PandoGen: Generating complete instances of future SARS-CoV2 sequences using Deep Learning
biorxiv(2023)
摘要
Deep generative models have achieved state-of-the-art performance in many areas including image generation, code generation and natural language generation. We explore the use of deep generative models in producing complete instances of as-yet undiscovered SARS-CoV2 Spike protein sequences. The Spike protein is the primary initiator of infection by the SARS-CoV2 virus, and hence, the ability to predict future manifestations of the Spike protein is invaluable, enabling critical tasks such as advance validation of pharmaceutical interventions. We examine specific requirements of generating sequences for a pandemic and formulate a novel framework for training models for these requirements. Our solution only uses sequence information submitted in SARS-CoV2 repositories without the need for additional laboratory experiments. Resulting models substantially outperform a state-of-the-art generative model for protein sequences finetuned on SARS-CoV2 data. Samples produced from our models are four times as likely to be novel and real SARS-CoV2, and ten times as infectious, cumulatively. We find that among higher ranked sequences generated from our model, over 70% are discovered in the future, over twice the rate of the baseline. Our models represent a promising source of hypothetical SARS-CoV2 sequences, thus providing a key tool for advance preparation against the pandemic. PandoGen is available at
### Competing Interest Statement
The authors have declared no competing interest.
更多查看译文
AI 理解论文
溯源树
样例
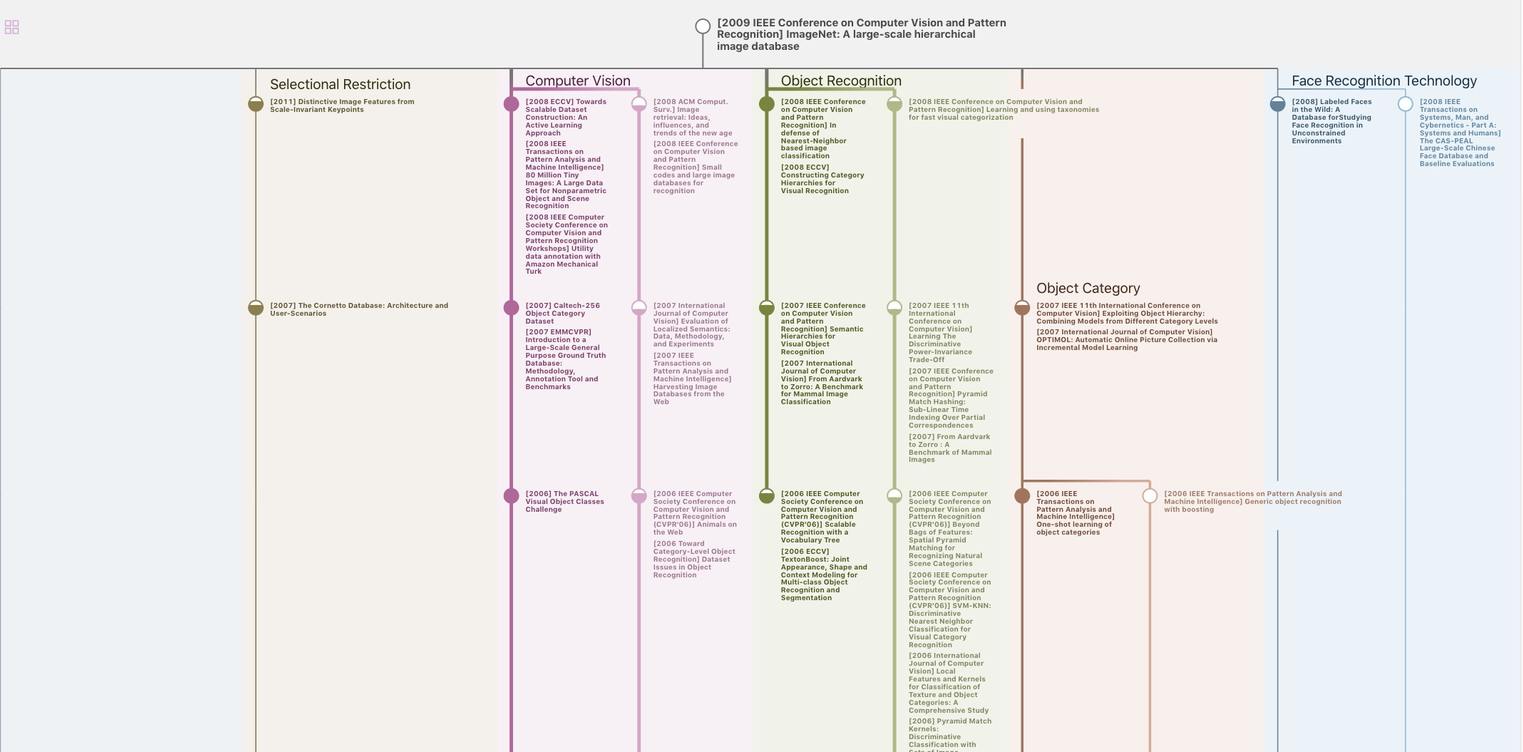
生成溯源树,研究论文发展脉络
Chat Paper
正在生成论文摘要