LVSegNet: A novel deep learning-based framework for left ventricle automatic segmentation using magnetic resonance imaging
Computer Communications(2023)
摘要
Automatic segmentation of the left ventricle(LV) using magnetic resonance image (MRI) is essential for assessing cardiac functionality such as area, volume, and ejection fraction in clinical applications. With the development of deep learning(DL), LV segmentation has achieved a series of huge breakthroughs and promising performance in recent years. However, it also encounters some dilemmas in which ill-defined borders and operator dependence problems. U-net is a well-known architecture in medical image segmentation based on an encoder–decoder method, which model achieves outstanding performance. However, the most current methods that follow the existing U-net-based paradigm ignore the contribution of context semantic strengths during the segmentation, which cannot explicitly and thoroughly exploit the intrinsic similarity of medical images. To tackle these problems, we propose LVSegNet, a comprehensive model, which explicitly fulfills the segmentation of LV using MRI via elaborately combining various semantic learning modules. This model includes three parts: encoder, center, and decoder. Specifically, the ResLink block of the encoder part designs pipeline attention, which integrates the context feature across channels. The dense atrous spatial pyramid pooling (DenseASPP) module of the center part is leveraged to magnify the receptive field. The dense up-sampling convolution (DUC) module of the decoder is utilized to replace common up-sampling, which recovers the feature information of images to the greatest extent. Moreover, we also conduct extensive experiments on public LV segmentation benchmarks of Sunnybrook Cardiac Data and our clinical dataset. We demonstrate and validate the performance superiority of LVSegNet, outperforming the previous outstanding counterpart methods by 15.4%, 6.6%, and 5.3% in F1-score compared to U-net, deeplabV3, and deeplab V3+ respectively. Experimental results have verified that the proposed LV automatic segmentation model is more robust than various encoder–decoder counterparts.
更多查看译文
关键词
0000,1111,Deep learning,Image segmentation,Left ventricle,Magnetic resonance image,Convolution neural network
AI 理解论文
溯源树
样例
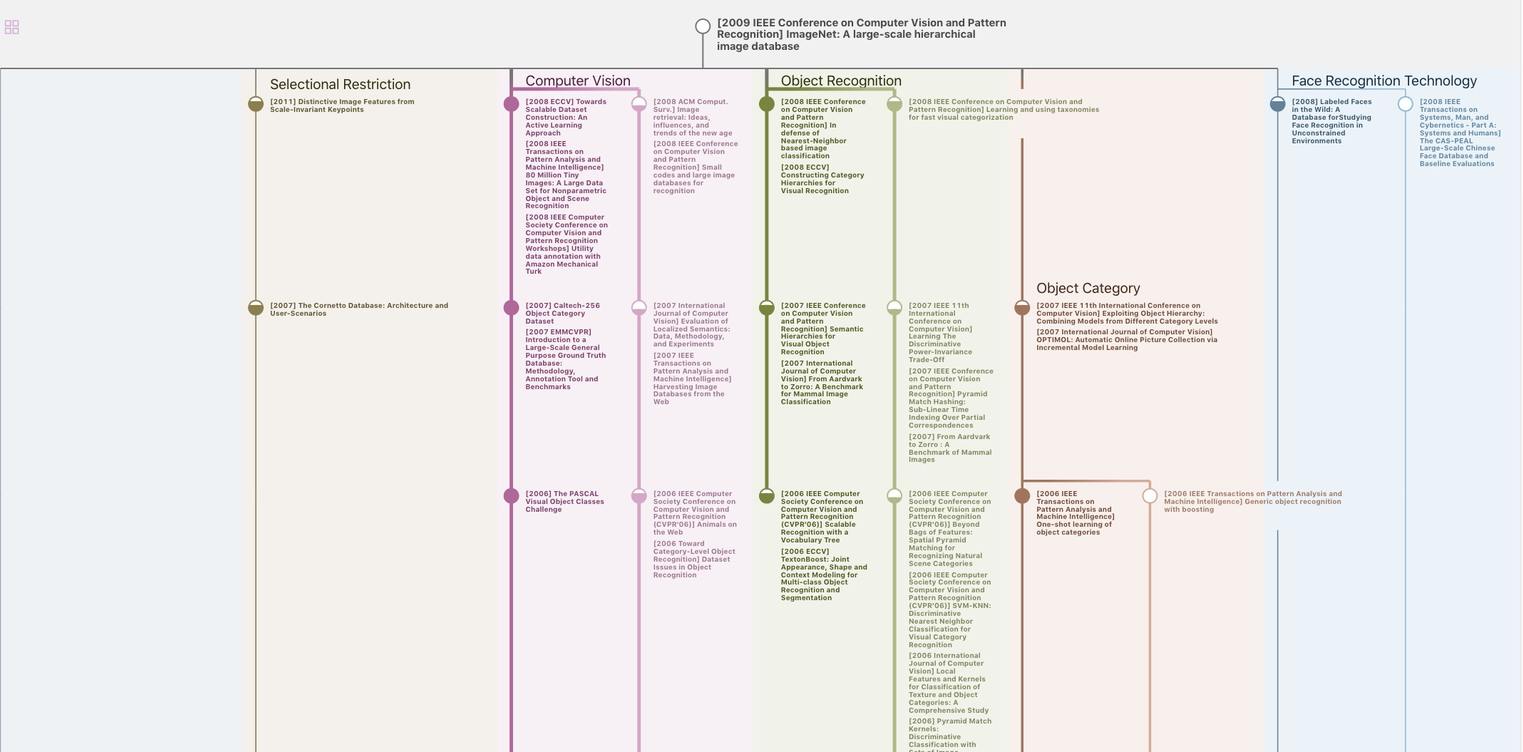
生成溯源树,研究论文发展脉络
Chat Paper
正在生成论文摘要