Soft Wearable Thermal Devices Integrated with Machine Learning
ADVANCED MATERIALS TECHNOLOGIES(2023)
摘要
Core body temperature (CBT) is a vital parameter that provides insight into individuals' overall health. However, existing methods to monitor CBT are mainly invasive and limited to applications in operating rooms. This work reports a soft wearable thermal device with low power operation to accurately monitor the core temperature and overcome these limitations. The thermal device comprises multiple temperature sensors separated with insulating materials of different thermal conductivities. The design provides a well-defined thermal gradient to characterize the heat flux across the device. Thermal simulation of the devices with finite element analysis provides guidelines on the device design. Experimental studies involving tissue phantom and human subjects characterize and validate the device performance. A machine learning approach can account for heterogeneous, hard-to-measure parameters among individuals such as tissue thermal conductivity and heat generation rate. The machine learning algorithms can be trained to accurately quantify the core temperature in human subjects using the zero-heat-flux device measured temperature as a reference. The results show that the mean core temperature difference between the zero-heat-flux and the devices is 0.01 degrees C with 95% limits of agreement in the range of -0.08 degrees C and 0.1 degrees C.
更多查看译文
关键词
soft wearable thermal devices,machine learning
AI 理解论文
溯源树
样例
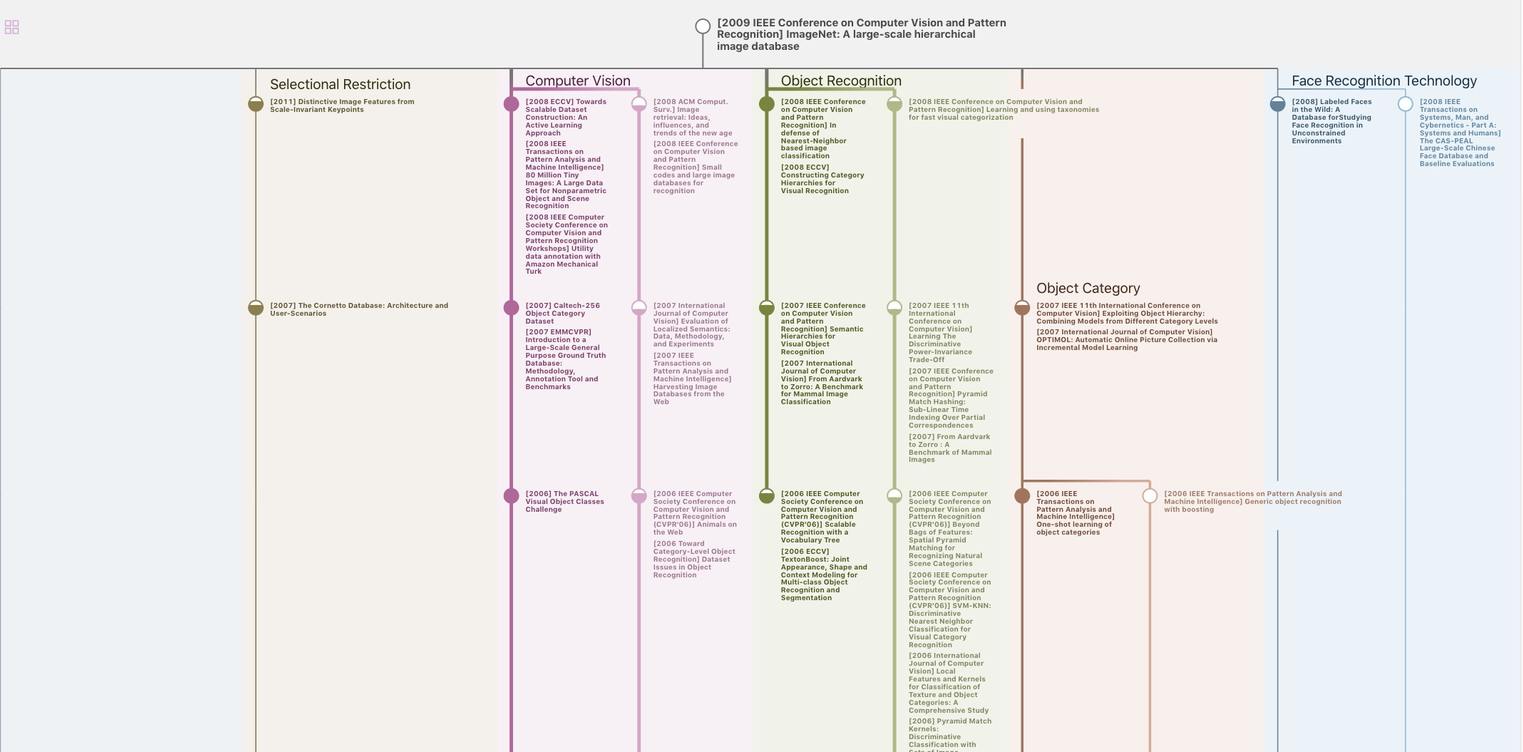
生成溯源树,研究论文发展脉络
Chat Paper
正在生成论文摘要