Statistical learning reflects inferences about unique predictive relations
crossref(2021)
摘要
The mind adeptly registers statistical regularities in experience, often incidentally and implicitly. We used a visual statistical learning paradigm to study what kinds of statistics it spontaneously computes in such conditions. We found that participants’ learning of pairwise predictive relations was best explained by an inferentially sophisticated quantity, deltaP, which reflects whether a high conditional probability between an event pair is unique. We showed that uniqueness can be reduced by either a strong competing predictor or an overall high base rate of the outcome. Both can result from normalization: if predictors of the same effect trade off, a predictor must raise the probability of the effect more than the others to be effective. Adding normalization to the Rescorla-Wagner learning model captures these results. We argue that the uniqueness of a relation is an intrinsically important statistical property that governs learning without incentive or deliberation.
更多查看译文
AI 理解论文
溯源树
样例
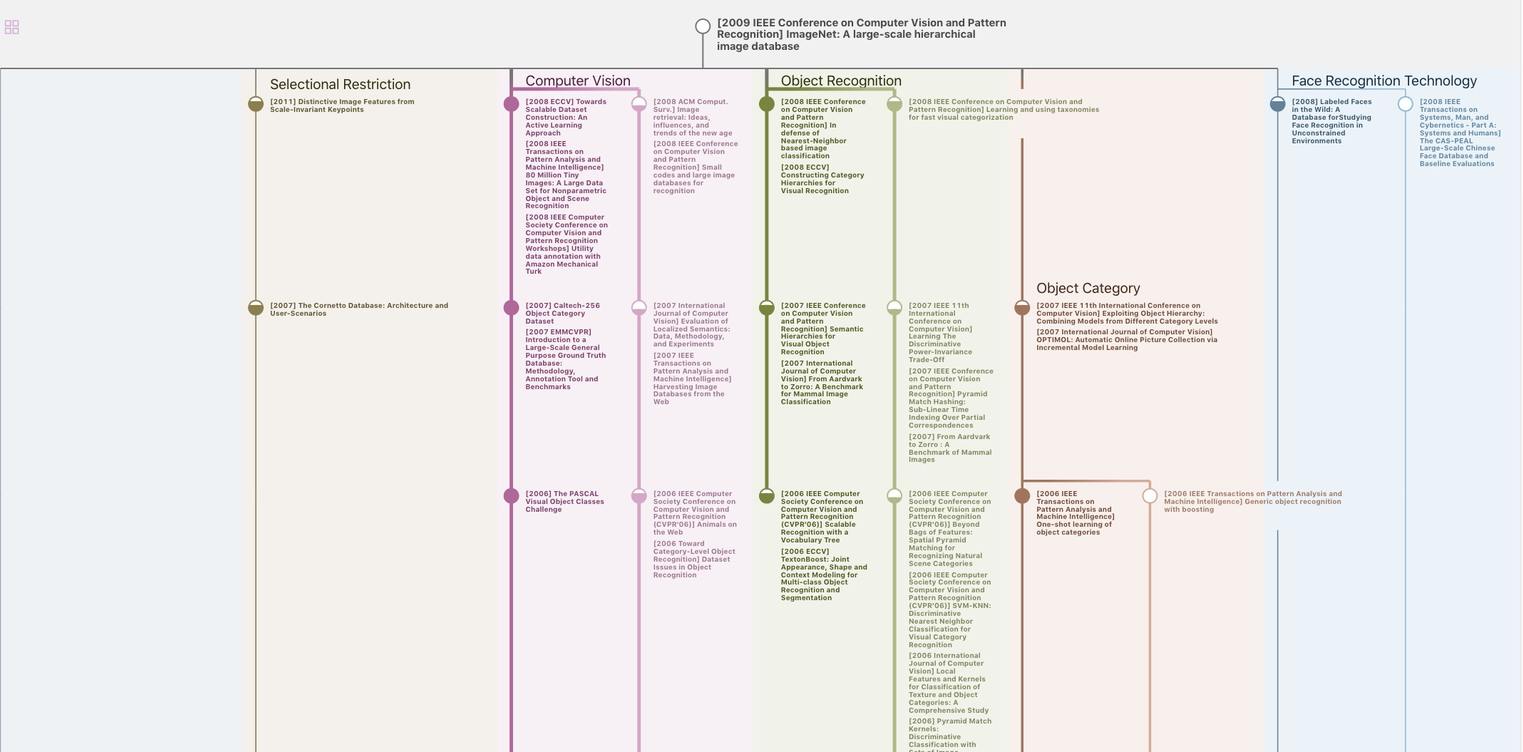
生成溯源树,研究论文发展脉络
Chat Paper
正在生成论文摘要