High-Precision Mapping of Soil Organic Matter Based on UAV Imagery Using Machine Learning Algorithms
DRONES(2023)
摘要
Soil organic matter (SOM) is a critical indicator of soil nutrient levels, and the precise mapping of its spatial distribution through remote sensing is essential for soil regulation, precise fertilization, and scientific management and protection. This information can offer decision support to agricultural management departments and various agricultural producers. In this paper, two new soil indices, NLIrededge2 and GDVI(rededge2), were proposed based on the sensitive spectral response characteristics of SOM in Northeast China. Nine parameters suitable for SOM mapping and modeling were determined using the competitive adaptive reweighted sampling (CARS) method, combined with spectrum reflectance, mathematical transformations of reflectance, vegetation indices, and so on. Then, utilizing unmanned aerial vehicle (UAV)-based multispectral images with centimeter-level resolution, a random forest machine learning algorithm was used to construct the inversion model of SOM and mapping SOM in the study area. The results showed that the random forest algorithm performed best for estimating SOM (R-2 = 0.91, RMSE = 0.95, MBE = 0.49, and RPIQ = 3.25) when compared with other machine learning algorithms such as support vector regression (SVR), elastic net, Bayesian ridge, and linear regression. The findings indicated a negative correlation between SOM content and altitude. The study concluded that the SOM modeling and mapping results could meet the needs of farmers to obtain basic information and provide a reference for UAVs to monitor SOM.
更多查看译文
关键词
soil organic matter, multispectral image, competitive adaptive reweighted sampling, random forest regression, unmanned aerial vehicle
AI 理解论文
溯源树
样例
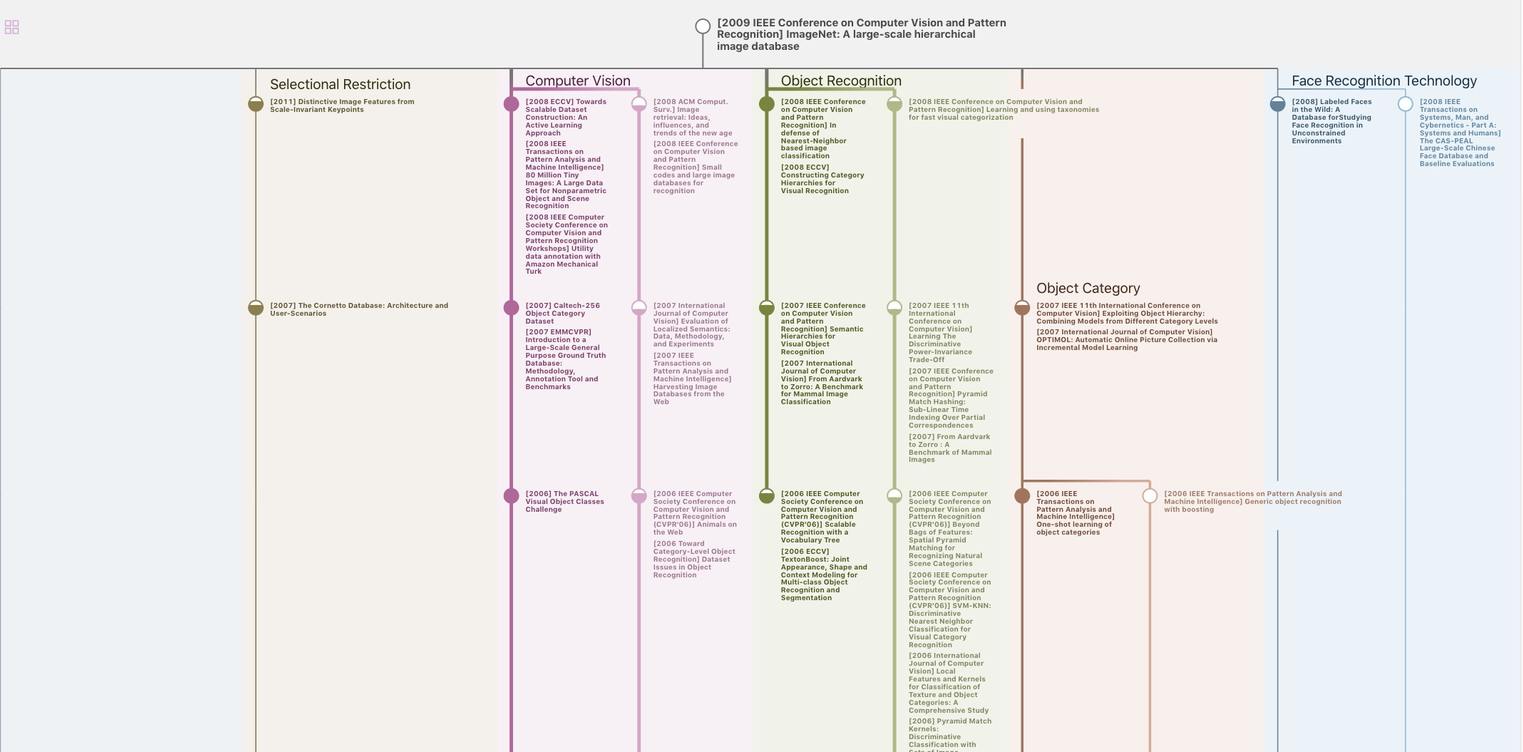
生成溯源树,研究论文发展脉络
Chat Paper
正在生成论文摘要