ADfM-Net: An Adversarial Depth-From-Motion Network Based on Cross Attention and Motion Enhanced
IEEE Robotics and Automation Letters(2023)
摘要
The temporal consistent and accurate depth estimation for consecutive images is essential for many downstream applications. However, most existing methods only infer depth from a single image, ignoring the temporal information and important depth cues from motion in the sequence. Additionally, the depths of adjacent frames are estimated separately without any constraint. In this paper, we promote the temporal consistency and accuracy of depth results from the aforementioned two aspects: multi-frame framework and consistency constraint. Firstly, a framework with cross-frame attention and motion enhancement module is proposed for better temporal consistency and depth precision. Secondly, an adversarial metric learning strategy is introduced to further constrain the consistency of adjacent depth results, without any additional computation and memory cost. The experiments on KITTI and Cityscapes datasets demonstrate the effectiveness of our framework. Furthermore, noting that the traditional metrics can not reveal the consistency of the depth results, a new temporal consistency metric is proposed, which would facilitate further research.
更多查看译文
关键词
Deep learning for visual perception,deep learning methods,visual learning
AI 理解论文
溯源树
样例
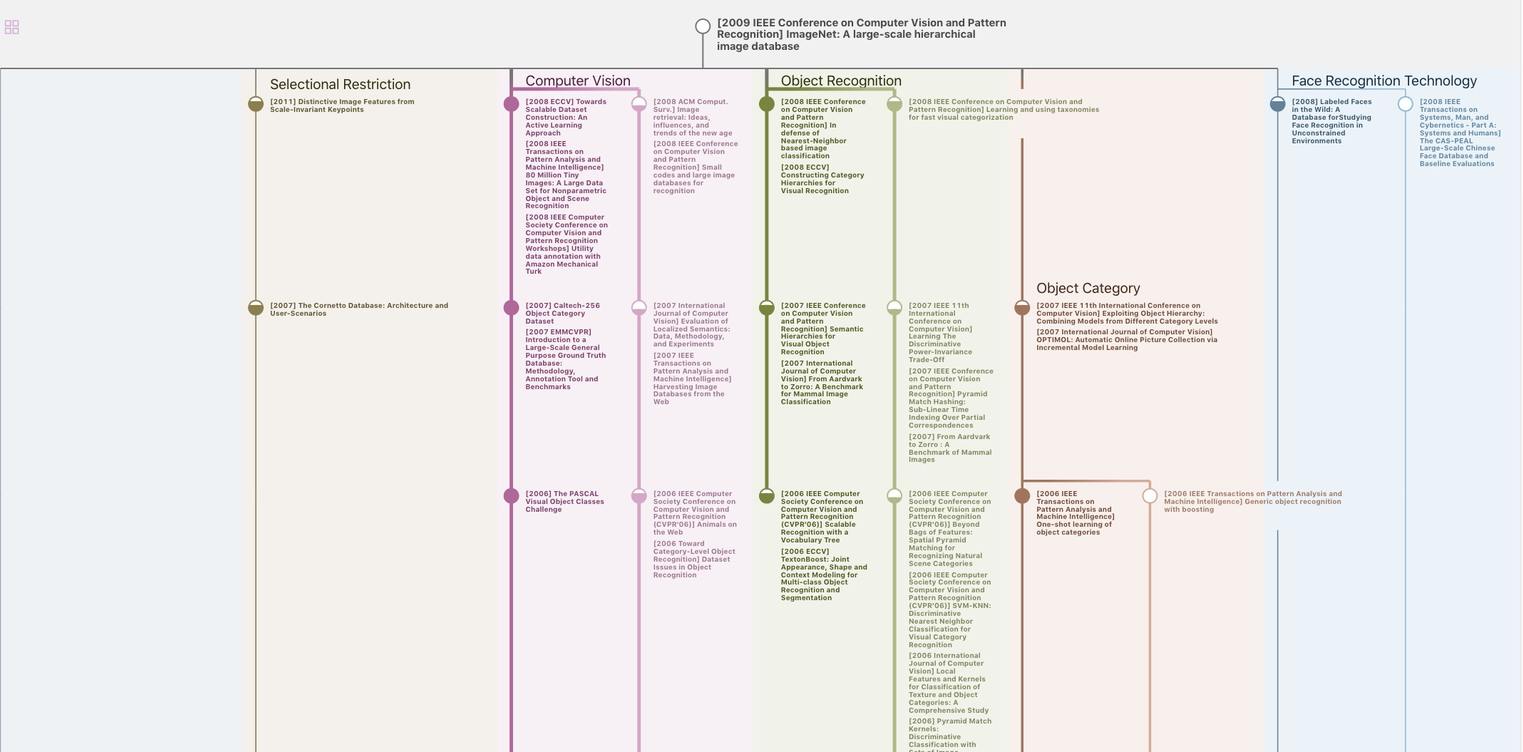
生成溯源树,研究论文发展脉络
Chat Paper
正在生成论文摘要