Can we and should we use artificial intelligence for formative assessment in science?
JOURNAL OF RESEARCH IN SCIENCE TEACHING(2023)
摘要
Journal of Research in Science TeachingEarly View COMMENT Can we and should we use artificial intelligence for formative assessment in science? Tingting Li, Corresponding Author Tingting Li [email protected] orcid.org/0000-0002-5692-2042 Department of Counseling, Educational Psychology, and Special Education, College of Education, Michigan State University, East Lansing, Michigan, USA CREATE for STEM Institute, College of Education, Michigan State University, East Lansing, Michigan, USA Correspondence Tingting Li, Department of Counseling, Educational Psychology, and Special Education, College of Education, Michigan State University, East Lansing, MI, USA. Email: [email protected]Search for more papers by this authorEmily Reigh, Emily Reigh orcid.org/0000-0003-0922-3537 Berkeley School of Education, University of California, Berkeley, California, USASearch for more papers by this authorPeng He, Peng He orcid.org/0000-0002-2877-0117 Department of Counseling, Educational Psychology, and Special Education, College of Education, Michigan State University, East Lansing, Michigan, USA CREATE for STEM Institute, College of Education, Michigan State University, East Lansing, Michigan, USASearch for more papers by this authorEmily Adah Miller, Emily Adah Miller orcid.org/0000-0003-3473-5729 Mary Frances Early College of Education, University of Georgia, Athens, Georgia, USASearch for more papers by this author Tingting Li, Corresponding Author Tingting Li [email protected] orcid.org/0000-0002-5692-2042 Department of Counseling, Educational Psychology, and Special Education, College of Education, Michigan State University, East Lansing, Michigan, USA CREATE for STEM Institute, College of Education, Michigan State University, East Lansing, Michigan, USA Correspondence Tingting Li, Department of Counseling, Educational Psychology, and Special Education, College of Education, Michigan State University, East Lansing, MI, USA. Email: [email protected]Search for more papers by this authorEmily Reigh, Emily Reigh orcid.org/0000-0003-0922-3537 Berkeley School of Education, University of California, Berkeley, California, USASearch for more papers by this authorPeng He, Peng He orcid.org/0000-0002-2877-0117 Department of Counseling, Educational Psychology, and Special Education, College of Education, Michigan State University, East Lansing, Michigan, USA CREATE for STEM Institute, College of Education, Michigan State University, East Lansing, Michigan, USASearch for more papers by this authorEmily Adah Miller, Emily Adah Miller orcid.org/0000-0003-3473-5729 Mary Frances Early College of Education, University of Georgia, Athens, Georgia, USASearch for more papers by this author First published: 10 May 2023 https://doi.org/10.1002/tea.21867Citations: 1 All