Integrating Machine Learning and Evidential Reasoning for User Profiling and Recommendation
JOURNAL OF SYSTEMS SCIENCE AND SYSTEMS ENGINEERING(2023)
摘要
User profiles representing users’ preferences and interests play an important role in many applications of personalized recommendation. With the rapid growth of social platforms, there is a critical need for efficient solutions to learn user profiles from the information they shared on social platforms so as to improve the quality of recommendation services. The problem of user profile learning is significantly challenging due to difficulty in handling data from multiple sources, in different formats and often associated with uncertainty. In this paper, we introduce an integrated approach that combines advanced Machine Learning techniques with evidential reasoning based on Dempster-Shafer theory of evidence for user profiling and recommendation. The developed methods for user profile learning and multi-criteria collaborative filtering are demonstrated with experimental results and analysis that show the effectiveness and practicality of the integrated approach. A proposal for extending multi-criteria recommendation systems by incorporating user profiles learned from different sources of data into the recommendation process so as to provide better recommendation capabilities is also highlighted.
更多查看译文
关键词
Machine learning,Dempster-Shafer theory of evidence,user profiles,personalized recommendation,preferences
AI 理解论文
溯源树
样例
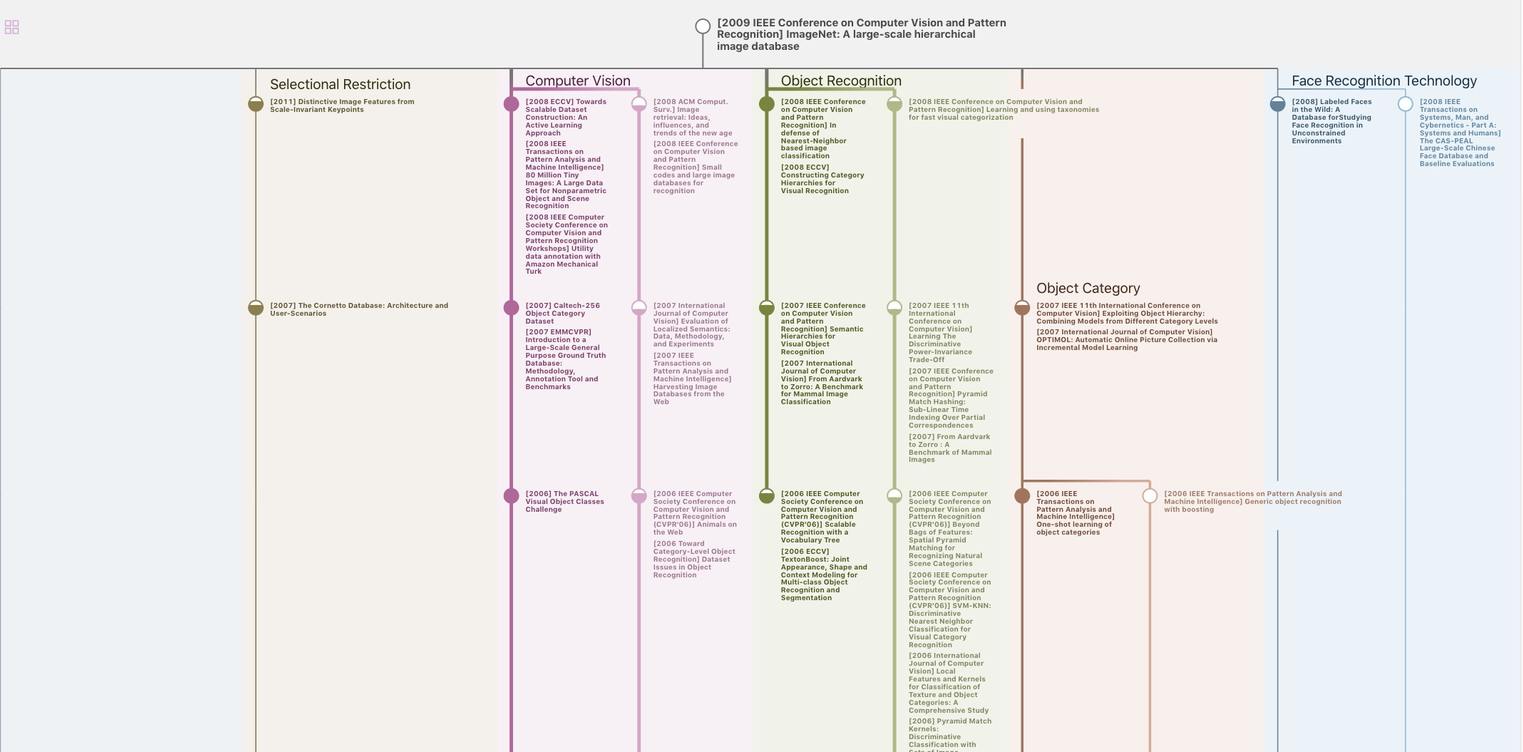
生成溯源树,研究论文发展脉络
Chat Paper
正在生成论文摘要