InGene: Finding influential genes from embeddings of nonlinear dimension reduction techniques
biorxiv(2024)
摘要
We introduce InGene , the first of its kind, fast and scalable non-linear, unsupervised method for analyzing single-cell RNA sequencing data (scRNA-seq). While non-linear dimensionality reduction techniques such as t-SNE and UMAP are effective at visualizing cellular sub-populations in low-dimensional space, they do not identify the specific genes that influence the transformation. InGene addresses this issue by assigning an importance score to each expressed gene based on its contribution to the construction of the low-dimensional map. InGene can provide insight into the cellular heterogeneity of scRNA-seq data and accurately identify genes associated with cell-type populations or diseases, as demonstrated in our analysis of scRNA-seq datasets.
### Competing Interest Statement
The authors have declared no competing interest.
更多查看译文
AI 理解论文
溯源树
样例
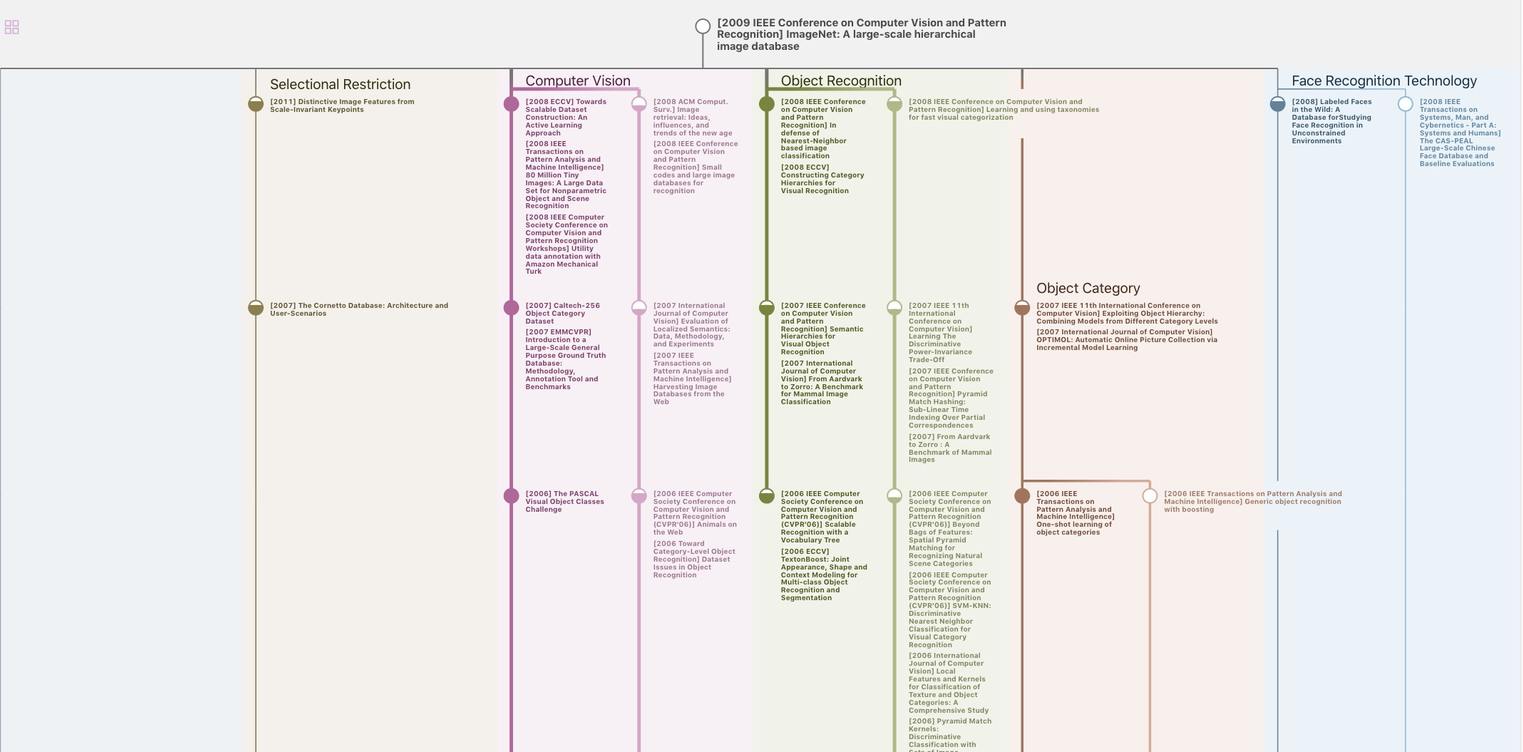
生成溯源树,研究论文发展脉络
Chat Paper
正在生成论文摘要