Assessment in Conversational Intelligent Tutoring Systems: Are Contextual Embeddings Really Better?
AIED (Posters/Late Breaking Results/...)(2023)
摘要
This research investigates the ability of semantic text models to assess student responses during tutoring compared with expert human judges. Recent interest in text similarity has led to a proliferation of models that can potentially be used for assessing student responses; however, whether these models perform as well as traditional distributional semantic models like Latent Semantic Analysis for student response assessment in automatic short answer grading is unclear. We assessed 5166 response pairings of 219 participants across 118 electronics questions and scored each with 13 different computational text models, including models that use regular expressions, distributional semantics, word embeddings, contextual embeddings, and combinations of these features. We show a few semantic text models performing comparably to Latent Semantic Analysis, and in some cases outperforming the model. Furthermore, combination models outperformed individual models in agreement with human judges. Choosing appropriate computational techniques and optimizing the text model may continue to improve the accuracy, recall, weighted agreement and therefore, the effectiveness of conversational ITSs.
更多查看译文
关键词
conversational intelligent tutoring systems,contextual embeddings,assessment
AI 理解论文
溯源树
样例
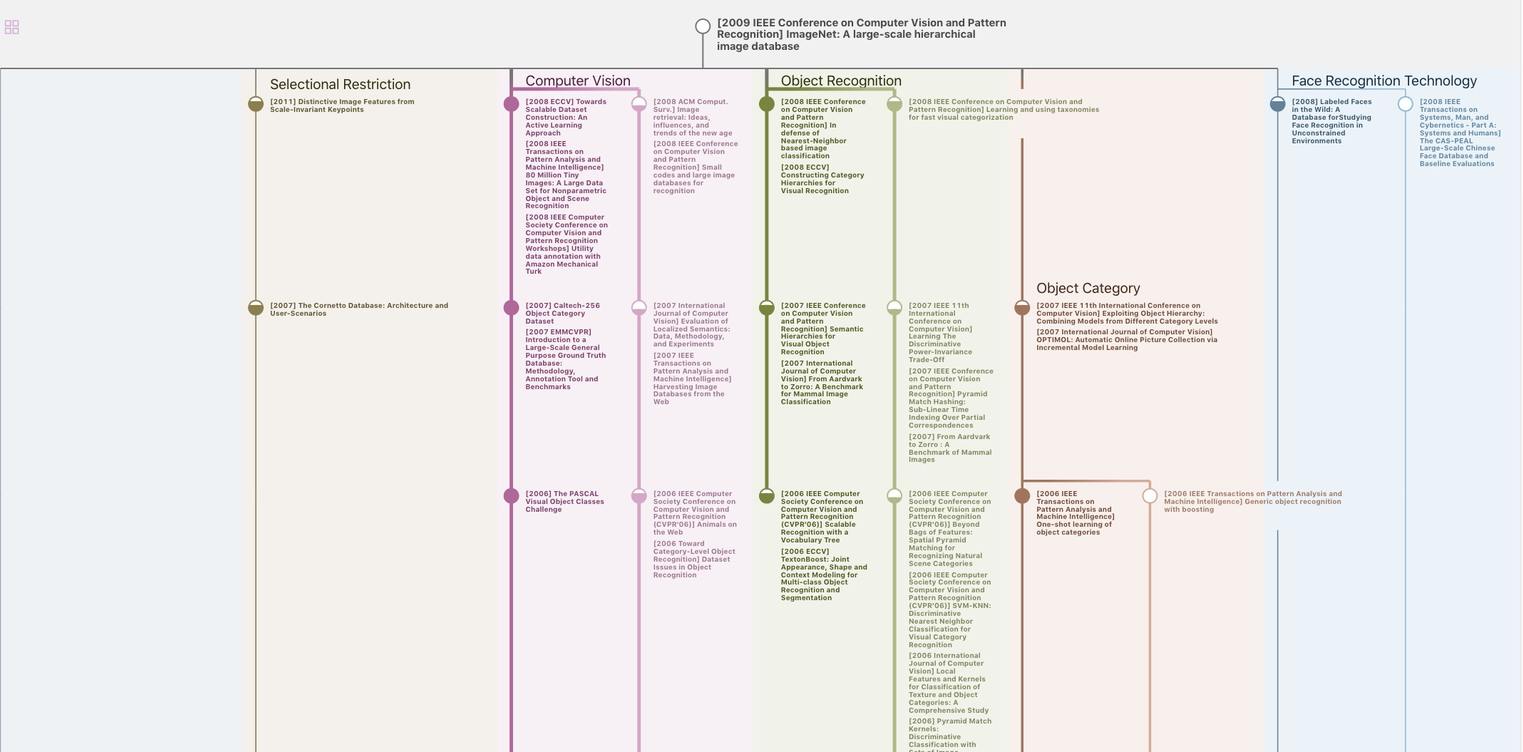
生成溯源树,研究论文发展脉络
Chat Paper
正在生成论文摘要