Model-X knockoffs reveal data-dependent limits on regulatory network identification
biorxiv(2023)
摘要
Computational biologists have long sought to automatically infer transcriptional regulatory networks (TRNs) from gene expression data, but such approaches notoriously suffer from false positives. Two points of failure could yield false positives: faulty hypothesis testing, or erroneous assumption of a classic criterion called causal sufficiency . We show that a recent statistical development, model-X knockoffs, can effectively control false positives in tests of conditional independence in mouse and E. coli data, which rules out faulty hypothesis tests. Yet, benchmarking against ChIP and other gold standards reveals highly inflated false discovery rates. This identifies the causal sufficiency assumption as a key limiting factor in TRN inference.
### Competing Interest Statement
A.B. is a stockholder for Alphabet, Inc, and has consulted for Third Rock Ventures.
更多查看译文
关键词
data-dependent
AI 理解论文
溯源树
样例
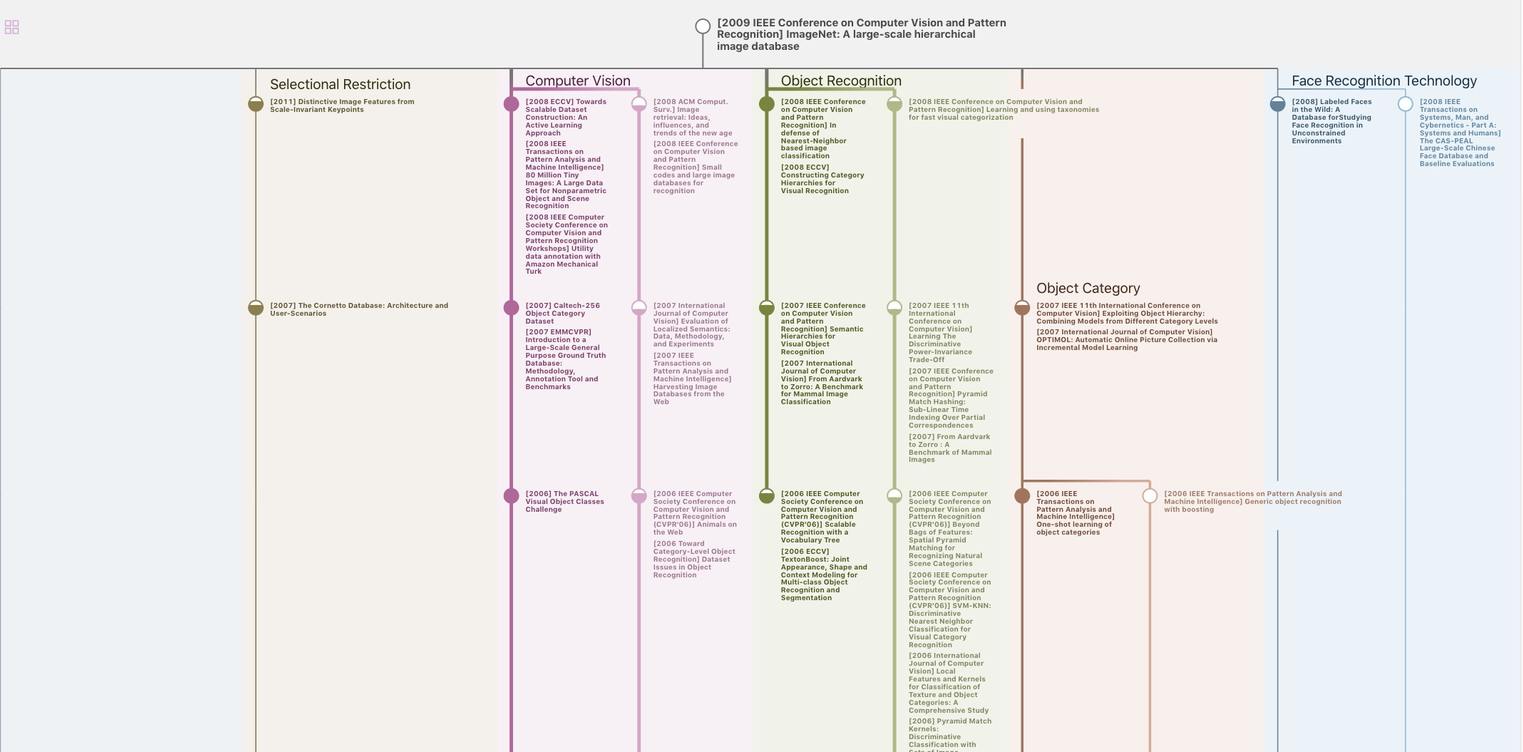
生成溯源树,研究论文发展脉络
Chat Paper
正在生成论文摘要