Dynamic scheduling for dual-objective job shop with machine breakdown by reinforcement learning
PROCEEDINGS OF THE INSTITUTION OF MECHANICAL ENGINEERS PART B-JOURNAL OF ENGINEERING MANUFACTURE(2024)
摘要
In modern complicated and changing manufacturing environments, unforeseen dynamic events such as machine breakdown or unexpected job arrival make required production resources unpredictable. The scheduling scheme is desired to maintain high stability in dynamic manufacturing environments. To cope with the classic disturbance of machine breakdown, a robust pro-active scheduling scheme is proposed by inserting the repair time into a disjunctive graph for reinforcement learning (IRDRL) in this paper. Firstly, a new mathematical model is developed to predict the machine fault which is assumed to be determined by service time and bearing load. Secondly, a disjunctive graph with breakdown information is designed to express the dynamic scheduling status. Then, an online scheduling framework is built based on the well-trained model through the proximal policy optimization (PPO) algorithm. Finally, compared with the classical methods such as the right-shift strategy and static model of reinforcement learning (RL), the proposed robust pro-active scheduling scheme is verified with high robustness, stability, and short running time.
更多查看译文
关键词
Dynamic manufacturing environment,machine breakdown,reinforcement learning,online scheduling framework,robust pro-active scheduling
AI 理解论文
溯源树
样例
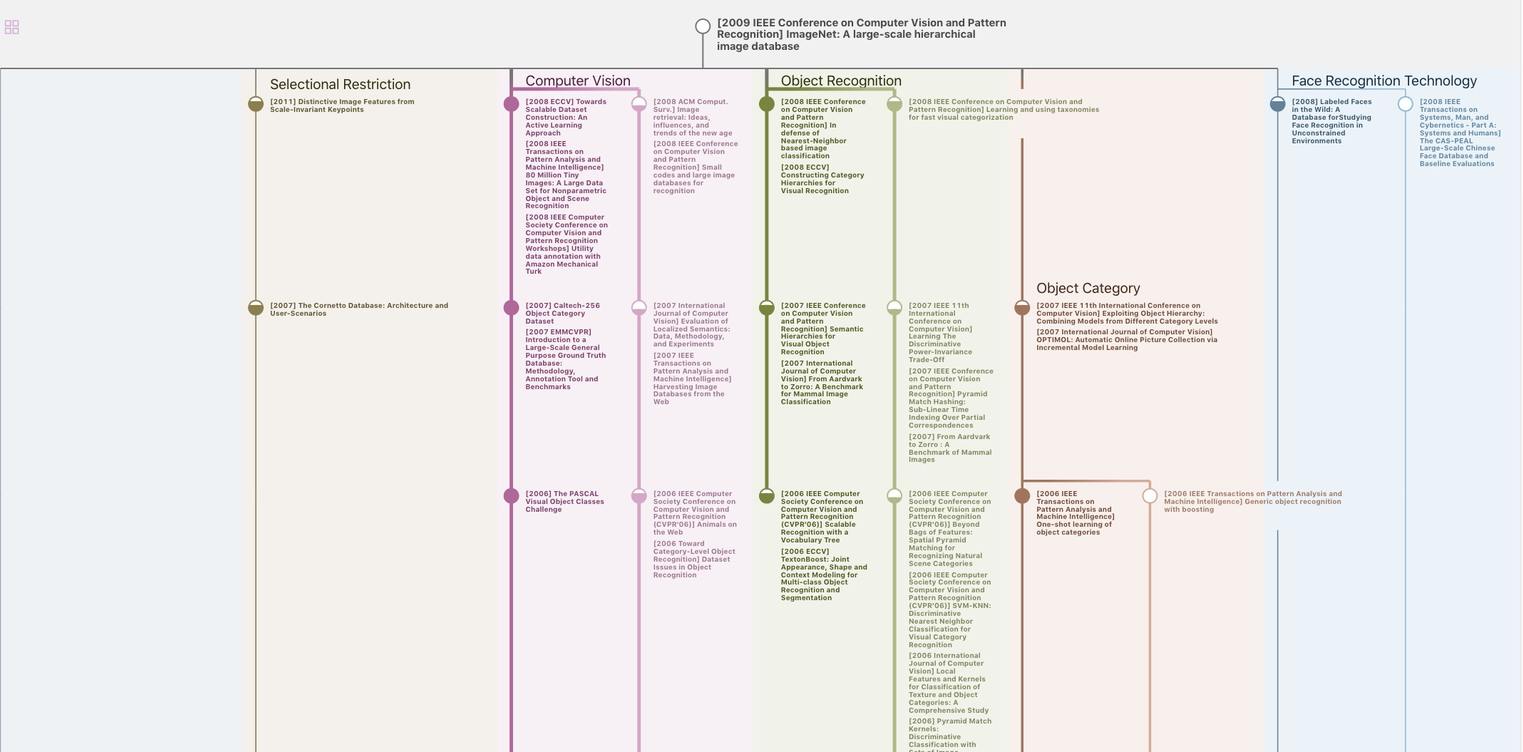
生成溯源树,研究论文发展脉络
Chat Paper
正在生成论文摘要