Probabilistic forecasting of daily COVID-19 admissions using machine learning
IMA JOURNAL OF MANAGEMENT MATHEMATICS(2023)
摘要
Accurate forecasts of daily Coronavirus-2019 (COVID-19) admissions are critical for healthcare planners and decision-makers to better manage scarce resources during and around infection peaks. Numerous studies have focused on forecasting COVID-19 admissions at the national or global levels. Localized predictions are vital, as they allow for resource planning redistribution, but also scarce and harder to get right. Several possible indicators can be used to predict COVID-19 admissions. The inherent variability in the admissions necessitates the generation and evaluation of the forecast distribution of admissions, as opposed to producing only a point forecast. In this study, we propose a quantile regression forest (QRF) model for probabilistic forecasting of daily COVID-19 admissions for a local hospital trust (aggregation of 3 hospitals), up to 7 days ahead, using a multitude of different predictors. We evaluate point forecast accuracy as well as the accuracy of the forecast distribution using appropriate measures. We provide evidence that QRF outperforms univariate time series methods and other more sophisticated benchmarks. Our findings also show that lagged admissions, total positive cases, daily tests performed, and Google grocery and Apple driving are the most salient predictors. Finally, we highlight areas where further research is needed.
更多查看译文
关键词
probabilistic forecasting,machine learning,daily
AI 理解论文
溯源树
样例
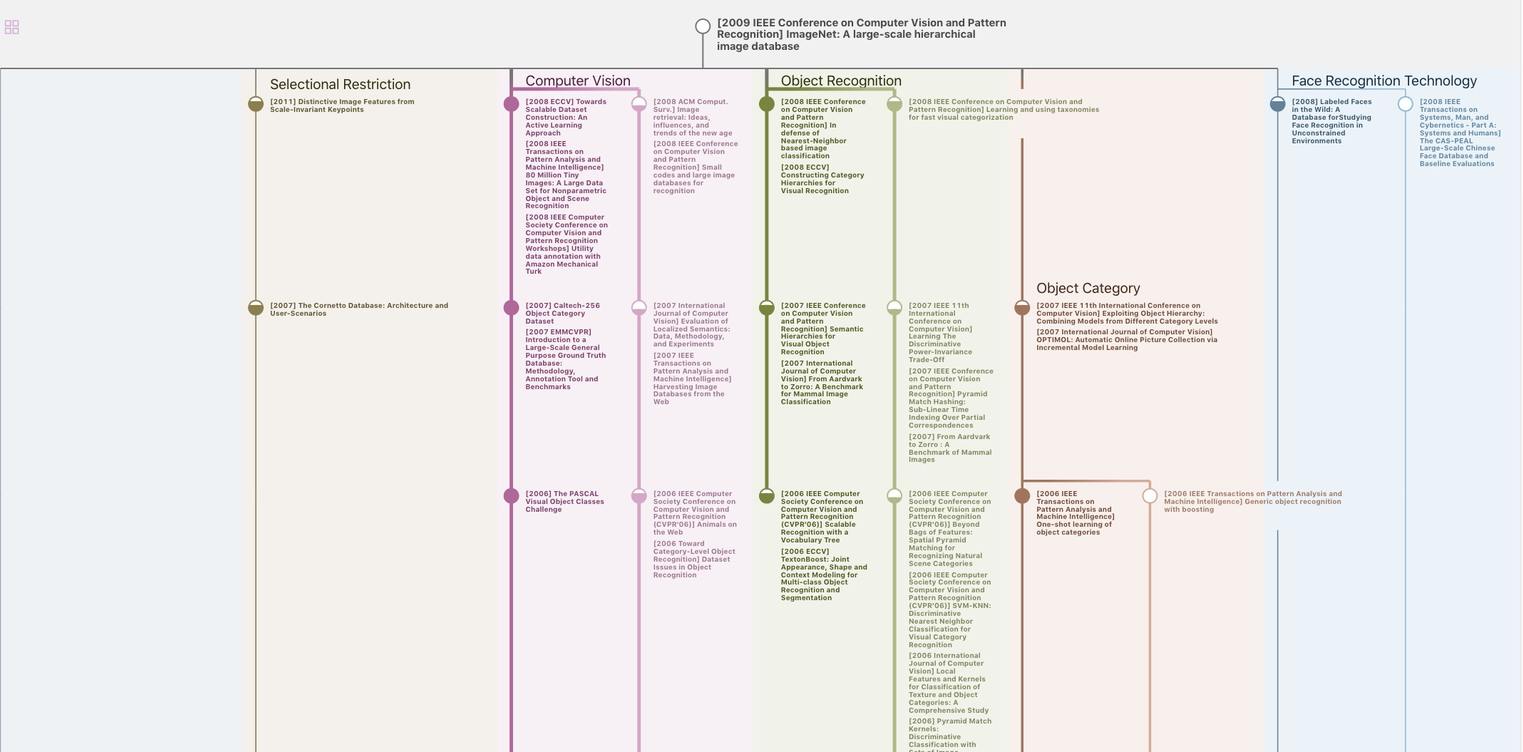
生成溯源树,研究论文发展脉络
Chat Paper
正在生成论文摘要