Bandwidth-aware adaptive chirp mode decomposition for railway bearing fault diagnosis
STRUCTURAL HEALTH MONITORING-AN INTERNATIONAL JOURNAL(2024)
摘要
It is a challenging task to accurately diagnose a railway bearing fault since bearing vibration signals are under strong interferences from wheel-rail excitations. The commonly used Kurtogram-based methods are often trapped in components induced by the wheel-rail excitations while adaptive mode decomposition methods are sensitive to input control parameters. To address these issues, based on a recently developed powerful signal decomposition method, that is, adaptive chirp mode decomposition (ACMD), a novel method called bandwidth-aware ACMD (BA-ACMD) is proposed in this article. First, the filter bank property of ACMD is thoroughly analyzed based on Monte-Carlo simulation and then a bandwidth expression with respect to the penalty parameter is first obtained by fitting a power law model. Then, a weighted spectrum trend (WST) method is proposed to partition frequency bands and then guide the parameter determination of ACMD through the integration of the obtained bandwidth expression. In addition, according to the order of magnitude of the WST in each band, the BA-ACMD adopts a recursive framework to extract signal modes one by one. In this way, dominating signal modes related to wheel-rail excitations can be extracted and then subtracted from the vibration signal in advance so that the bearing faults induced signal modes can be successfully identified. Both simulation and experimental validations are conducted showing that BA-ACMD can effectively detect single and compound faults of railway bearings under strong wheel-rail excitations.
更多查看译文
关键词
Rolling bearing,fault diagnosis,railway vehicle,spectrum trend,resonance band
AI 理解论文
溯源树
样例
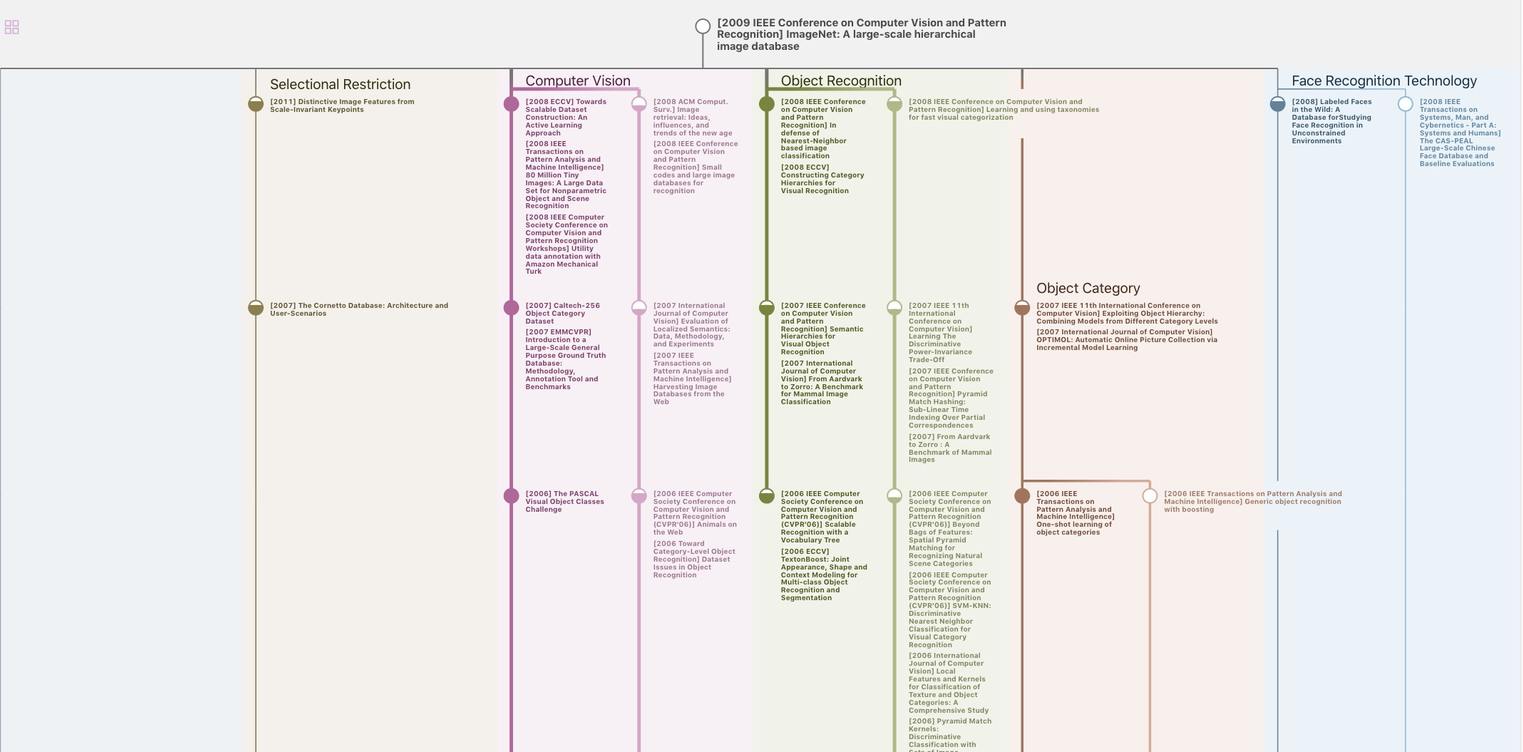
生成溯源树,研究论文发展脉络
Chat Paper
正在生成论文摘要