Convergence analysis of flow direction algorithm in continuous search space and its improvement
Mathematics and Computers in Simulation(2023)
摘要
The flow direction algorithm (FDA) is a new physics-based meta-heuristic optimization algorithm that is being used successfully in a variety of applications. However, FDA lacks theoretically rigorous convergency analysis and suffers from several drawbacks, such as premature convergence, lack of population diversity, and imbalance between exploitation and exploration. In this paper, the supermartingale convergence theorem is used to analyse the global convergence of FDA in a continuous search space. We first demonstrate that FDA’s global convergence is determined by the accumulation of the minimum probability (Pt∗) that the flow swarm fall into the global optimal region in each iteration. Then, an improved flow direction algorithm, namely, guided flow direction algorithm (GFDA), is proposed to increase the minimum probability by making full use of the neighbourhood information. Comprehensive experimental studies were conducted to test and validate the proposed GFDA. Twenty competitive meta-heuristic optimization algorithms, twenty-three classical benchmark functions, ten recently single objective bound constrained numerical optimization problems(CEC2020), and four constrained engineering problems were used. Finally, the experimental results and statistical tests (Friedman test and Wilcoxon test) demonstrate the superiority of GFDA compared to other algorithms.
更多查看译文
关键词
Flow direction algorithm (FDA),Supermartingale,Information utilization,Guiding flow (GF)
AI 理解论文
溯源树
样例
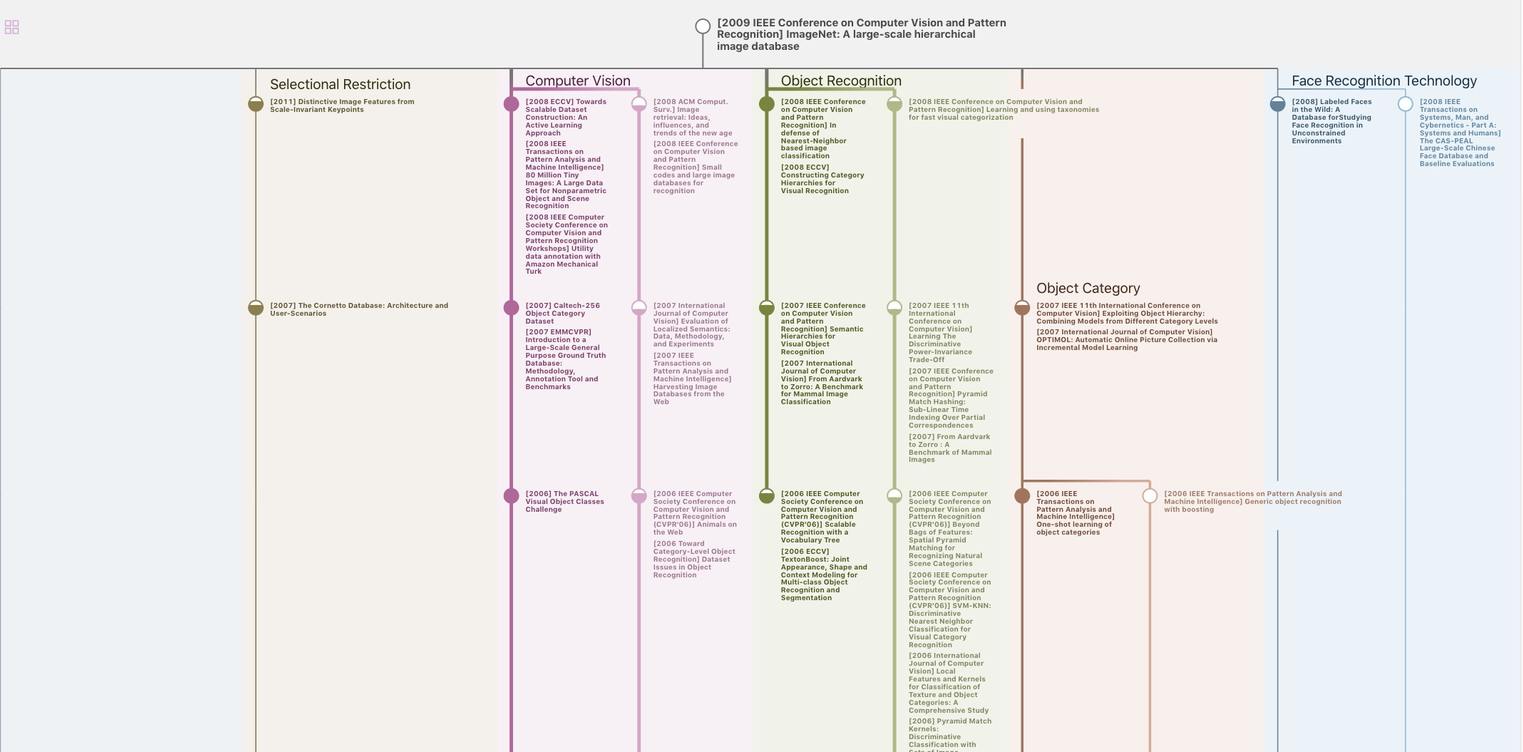
生成溯源树,研究论文发展脉络
Chat Paper
正在生成论文摘要