Physics informed and data-based augmented learning in structural health diagnosis
Computer Methods in Applied Mechanics and Engineering(2023)
摘要
Data-based diagnosis has been extensively addressed in the domain of structural health monitoring. Data exhibit patterns able to infer the existence of damaged areas, and in some cases to locate them. However, optimal predictive maintenance requires the ability to make fine predictions, which can be obtained by interrogating a model describing the systems under scrutiny. Thus, data is not only expected to enable diagnosis, but also to update the nominal model to incorporate the effects of localized damage, from data assimilation. Such a data-driven model enrichment can be performed by using different routes. In the present paper we propose an augmented physics-informed neural network procedure that allows updating the model, while completing the data collected by few sensors, using physics-informed NN and an appropriate regularized loss function. & COPY; 2023 The Author(s). Published by Elsevier B.V. This is an open access article under the CC BY license (http://creativecommons.org/licenses/by/4.0/).
更多查看译文
关键词
Physics -informed neural networks,Elastic equation,Model correction,Machine learning,Data completion
AI 理解论文
溯源树
样例
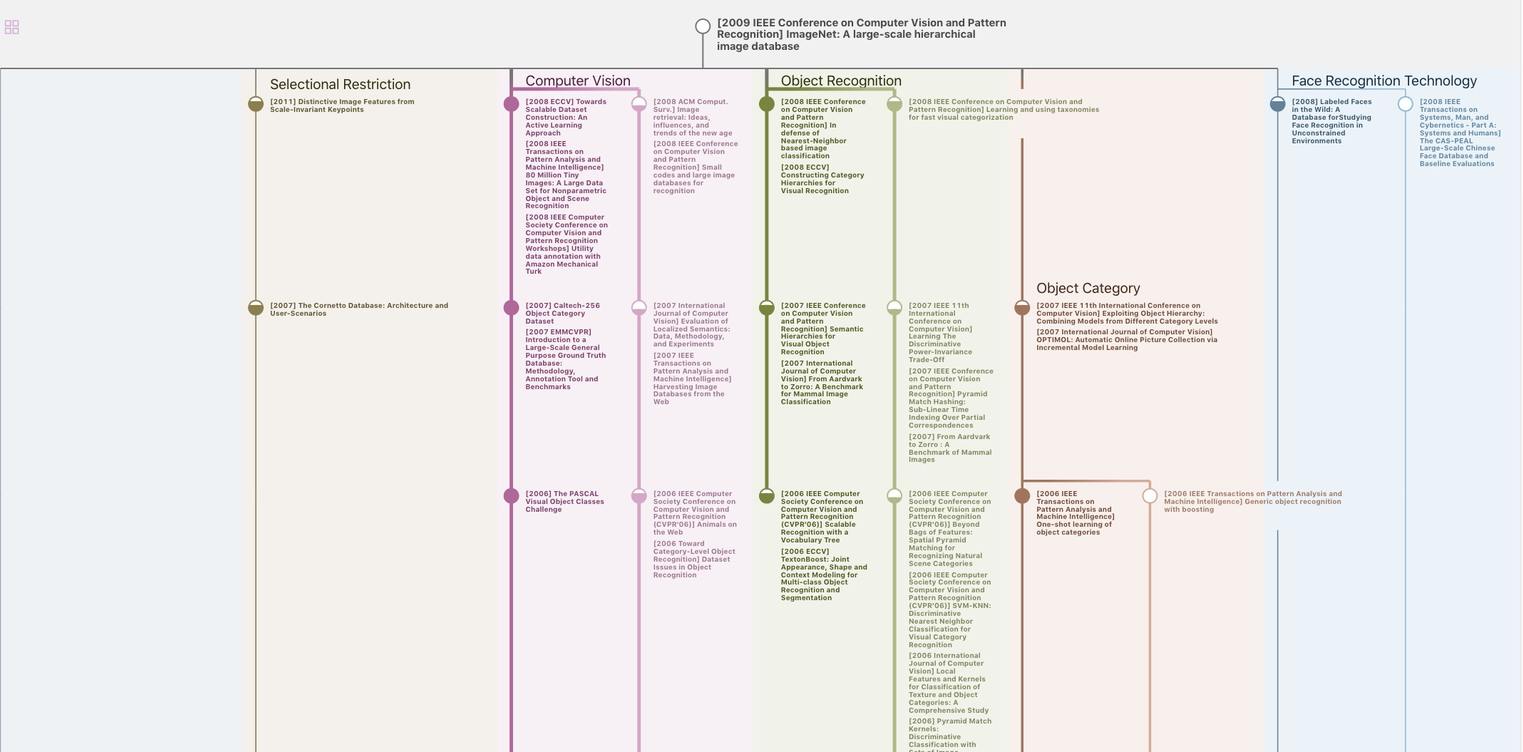
生成溯源树,研究论文发展脉络
Chat Paper
正在生成论文摘要