A feature extraction and deep learning approach for network traffic volume prediction considering detector reliability
COMPUTER-AIDED CIVIL AND INFRASTRUCTURE ENGINEERING(2024)
摘要
Accurate traffic volume prediction plays a crucial role in urban traffic control by relieving congestion through improved regulation of traffic volume. Network-level traffic volume prediction and detector failure have rarely been considered in the literature. This paper proposes a framework based on long short-term memory and the multilayer perceptron that can predict network-level traffic volumes even with detector failure. A profile model learns the profile of the detector's signature (traffic pattern). Detectors with similar profiles are considered to have similar traffic patterns and are grouped into a cluster. Failed detectors can obtain reference information from similar detectors in the same cluster without additional information. A predictive model is developed for each cluster. The proposed method is validated using Japan Road Traffic Information Center data for three cities. The computational results indicate that the proposed method performs well both on typical days and atypical days (the COVID-19 lockdown period and the 2021 Tokyo Olympics). Further, it considers detector reliability: the increase in mean absolute error is less than 1 veh/5 min when the probability of detector failure increases to 20%.
更多查看译文
AI 理解论文
溯源树
样例
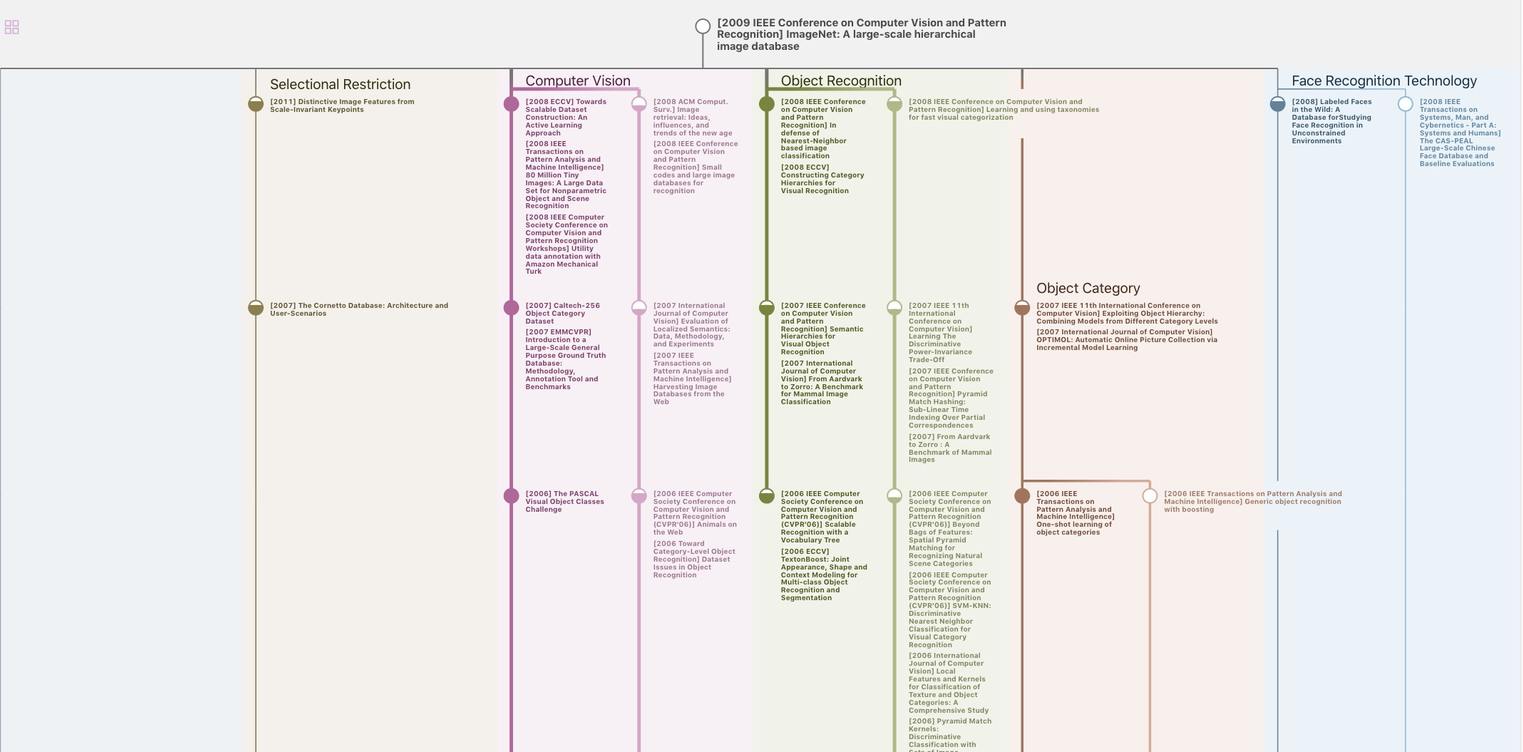
生成溯源树,研究论文发展脉络
Chat Paper
正在生成论文摘要