Evidence-based recommender system and experimental validation for high-entropy alloys
crossref(2021)
摘要
Abstract We present a data-driven approach to explore high-entropy alloys (HEAs). To overcome the challenges with numerous element-combination candidates, selecting appropriate descriptors, and the limitations and biased of existing data, we apply the evidence theory to develop a descriptor-free evidence-based recommender system (ERS) for recommending HEAs. The proposed system measures the similarities between element combinations and utilizes it to recommend potential HEAs. To evaluate the ERS, we compare its HEA-recommendation capability with those of matrix-factorization- and supervised-learning-based recommender systems on four widely known data sets, including binary and ternary alloys. The results of experiments using k-fold cross-validation on the data sets show that the ERS outperforms all competitors. Furthermore, the ERS shows excellent extrapolation capabilities in experiments of recommending quaternary and quinary HEAs. We experimentally validate the most strongly recommended Fe-Co-based magnetic HEA, viz. FeCoMnNi, and confirm that it shows a body-centered cubic structure and is stable at high temperatures.
更多查看译文
AI 理解论文
溯源树
样例
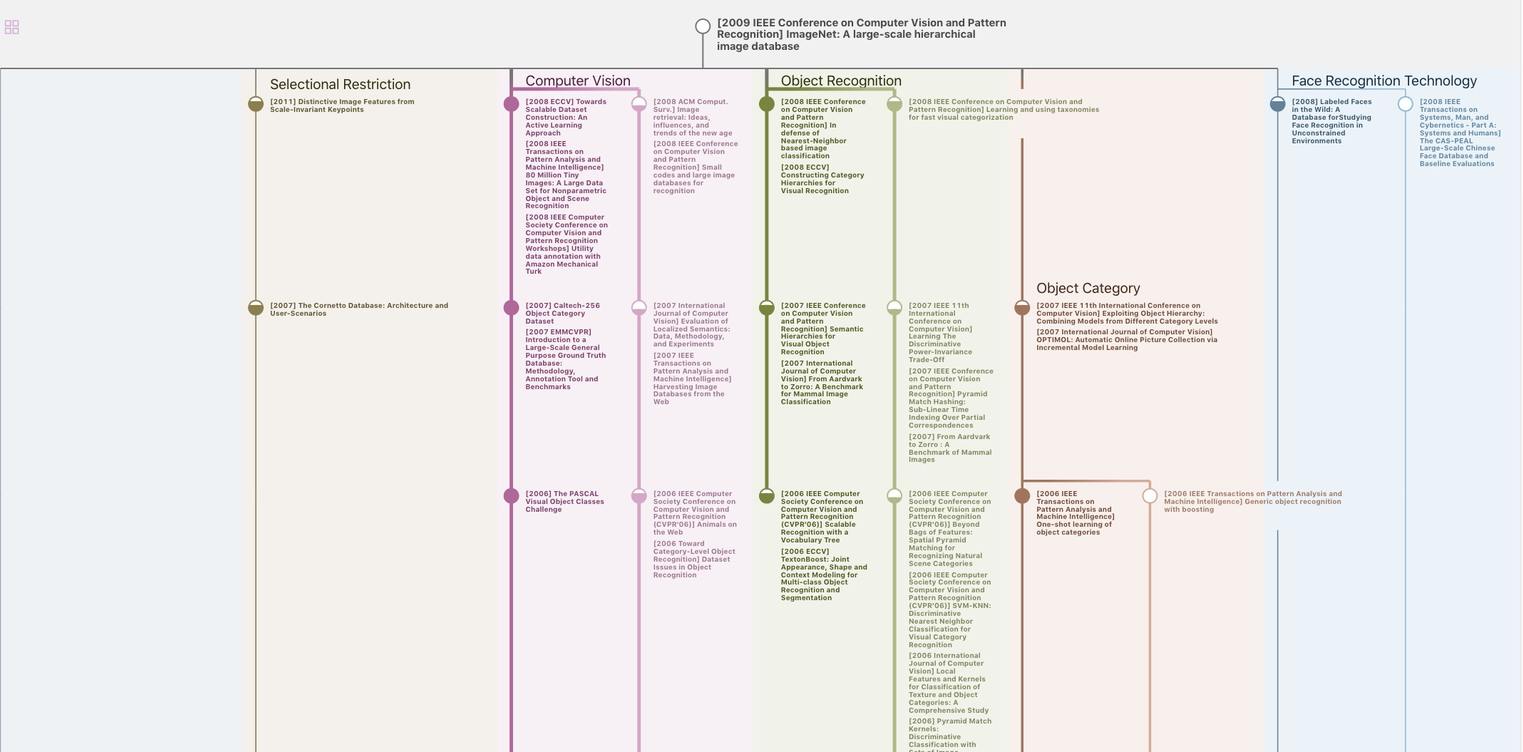
生成溯源树,研究论文发展脉络
Chat Paper
正在生成论文摘要