VTwins: Identifying Robust Associations from High-dimensional Data of Limited Samples
Research Square (Research Square)(2021)
摘要
Abstract Robust associations are strong indicators for causalities but challenging for identification from high-dimensional datasets. In examples of metagenomic research where microbiota is highly complex and variable, low concordance between studies in identifying disease-causative microbes has become the main obstacle in the field. Here, we develop a simple method—Virtual Twins (VTwins)—for inferring robust associations, imitating the twins in genetic research. From the original groups, paired samples of distinct phenotypes but matched taxonomical profiles are selected to reconstruct a “Twin” cohort, where statistical significance is often achieved. In direct comparison to current methods by revisiting the largest meta-analysis metagenomic dataset, VTwins can 10-fold reduce the sample-size for recalling disease-associated microbes robustly across-datasets and constructing machine-learning models of the same accuracy level as pooled samples in predicting disease status. In practice, VTwins is straightforward, powerful, and versatile in handling highly variable and high-dimensional datasets, suggesting potentials in mining causalities in the Big-data Era.
更多查看译文
关键词
robust associations,vtwins,data,high-dimensional
AI 理解论文
溯源树
样例
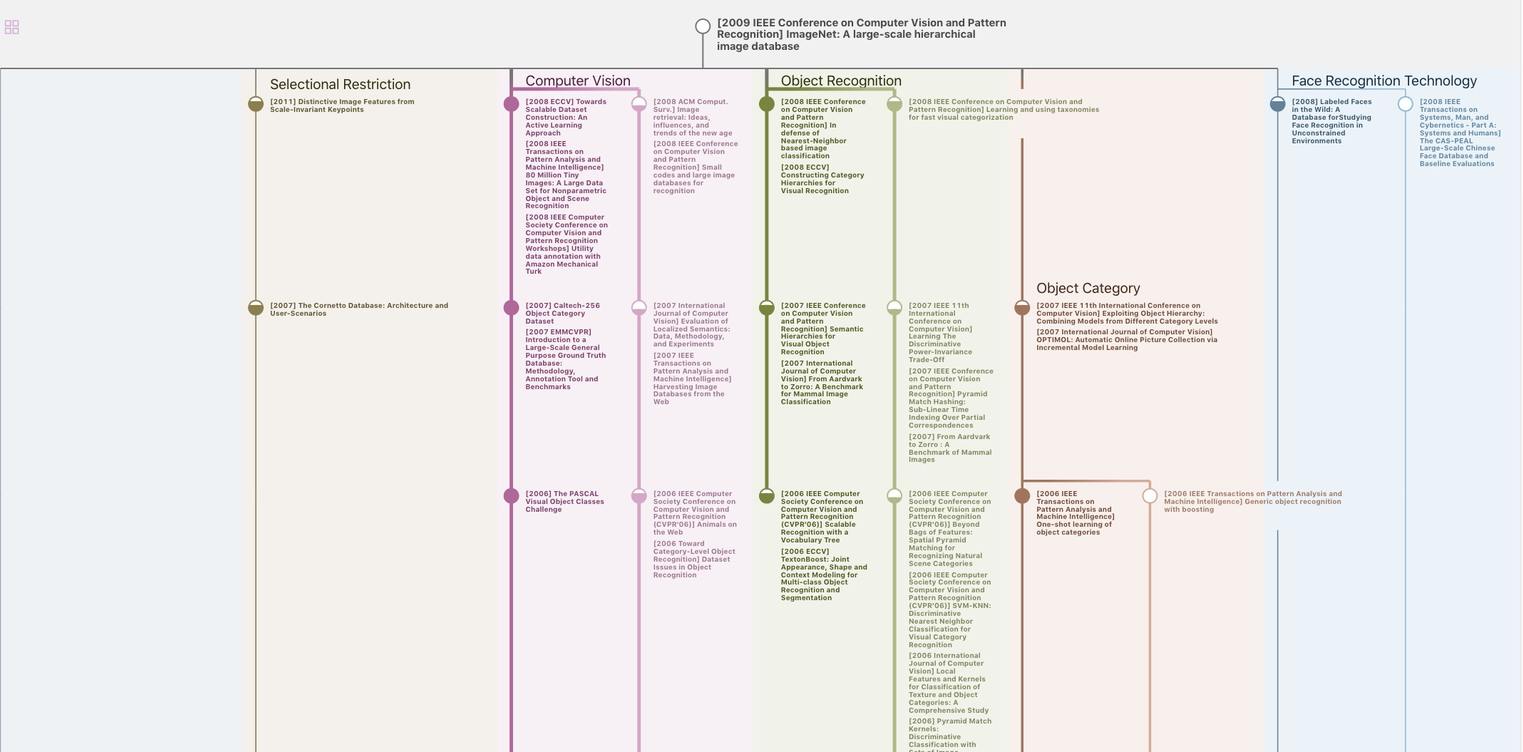
生成溯源树,研究论文发展脉络
Chat Paper
正在生成论文摘要