authors have made equal and significant contributions to this paper. Read the full textAboutPDF ToolsRequest permissionExport citationAdd to favoritesTrack citation ShareShare Give accessShare full text accessShare full-text accessPlease review our Terms and Conditions of Use and check box below to share full-text version of article.I have read and accept the Wiley Online Library Terms and Conditions of UseShareable LinkUse the link below to share a full-text version of this article with your friends and colleagues. Learn more.Copy URL REFERENCES Black, P., & Wiliam, D. (2009). Developing the theory of formative assessment. Educational Assessment, Evaluation and Accountability (Formerly: Journal of Personnel Evaluation in Education), 21, 5– 31. Cheuk, T. (2021). Can AI be racist? Color-evasiveness in the application of machine learning to science assessments. Science Education, 105(5), 825– 836. https://doi.org/10.1002/sce.21671 Furtak, E. M., Heredia, S. C., & Morrison, D. (2019). Formative assessment in science education: Mapping a shifting terrain. In Handbook of formative assessment in the disciplines (pp. 97– 125). Routledge. Haverly, C., Calabrese Barton, A., Schwarz, C. V., & Braaten, M. (2020). “Making space”: How novice teachers create opportunities for equitable sense-making in elementary science. Journal of Teacher Education, 71(1), 63– 79. https://doi.org/10.1177/0022487118800706 Holstein, K., Wortman Vaughan, J., Daumé, H., III, Dudik, M., & Wallach, H. (2019, May). Improving fairness in machine learning systems: What do industry practitioners need? In Proceedings of the 2019 CHI conference on human factors in computing systems (pp. 1–16). Ladson-Billings, G. (1995). Toward a theory of culturally relevant pedagogy. American Educational Research Journal, 32(3), 465– 491. Li, C., Xing, W., & Leite, W. (2022). Using fair AI to predict students' math learning outcomes in an online platform. Interactive Learning Environments, 1–20, 1– 20. https://doi.org/10.1080/10494820.2022.2115076 Li, T., & Adah Miller, E. (n.d.). Culturally and linguistically “blind” or biased? Challenges for AI scoring of multilingual elementary students' hand-drawn scientific models. (manuscript under preparation). Mensah, F. M. (2022). “Now, I see”: Multicultural science curriculum as transformation and social action. The Urban Review, 54(1), 155– 181. Miller, E. C., Severance, S., & Krajcik, J. (2021). Motivating teaching, sustaining change in practice: Design principles for teacher learning in project-based learning contexts. Journal of Science Teacher Education, 32(7), 757– 779. https://doi.org/10.1080/1046560X.2020.1864099 Nasir, N. S., Rosebery, A. S., Warren, B., & Lee, C. D. (2014). Learning as a cultural process: Achieving equity through diversity. In R. K. Sawyer (Ed.), The Cambridge handbook of the learning sciences (pp. 686– 706). Cambridge University Press. National Research