Hardware Compute Partitioning on NVIDIA GPUs.
RTAS(2023)
摘要
Embedded and autonomous systems are increasingly integrating AI/ML features, often enabled by a hardware accelerator such as a GPU. As these workloads become increasingly demanding, but size, weight, power, and cost constraints remain unyielding, ways to increase GPU capacity are an urgent need. In this work, we provide a means by which to spatially partition the computing units of NVIDIA GPUs transparently, allowing oft-idled capacity to be reclaimed via safe and efficient GPU sharing. Our approach works on any NVIDIA GPU since 2013, and can be applied via our easy-to-use, user-space library titled libsmctrl. We back the design of our system with deep investigations into the hardware scheduling pipeline of NVIDIA GPUs. We provide guidelines for the use of our system, and demonstrate it via an object detection case study using YOLOv2.
更多查看译文
关键词
real time systems,gpus,nvidia,cuda,yolov2,scheduling,linux,computer architecture,gpu architecture,spatial partitioning,spacial partitioning
AI 理解论文
溯源树
样例
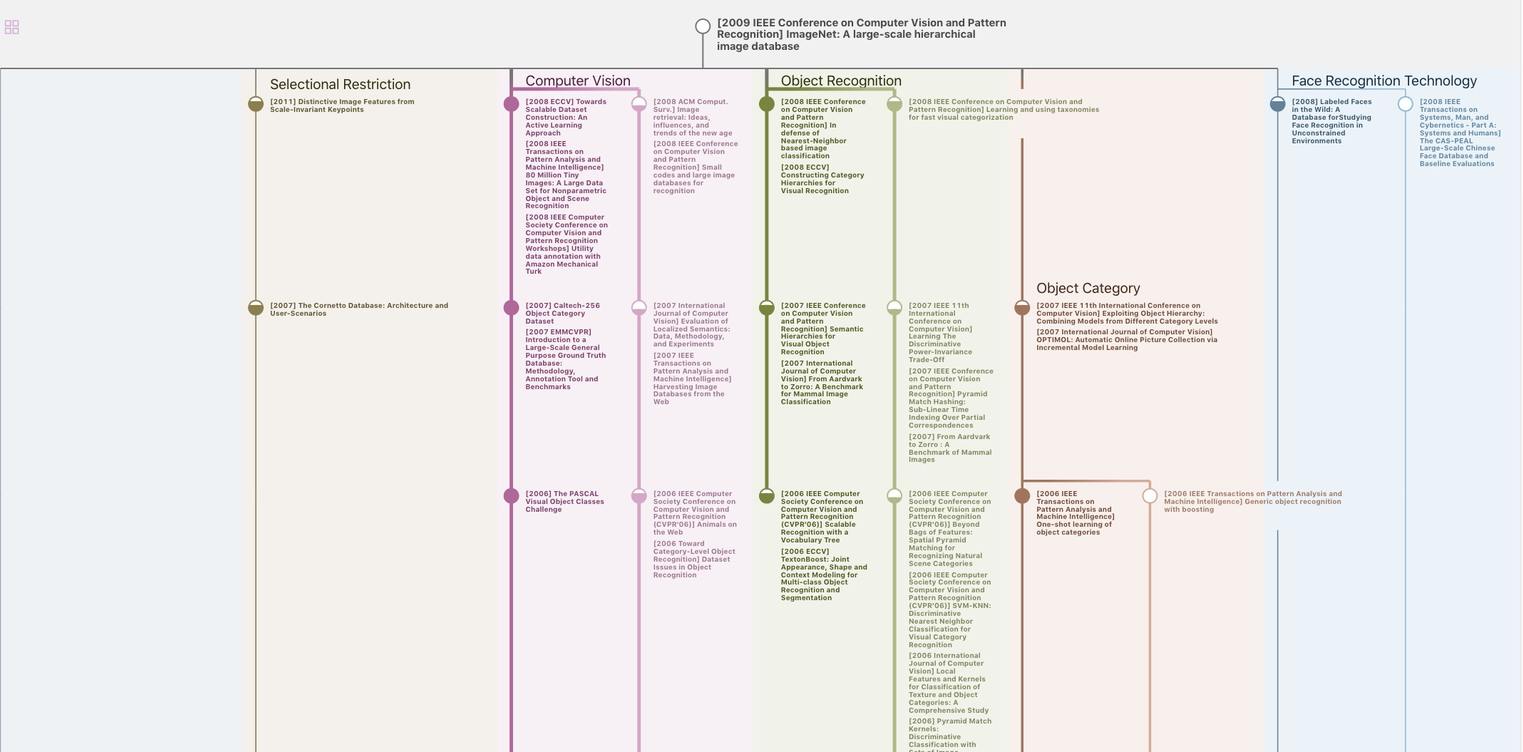
生成溯源树,研究论文发展脉络
Chat Paper
正在生成论文摘要