An attempt at improving atmospheric corrections\\in InSAR using cycle-consistent adversarial networks
EarthArXiv (California Digital Library)(2020)
摘要
Interferometry from satellite radar has thrived as a major asset to study surface deformations from earthquakes, volcanoes, aquifers, glaciers, landslides, etc. Most signals recorded in an interferogram have precise enough models to remove them almost completely. Yet, current models still fail to capture the full range and scales of variations of atmospheric perturbations. This work explores the use of an image-to-image translation method, CycleGAN, to learn a function that wraps and improves an imperfect model for atmospheric correction. CycleGAN is a generative adversarial network, in which discriminators determine which images are real, and generators produce images to fool the discriminators. Training the discriminators and generators against each other improves the resulting translation function. We have tested this approach using Sentinel-1A data gathered around Puebla, Mexico, which was the starting point of the 2017 earthquake that devastated Mexico City. CycleGAN can generate visually compelling fringes, including small-scale perturbations that are absent from the atmospheric models, while limiting noisy areas. However, it fails to capture the variations of amplitude behind the fringes, especially at large scale, and the generated interferograms remain too different from the real interferograms. Solving that amplitude issue could create practical applications for a CycleGAN-type method in atmospheric correction or phase unwrapping. The large amount of InSAR data and the continuous progress of deep learning methods provide ample opportunity for improvement.
更多查看译文
关键词
networks,cycle-consistent
AI 理解论文
溯源树
样例
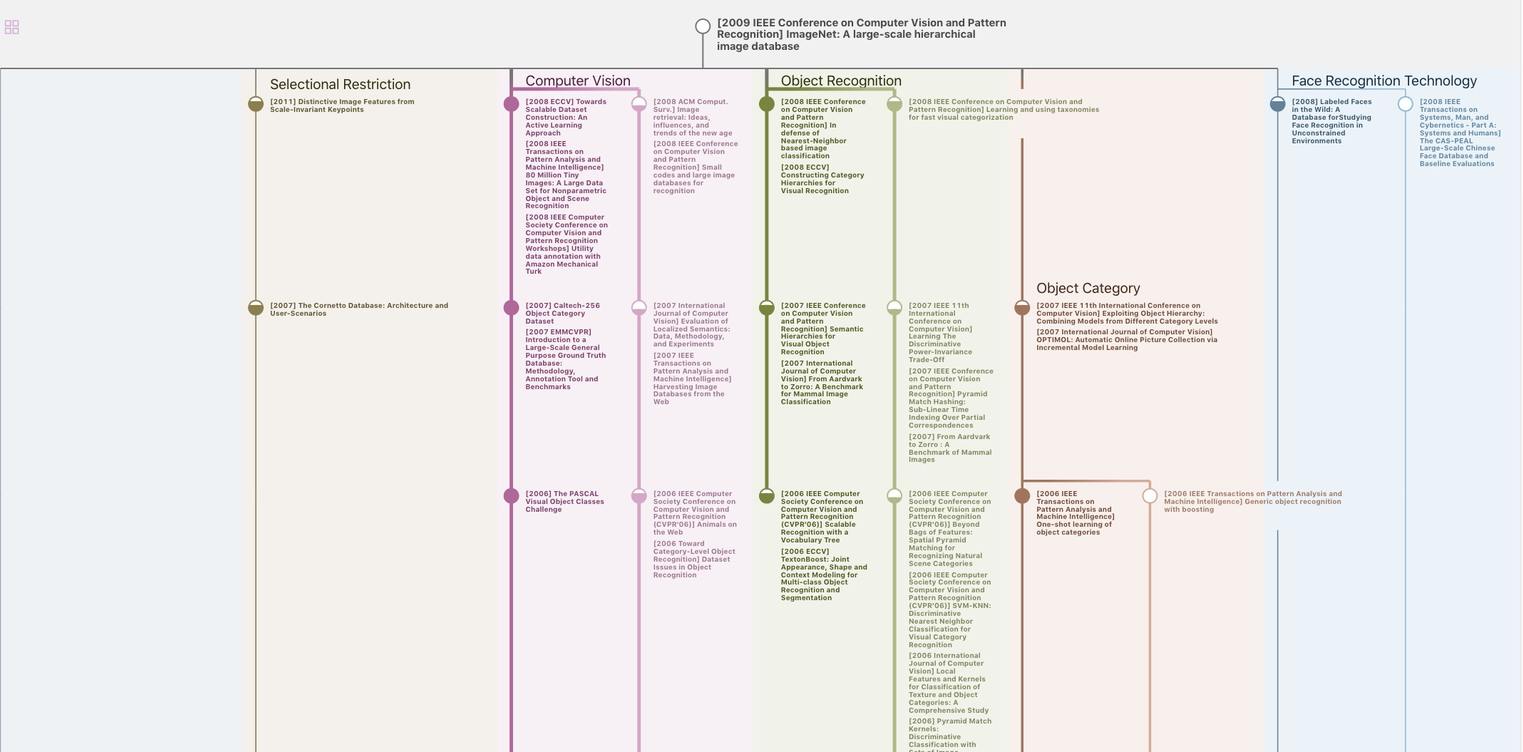
生成溯源树,研究论文发展脉络
Chat Paper
正在生成论文摘要