Mind-the-gap Part II: Improving quantitative estimates of cloud and rain water path in oceanic warm rain using spaceborne radars
crossref(2020)
摘要
Abstract. The intrinsic small spatial scales and low reflectivity structure of oceanic warm precipitating clouds suggest that millimeter spaceborne radars are best suited to provide quantitative estimates of cloud and rain liquid water path (LWP). This assertion is based on their smaller horizontal footprint, high sensitivities, and a wide dynamic range of path integrated attenuations associated to warm rain cells across the millimeter wavelength spectrum, with diverse spectral responses to rain and cloud partitioning. State-of-the-art single-frequency radar profiling algorithms of warm rain seem to be inadequate because of their dependence on uncertain assumptions on the rain/cloud partitioning and of the rain microphysics. Here, high resolution cloud resolving model simulations for the Rain in Cumulus over the Ocean field study and a spaceborne forward radar simulator are exploited to assess the potential of existing and future spaceborne radar system for quantitative warm rain microphysical retrievals. Specifically, the detrimental effects of non-uniform beam filling on path integrated attenuation (PIA) estimates, the added value of brightness temperatures (TBs) derived adopting radiometric radar modes, and the performances of multi-frequency PIA and/or TB combinations when retrieving liquid water path partitioning into cloud (c-LWP) and rain (r-LWP) are assessed. Results show that (1) Ka and W-band TBs add useful constraints and are effective at lower LWPs than the same frequency PIAs; (2) matched-beam combined TBs and PIAs from single/multi-frequency radars can significantly narrow down uncertainties in retrieved cloud and rain liquid water paths; (3) the configuration including PIAs, TBs and near surface reflectivities for the Ka-W band pairs in our synthetic retrieval can achieve rmse better than 30 % for c-LWPs and r-LWPs exceeding 100 g/m2.
更多查看译文
AI 理解论文
溯源树
样例
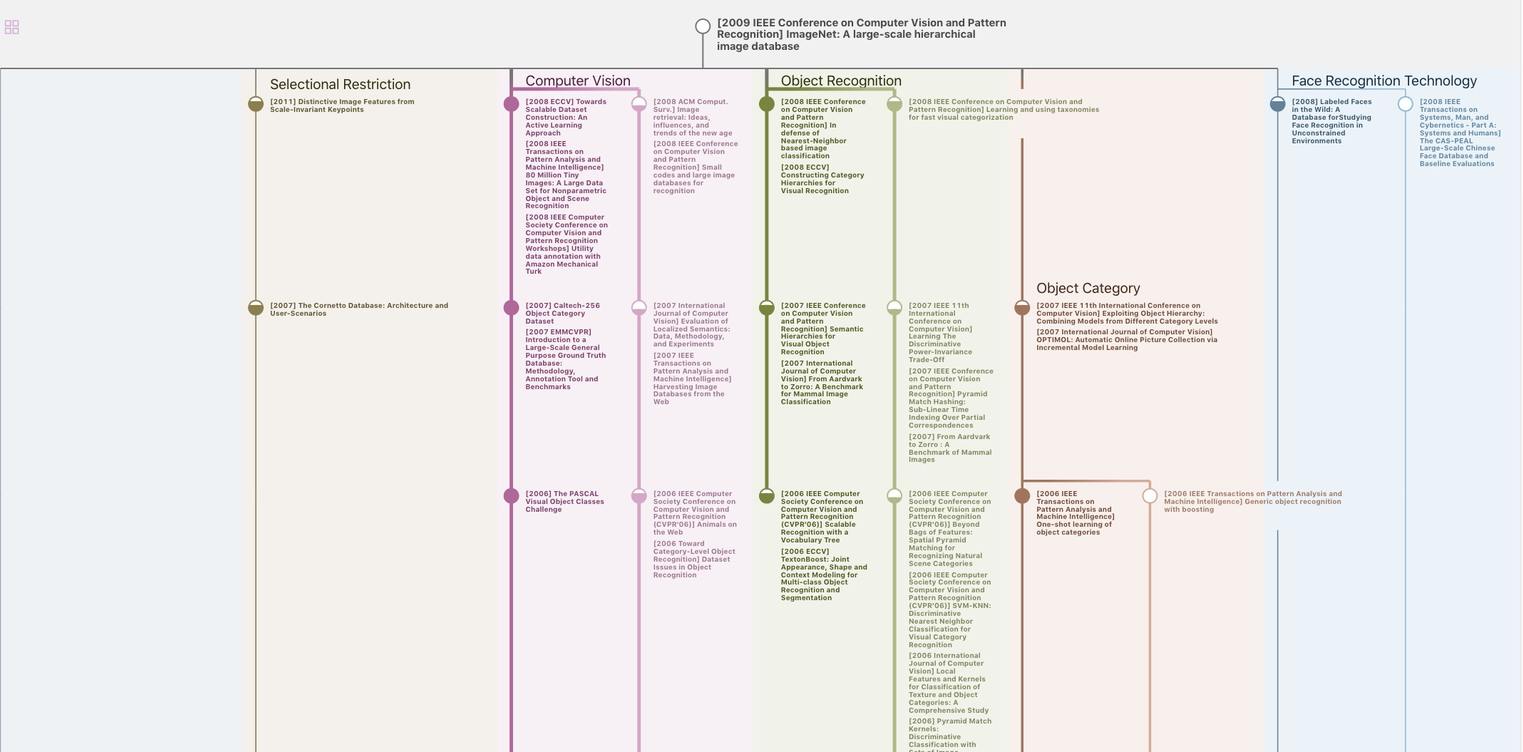
生成溯源树,研究论文发展脉络
Chat Paper
正在生成论文摘要