LoopTree: Enabling Exploration of Fused-layer Dataflow Accelerators.
ISPASS(2023)
摘要
Many accelerators today process deep neural networks layer by layer. As a consequence of this processing style, every intermediate feature map incurs expensive off-chip transfers. Layer fusion eliminates off-chip transfers of intermediate results, leading to better latency and energy efficiency. Prior works have explored only subsets of the fused-layer design space, looking only at a particular choice of tiling, scheduling, and buffering strategy. Their architectural models are also tailored for their proposed dataflow. The lack of a unified, systematic representation of designs and a versatile evaluation method has prevented thorough exploration of the design space. To enable systematic exploration of this design space, we present LoopTree, a framework for describing and evaluating any design in our expanded fused-layer dataflow design space. With a case study, we explore new designs to show that exploring our larger design space uncovers more efficient designs, especially for recent workloads with diverse layer types. Our design achieves 2.5x speedup and 2x lower energy compared to an optimized layer-by-layer design. Compared to a state-of-the-art fused-layer design, we match latency and energy while using 25% less onchip buffer space.
更多查看译文
关键词
analytical modeling,layer fusion,accelerators
AI 理解论文
溯源树
样例
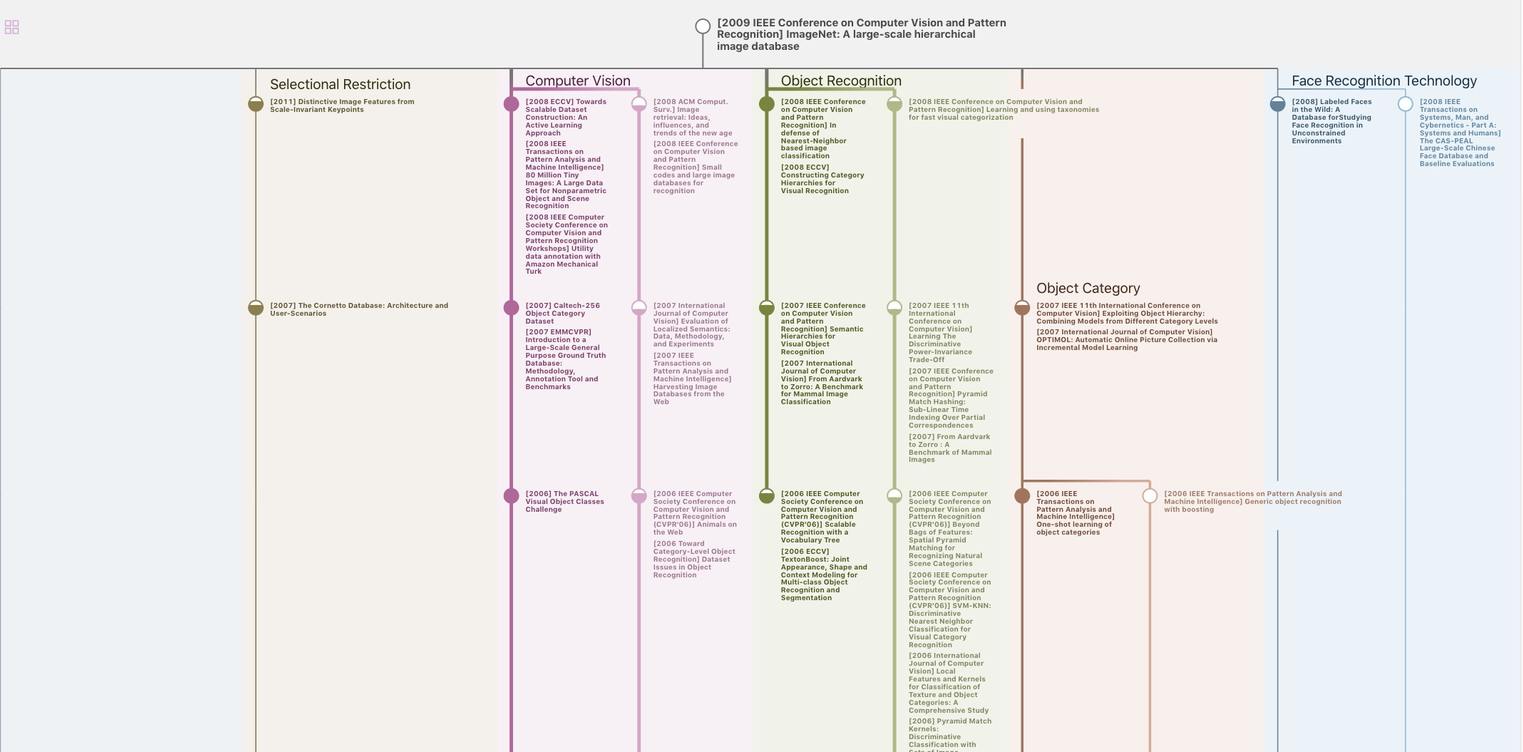
生成溯源树,研究论文发展脉络
Chat Paper
正在生成论文摘要