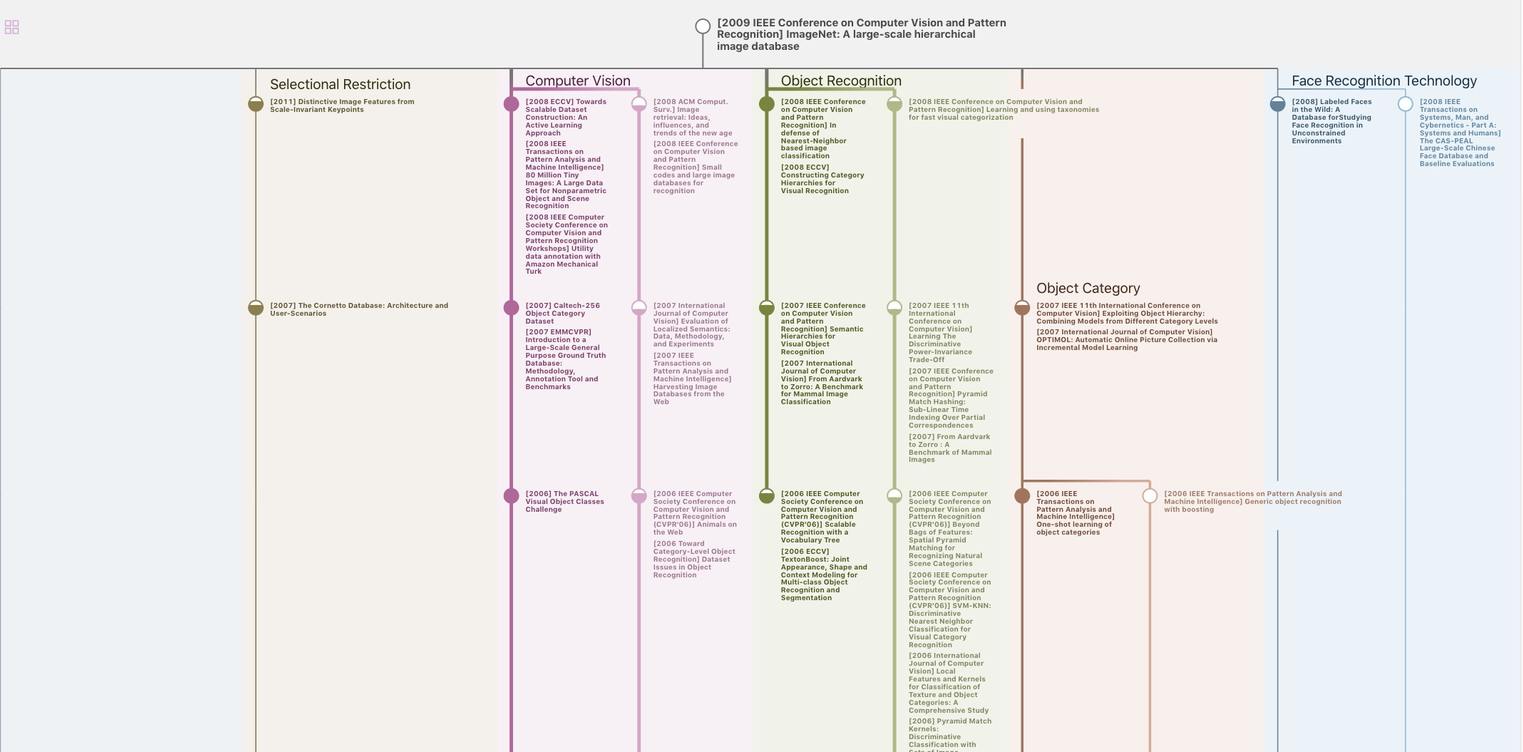
生成溯源树,研究论文发展脉络
Chat Paper
正在生成论文摘要