Council. (2000). Inquiry and the national science education standards: A guide for teaching and learning. National Academies Press. Nehm, R. H., Ha, M., & Mayfield, E. (2012). Transforming biology assessment with machine learning: Automated scoring of written evolutionary explanations. Journal of Science Education and Technology, 21(1), 183– 196. Noble, T., Suarez, C., Rosebery, A., O'Connor, M. C., Warren, B., & Hudicourt-Barnes, J. (2012). “I never thought of it as freezing”: How students answer questions on large scale science tests and what they know about science. Journal of Research in Science Teaching, 49(6), 778– 803. https://doi.org/10.1002/tea.21026 Riley, A. D., & Mensah, F. M. (2023). “My curriculum has no soul!”: A case study of the experiences of black women science teachers working at charter schools. Journal of Science Teacher Education, 34(1), 86–103. Rosebery, A. S., Ogonowski, M., DiSchino, M., & Warren, B. (2010). “The coat traps all your body heat”: Heterogeneity as fundamental to learning. The Journal of the Learning Sciences, 19(3), 322– 357. Rosebery, A. S., Warren, B., & Tucker-Raymond, E. (2016). Developing interpretive power in science teaching. Journal of Research in Science Teaching, 53(10), 1571– 1600. Schwarz, C. V., Ke, L., Salgado, M., & Manz, E. (2022). Beyond assessing knowledge about models and modeling: Moving toward expansive, meaningful, and equitable modeling practice. Journal of Research in Science Teaching, 59, 1086– 1096. Selwyn, N. (2019). Should robots replace teachers? AI and the future of education. John Wiley & Sons. Wang, J. R., Kao, H. L., & Lin, S. W. (2010). Preservice teachers' initial conceptions about assessment of science learning: The coherence with their views of learning science. Teaching and Teacher Education, 26(3), 522– 529. Yao, L., Cahill, A., & McCaffrey, D. F. (2020). The impact of training data quality on automated content scoring performance. In The AAAI workshop on artificial intelligence for education. Semantic Scholar. Retrieved May 7, 2023, from https://www.semanticscholar.org/paper/The-Impact-of-Training-Data-Quality-on-Automated-Yao-Cahill/8a7a0a47dd4fd989fb4431ffed2a1b3ec4b02556. Zhai, X., He, P., & Krajcik, J. (2022). Applying machine learning to automatically assess scientific models. Journal of Research in Science Teaching, 59(10), 1765– 1794. https://doi.org/10.1002/tea.21773 Zhai, X., Krajcik, J., & Pellegrino, J. W. (2021). On the validity of machine learning-based next generation science assessments: A validity inferential network. Journal of Science Education and Technology, 30, 298– 312. https://doi.org/10.1007/s10956-020-09879-9 Citing Literature Early ViewOnline Version of Record before inclusion in an issue ReferencesRelatedInformation
更多查看译文
关键词
formative assessment,artificial intelligence,science
AI 理解论文
溯源树
样例
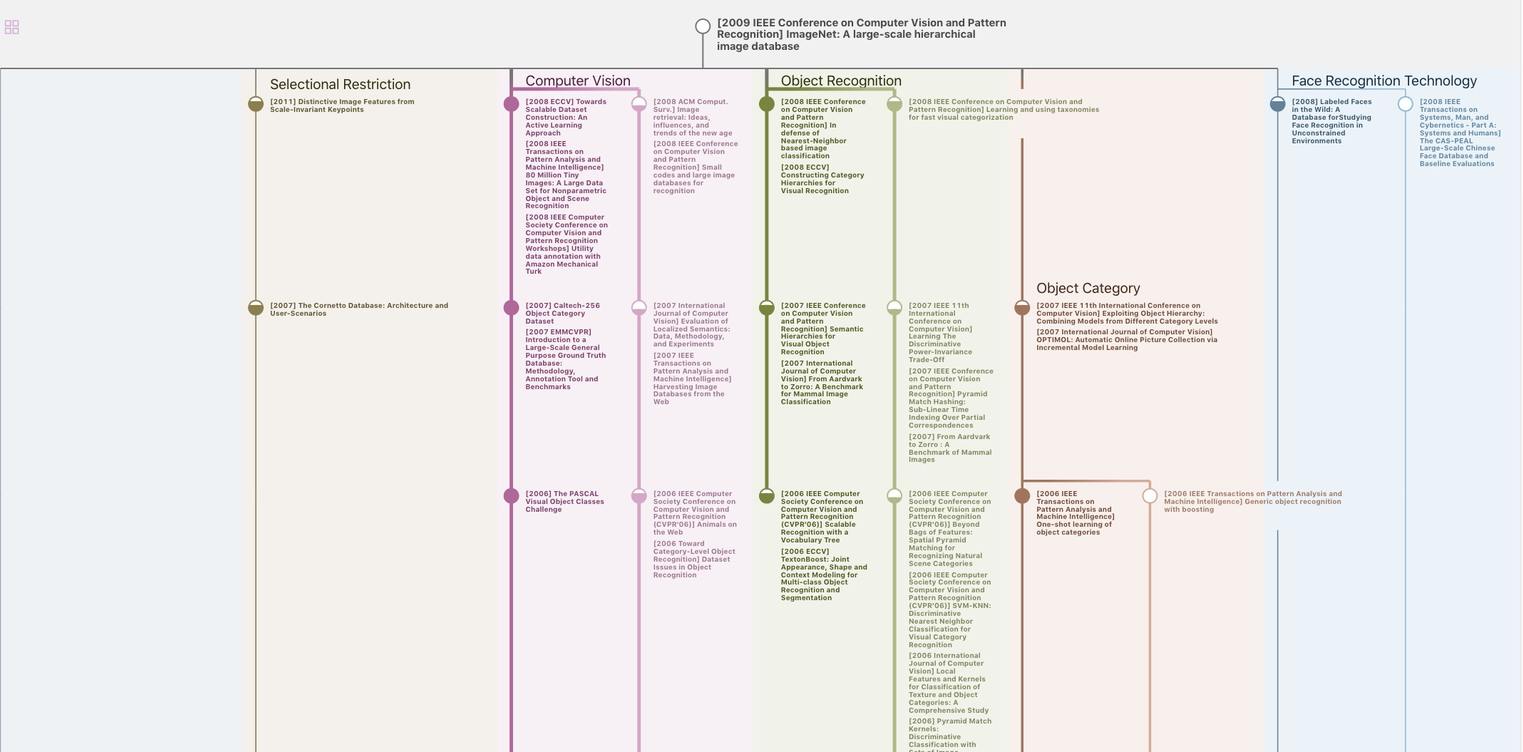
生成溯源树,研究论文发展脉络
Chat Paper
正在生成论文摘要