Time Series Imputation in Faulty Systems.
IbPRIA(2023)
摘要
Time series data has a crucial role in business. It reveals temporal trends and patterns, making it possible for decision-makers to make informed decisions and mitigate problems even before they happen. The existence of missing values in time series can bring difficulties in the analysis and lead to inaccurate conclusions. Thus, there is the need to solve this issue by performing missing data imputation on time series. In this work, we propose a Focalize KNN that takes advantage of time series properties to perform missing data imputation. The approach is tested with different methods, combinations of parameters and features. The results of the proposed approach, with overlap and disjoint missing patterns, show Focalize KNN is very beneficial in scenarios with disjoint missing patterns.
更多查看译文
关键词
time series imputation,time series,systems
AI 理解论文
溯源树
样例
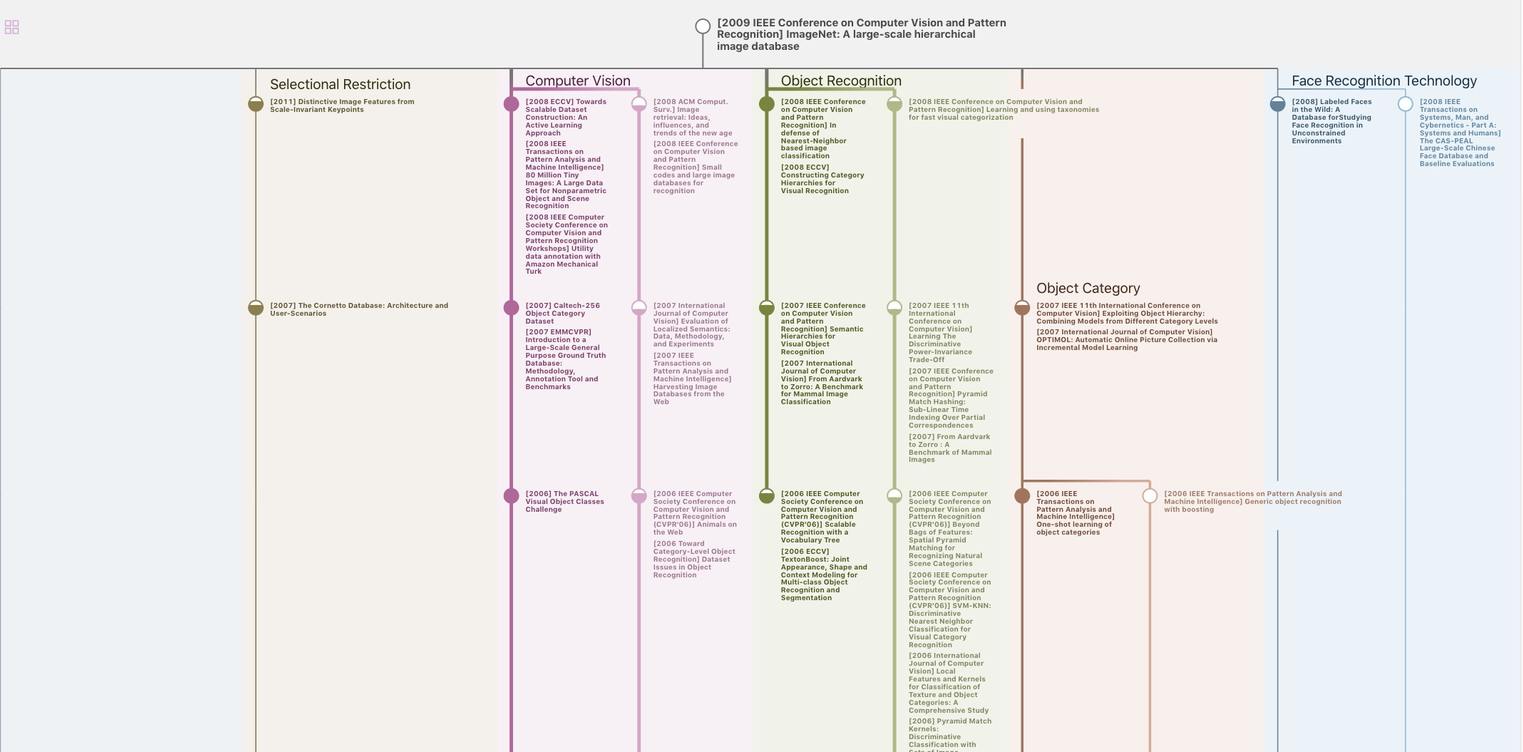
生成溯源树,研究论文发展脉络
Chat Paper
正在生成论文摘要