T4SEpp: a pipeline integrated with protein language models effectively predicting bacterial type IV secreted effectors
bioRxiv (Cold Spring Harbor Laboratory)(2023)
摘要
Many pathogenic bacteria use type IV secretion systems to deliver effectors (T4SEs) into the cytoplasm of eukaryotic cells and causes diseases. Identification of effectors is a crucial step in understanding the mechanisms of bacterial pathogenicity, but it remains a big challenge. In this study, we used the full-length embedding features generated by six pre-trained protein language models to train classifiers predicting T4SEs, and compared their performance. An integrated pipeline T4SEpp was assembled by a module searching full-length, signal sequence and effector domain homologs of known T4SEs, a machine learning module based on the hand-crafted features extracted from the signal sequences, and the third module containg three best-performed protein language pre-trained models. T4SEpp outperforms the other state-of-the-art software tools, achieving ~0.95 sensitivity at a high specificity of ~0.99 based on the assessment of an independent testing dataset. T4SEpp predicted 13 potential T4SEs, including the H. pylori cytotoxin-associated gene A (CagA). Among these, 10 T4SEs have the potential to interact with at least one human protein. This suggests that these potential T4SEs may be associated with the pathogenicity of H. pylori. Overall, T4SEpp provides a better solution to assist identification of bacterial T4SEs, and facilitates studies on bacterial pathogenicity. T4SEpp is freely accessible at https://bis.zju.edu.cn/T4SEpp.
### Competing Interest Statement
The authors have declared no competing interest.
更多查看译文
关键词
protein language models,bacterial type effectively
AI 理解论文
溯源树
样例
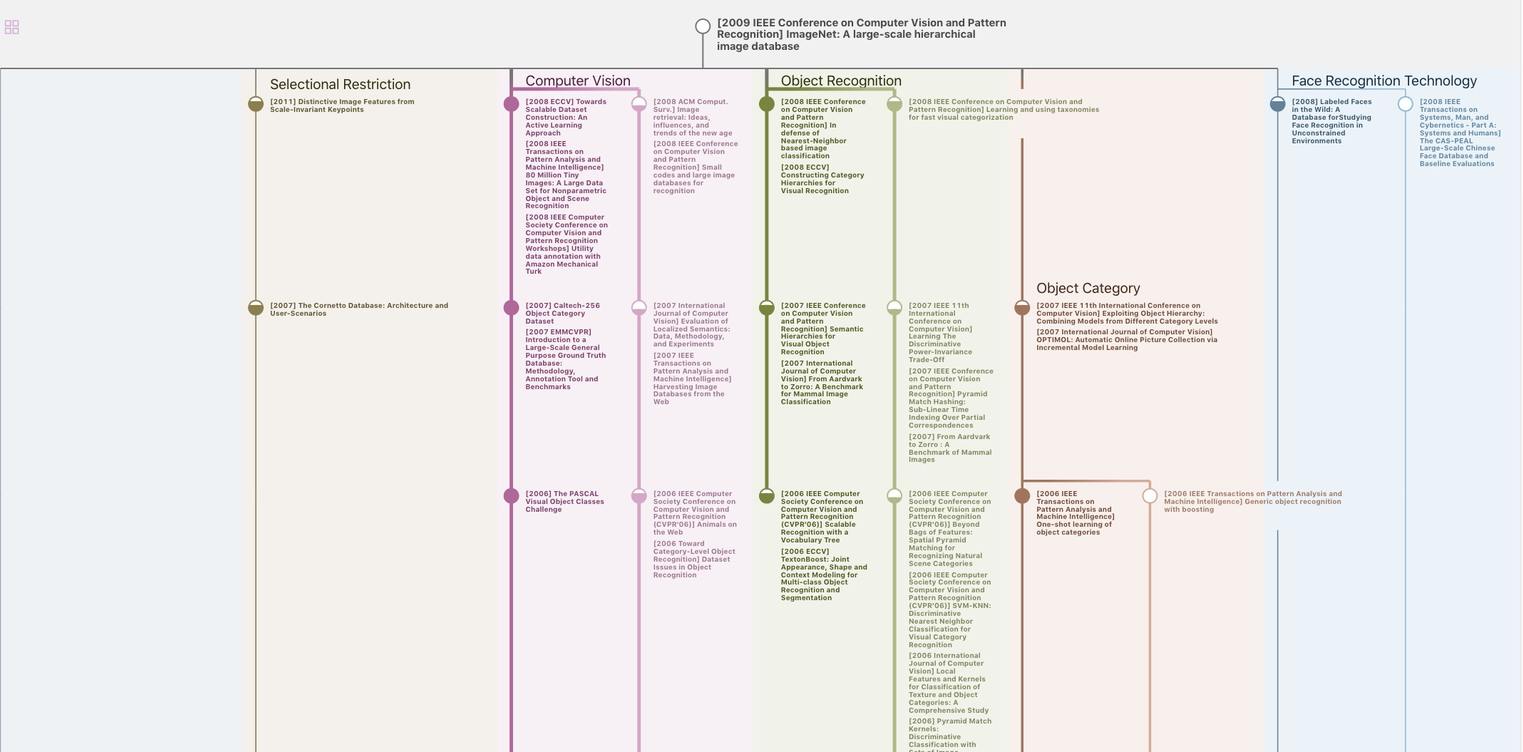
生成溯源树,研究论文发展脉络
Chat Paper
正在生成论文摘要