SSC3OD: Sparsely Supervised Collaborative 3D Object Detection from LiDAR Point Clouds
CoRR(2023)
摘要
Collaborative 3D object detection, with its improved interaction advantage among multiple agents, has been widely explored in autonomous driving. However, existing collaborative 3D object detectors in a fully supervised paradigm heavily rely on large-scale annotated 3D bounding boxes, which is labor-intensive and time-consuming. To tackle this issue, we propose a sparsely supervised collaborative 3D object detection framework SSC3OD, which only requires each agent to randomly label one object in the scene. Specifically, this model consists of two novel components, i.e., the pillar-based masked autoencoder (Pillar-MAE) and the instance mining module. The Pillar-MAE module aims to reason over high-level semantics in a self-supervised manner, and the instance mining module generates high-quality pseudo labels for collaborative detectors online. By introducing these simple yet effective mechanisms, the proposed SSC3OD can alleviate the adverse impacts of incomplete annotations. We generate sparse labels based on collaborative perception datasets to evaluate our method. Extensive experiments on three large-scale datasets reveal that our proposed SSC3OD can effectively improve the performance of sparsely supervised collaborative 3D object detectors.
更多查看译文
关键词
Point Cloud,LiDAR Point Clouds,3D Object Detection,Collaborative 3D,Multiple Agents,Objects In The Scene,Collaborative Framework,Pseudo Labels,Online Collaboration,Sparse Labeling,Self-supervised Manner,High-level Semantics,Training Set,Quantitative Results,Detection Performance,Detection Results,Autonomous Vehicles,Score Threshold,Perceptual Abilities,Pertinent Issues,Pre-trained Encoder,Positive Instances,Characteristics Of Agents,Pose Error,Point Cloud Information,Unlabeled Instances
AI 理解论文
溯源树
样例
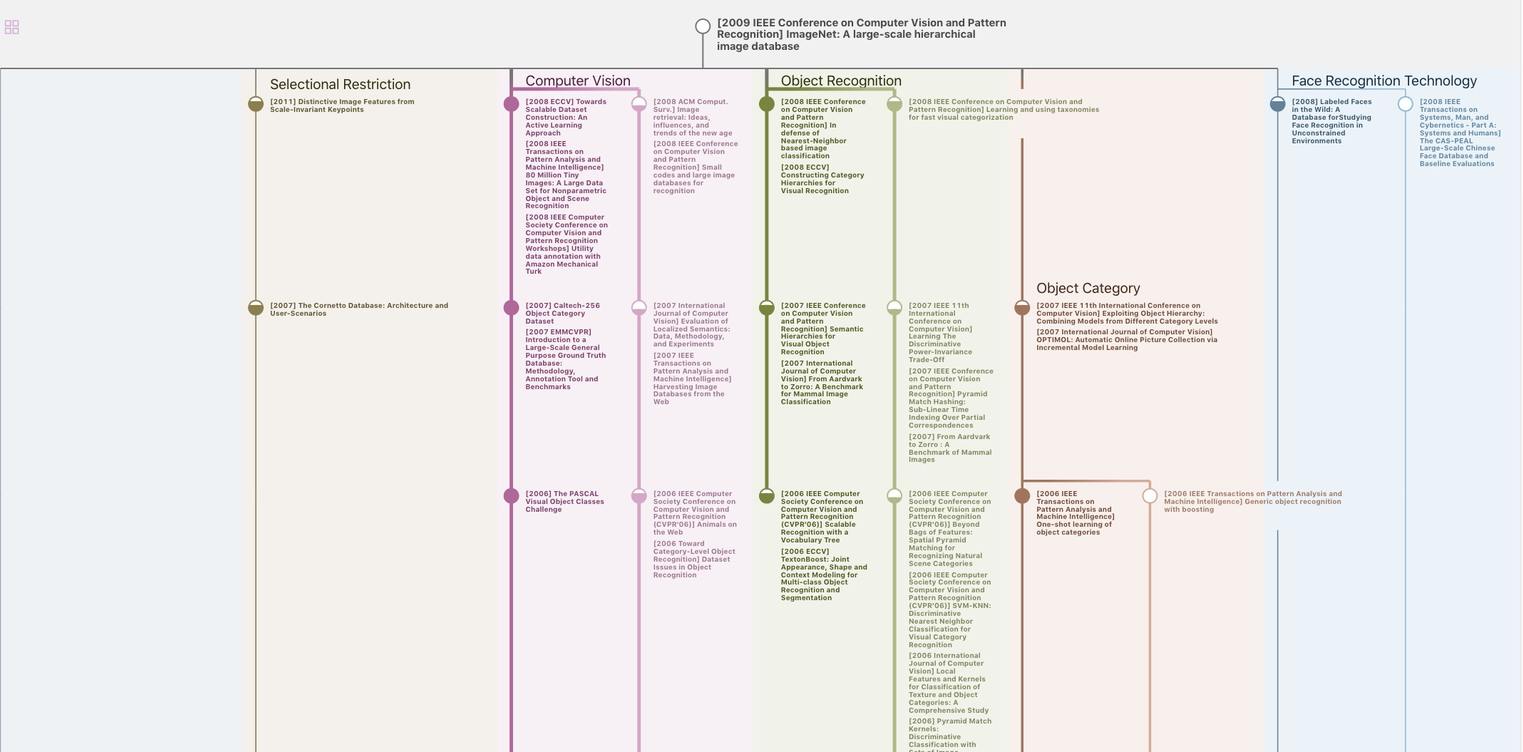
生成溯源树,研究论文发展脉络
Chat Paper
正在生成论文摘要