Mathematically aggregating experts' predictions of possible futures
crossref(2021)
摘要
Experts are often asked to represent their uncertainty as a subjective probability. Structured protocols offer a transparent and systematic way to elicit and combine probability judgements from multiple experts. As part of this process, experts are asked to individually estimate a probability (e.g., of a future event) which needs to be combined, or aggregated, into a final group prediction. The experts' judgements can be aggregated behaviourally (by striving for consensus), or mathematically (by using a mathematical rule to combine individual estimates). Mathematical rules (e.g., weighted linear combinations of judgments) provide an objective approach to aggregation. However, the choice of a rule is not straightforward, and the quality of the aggregated group judgement depends on it. The quality of an aggregation can be defined in terms of accuracy, calibration and informativeness. These measures can be used to compare different aggregation approaches and help decide on which aggregation produces the ``best” final prediction.In the ideal case, individual experts' performance (as probability assessors) would be scored on similar questions ahead of time, these scores translated into performance-based weights, and a performance-based weighted aggregation could then be used. When this is not possible though, several other aggregation methods, informed by measurable proxies for good performance, can be formulated and compared. Here, we develop a suite of aggregation methods, informed by previous experience and the available literature. Next, we investigate the relative performance of these aggregation methods using three datasets. Although the accuracy, calibration, and informativeness of the majority of methods are very similar, a couple of the aggregation methods consistently distinguish themselves as among the best or worst.
更多查看译文
AI 理解论文
溯源树
样例
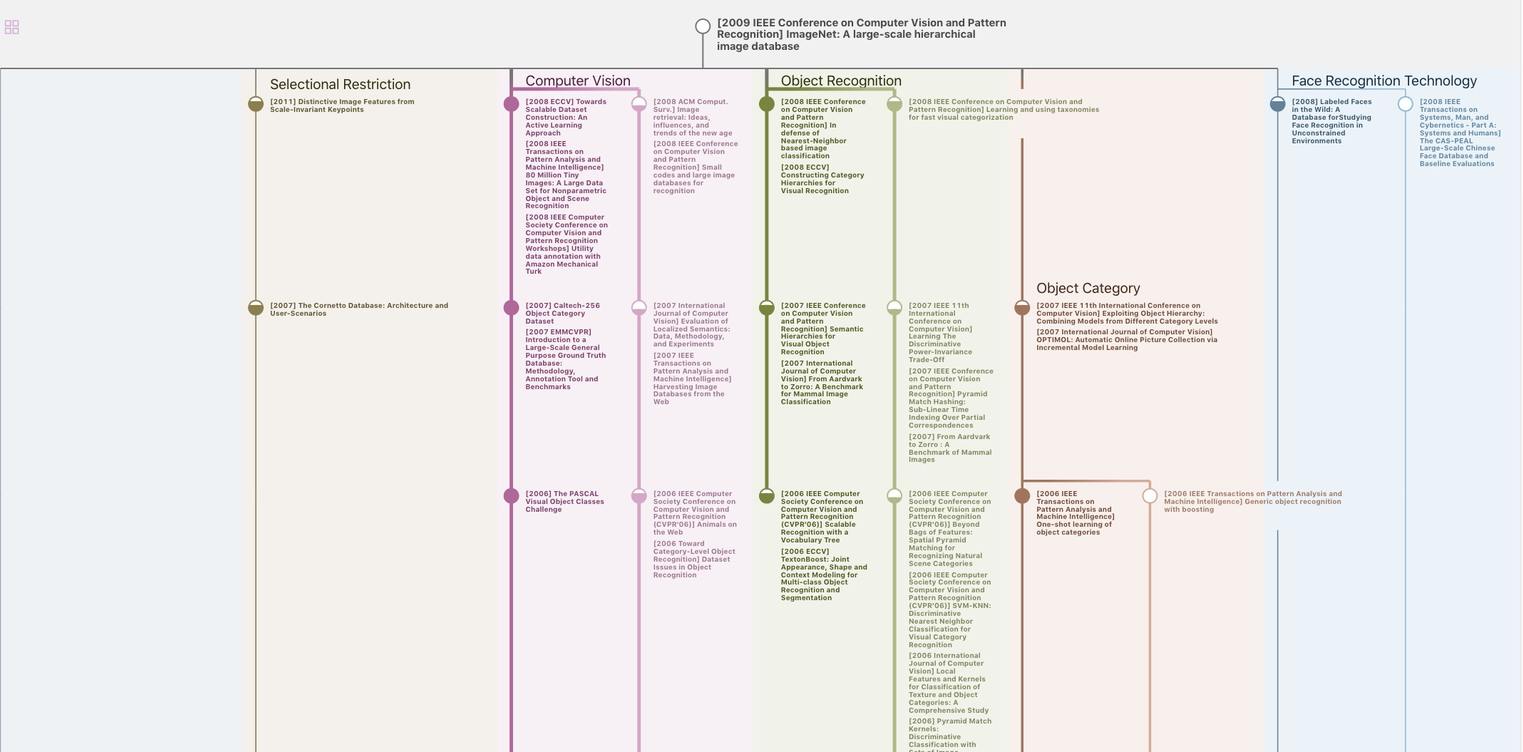
生成溯源树,研究论文发展脉络
Chat Paper
正在生成论文摘要