SMILE: Evaluation and Domain Adaptation for Social Media Language Understanding
PROCEEDINGS OF THE 29TH ACM SIGKDD CONFERENCE ON KNOWLEDGE DISCOVERY AND DATA MINING, KDD 2023(2023)
摘要
We study the ability of transformer-based language models (LMs) to understand social media language. Social media (SM) language is distinct from standard written language, yet existing benchmarks fall short of capturing LM performance in this socially, economically, and politically important domain. We quantify the degree to which social media language differs from conventional language and conclude that the difference is significant both in terms of token distribution and rate of linguistic shift. Next, we introduce a new benchmark for Social MedIa Language Evaluation (SMILE) that covers four SM platforms and eleven tasks. Finally, we show that learning a tokenizer and pretraining on a mix of social media and conventional language yields an LM that outperforms the best similar-sized alternative by 4.2 points on the overall SMILE score.
更多查看译文
关键词
language modeling,social media,transfer learning,T5,datasets,neural networks
AI 理解论文
溯源树
样例
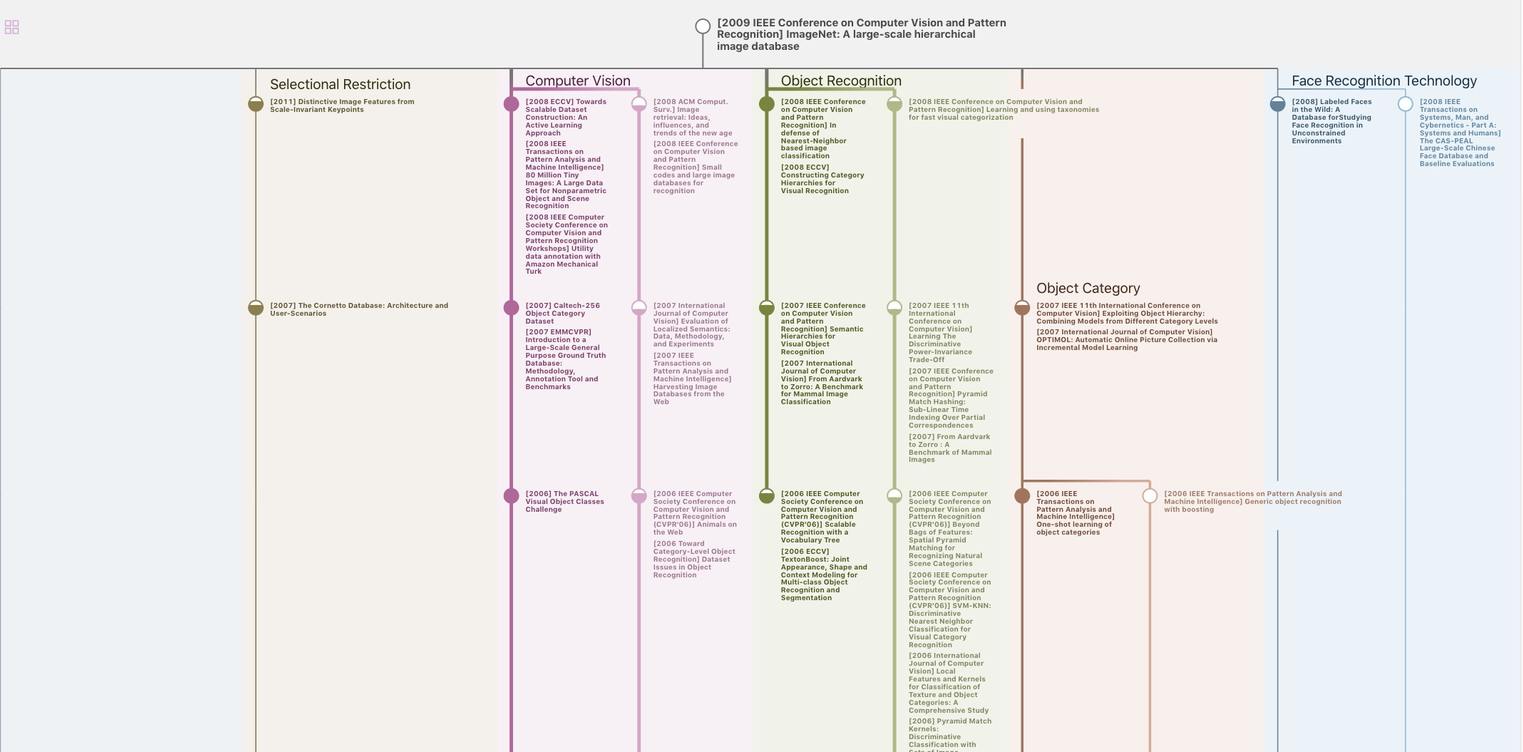
生成溯源树,研究论文发展脉络
Chat Paper
正在生成论文摘要