Rational design and glass-forming ability prediction of bulk metallic glasses via interpretable machine learning
JOURNAL OF MATERIALS SCIENCE(2023)
摘要
The prediction accuracy of current mainstream machine learning (ML) models depends on regulating many hyperparameters. In this paper, a deep forest (DF) model with a few hyperparameters and a non-excessive dependence on super parameter regulation was applied to the prediction of glass-forming ability (GFA) of bulk metallic glasses (BMGs). Compared with these of the mainstream ML models, including Support Vector Regression (SVR), random forest (RF), gradient boosted decision trees (GBDT), k-nearest neighbor (KNN), and eXtreme gradient boosting (XGBoost), the tenfold cross-validation shows that the determination coefficient ( R 2 ) of our suggested DF model is improved by 10.4
更多查看译文
关键词
bulk metallic glasses,ability prediction,machine learning,glass-forming
AI 理解论文
溯源树
样例
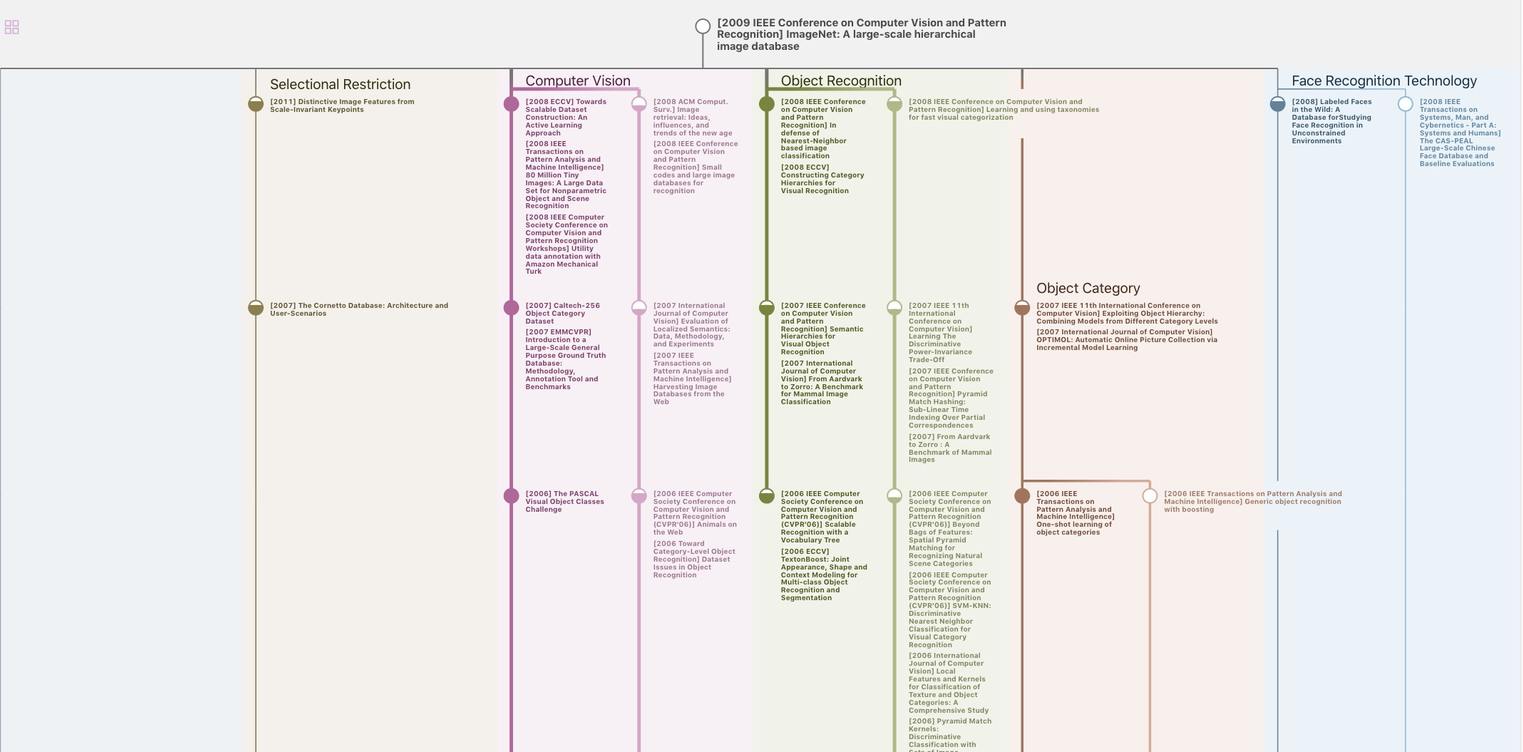
生成溯源树,研究论文发展脉络
Chat Paper
正在生成论文摘要