Inflated false-positive risk in common regression analyses: A combinatorial analysis of model sets
crossref(2021)
摘要
Even with a small number of variables researchers can test many possible models of their data thus increasing the risk of false-positive results. Using combinatorics, we show that one key independent variable and three covariates can generate 95 possible models, while six covariates can generate over 2.3 million models. Such large model sets nearly guarantee false-positive results. Using simulation, we show that preregistering a single analysis with a key independent variable heavily reduces the risk of false-positives. However, even so, many models produce false-positive results with a much higher probability than the expected 5 %. The worst-case scenario are models with interactions between binary dummy coded variables and omitted main effects. Such models can generate false-positive results up over 40 % of the time. While preregistration is a crucial step towards reducing false-positive results, researchers need to carefully consider what analyses they plan and we provide recommendations for what analyses to avoid. Our findings also suggest that interpreting p-values in exploratory analyses might be meaningless considering the high false-positive probability.
更多查看译文
AI 理解论文
溯源树
样例
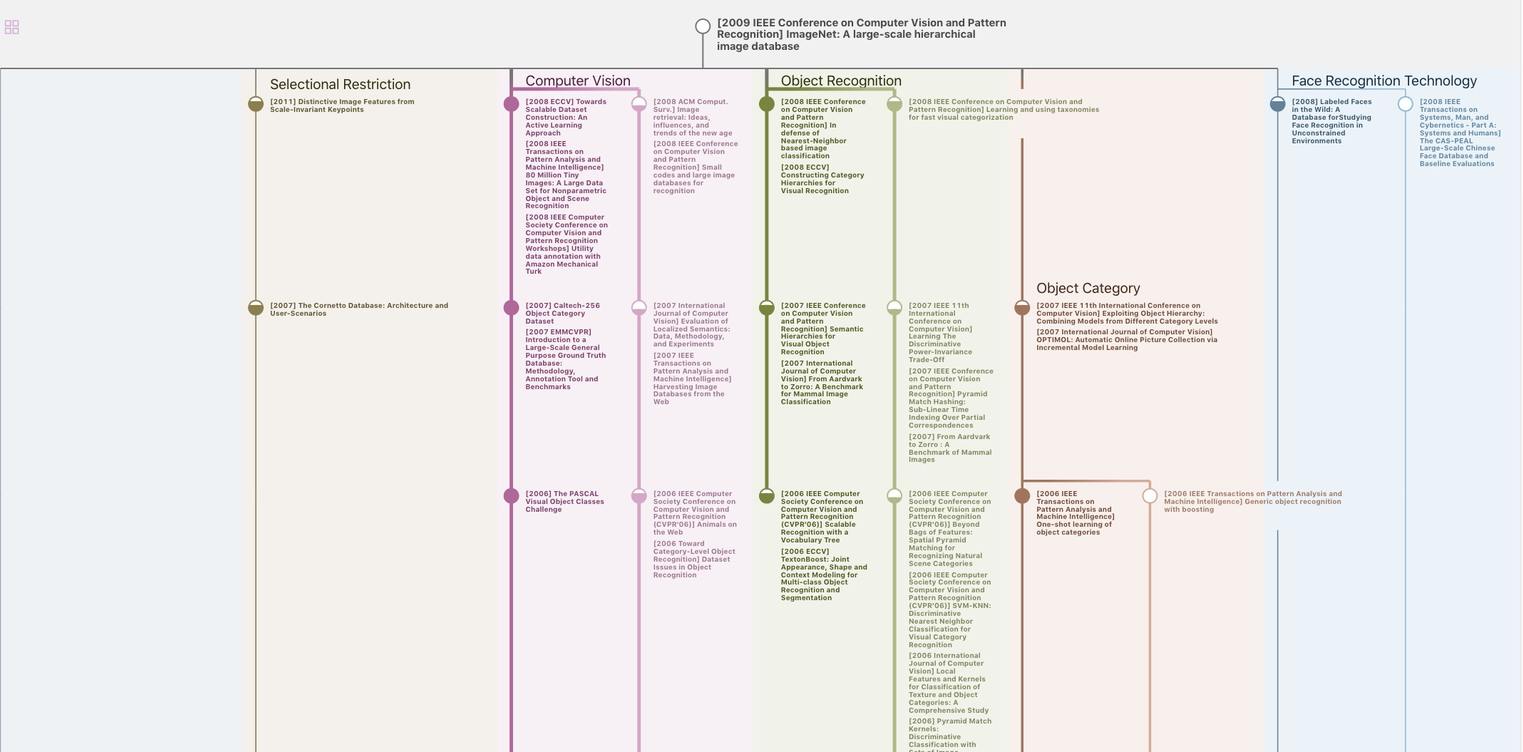
生成溯源树,研究论文发展脉络
Chat Paper
正在生成论文摘要