Real: A Representative Error-Driven Approach for Active Learning
MACHINE LEARNING AND KNOWLEDGE DISCOVERY IN DATABASES: RESEARCH TRACK, ECML PKDD 2023, PT I(2023)
摘要
Given a limited labeling budget, active learning (al) aims to sample the most informative instances from an unlabeled pool to acquire labels for subsequent model training. To achieve this, al typically measures the informativeness of unlabeled instances based on uncertainty and diversity. However, it does not consider erroneous instances with their neighborhood error density, which have great potential to improve the model performance. To address this limitation, we propose Real, a novel approach to select data instances with Representative Errors for Active Learning. It identifies minority predictions as pseudo errors within a cluster and allocates an adaptive sampling budget for the cluster based on estimated error density. Extensive experiments on five text classification datasets demonstrate that Real consistently outperforms all best-performing baselines regarding accuracy and F1-macro scores across a wide range of hyperparameter settings. Our analysis also shows that Real selects the most representative pseudo errors that match the distribution of ground-truth errors along the decision boundary. Our code is publicly available at https://github.com/withchencheng/ ECML PKDD 23 Real.
更多查看译文
关键词
Active learning,Text classification,Error-driven
AI 理解论文
溯源树
样例
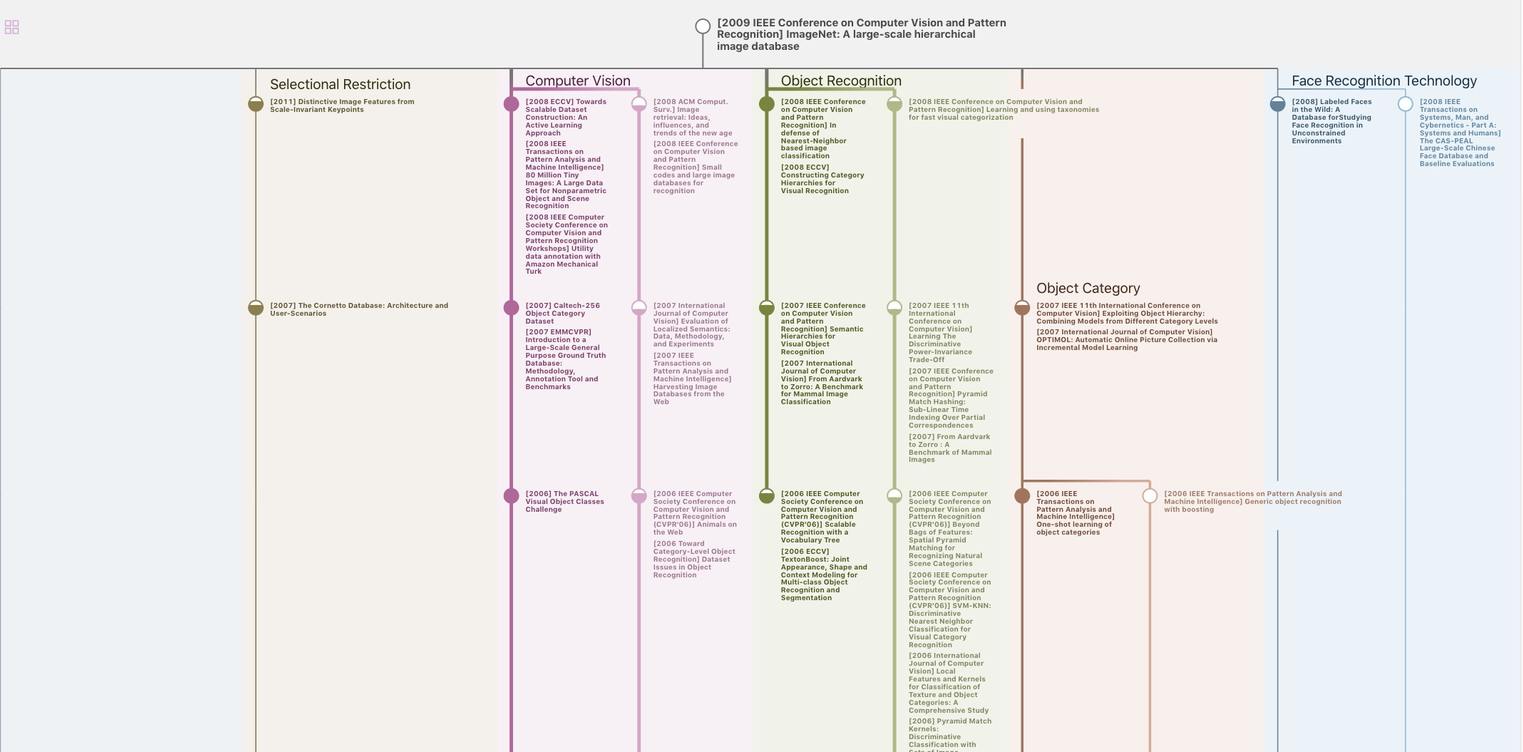
生成溯源树,研究论文发展脉络
Chat Paper
正在生成论文摘要