An Equivalent Graph Reconstruction Model and its Application in Recommendation Prediction
CoRR(2023)
摘要
Recommendation algorithm plays an important role in recommendation system (RS), which predicts users' interests and preferences for some given items based on their known information. Recently, a recommendation algorithm based on the graph Laplacian regularization was proposed, which treats the prediction problem of the recommendation system as a reconstruction issue of small samples of the graph signal under the same graph model. Such a technique takes into account both known and unknown labeled samples information, thereby obtaining good prediction accuracy. However, when the data size is large, solving the reconstruction model is computationally expensive even with an approximate strategy. In this paper, we propose an equivalent reconstruction model that can be solved exactly with extremely low computational cost. Finally, a final prediction algorithm is proposed. We find in the experiments that the proposed method significantly reduces the computational cost while maintaining a good prediction accuracy.
更多查看译文
关键词
equivalent graph reconstruction model,recommendation,prediction
AI 理解论文
溯源树
样例
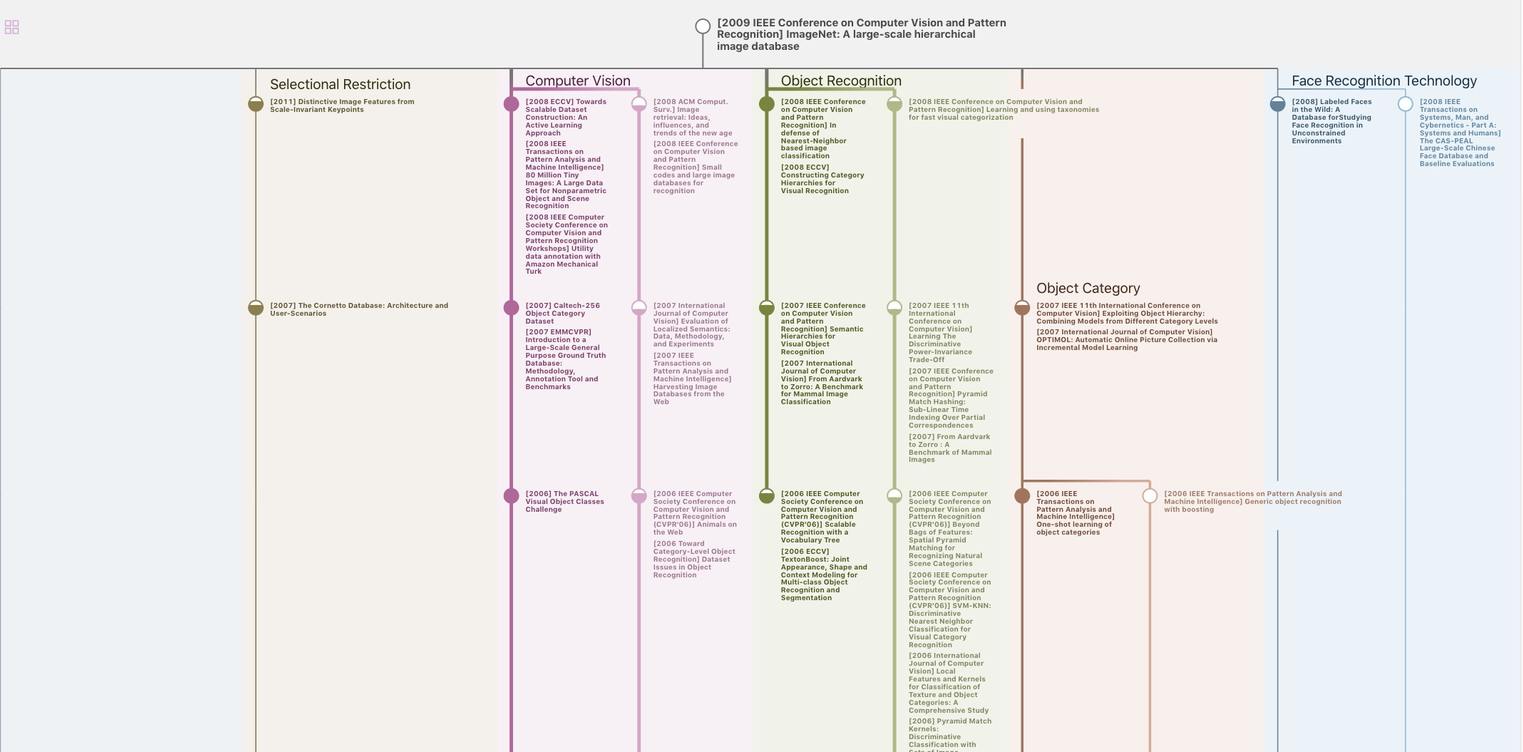
生成溯源树,研究论文发展脉络
Chat Paper
正在生成论文摘要