Representing Blurred Image without Deblurring
MATHEMATICS(2023)
摘要
The effective recognition of patterns from blurred images presents a fundamental difficulty for many practical vision tasks. In the era of deep learning, the main ideas to cope with this difficulty are data augmentation and deblurring. However, both facing issues such as inefficiency, instability, and lack of explainability. In this paper, we explore a simple but effective way to define invariants from blurred images, without data augmentation and deblurring. Here, the invariants are designed from Fractional Moments under Projection operators (FMP), where the blur invariance and rotation invariance are guaranteed by the general theorem of blur invariants and the Fourier-domain rotation equivariance, respectively. In general, the proposed FMP not only bears a simpler explicit definition, but also has useful representation properties including orthogonality, statistical flexibility, as well as the combined invariance of blurring and rotation. Simulation experiments are provided to demonstrate such properties of our FMP, revealing the potential for small-scale robust vision problems.
更多查看译文
关键词
image representation, invariants, blur, robustness, projection
AI 理解论文
溯源树
样例
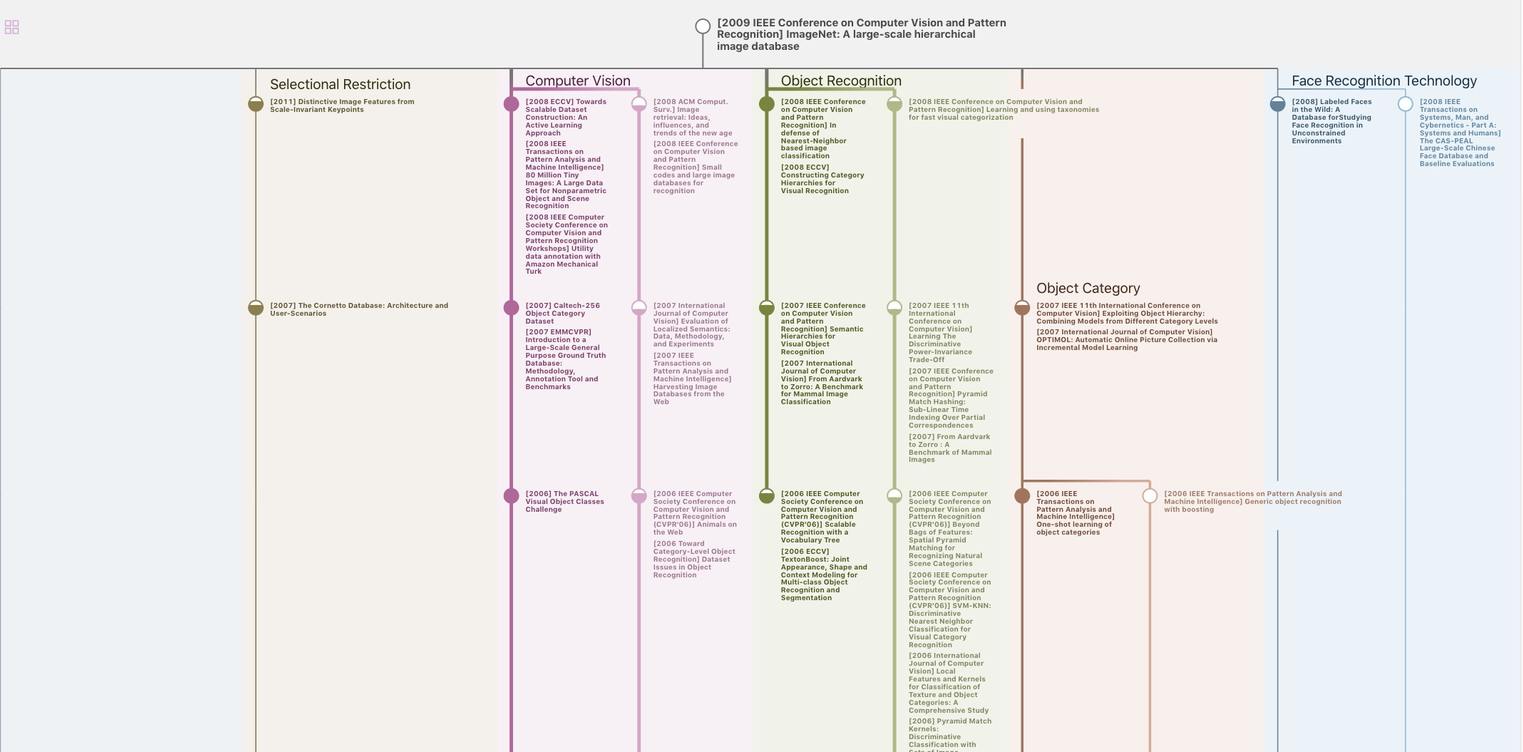
生成溯源树,研究论文发展脉络
Chat Paper
正在生成论文摘要