RPP-Net: Rigid Constrained Point Cloud Prediction Network
IEEE Transactions on Intelligent Transportation Systems(2023)
摘要
Forecasting the future environment is an essential and fundamental capability of autonomous driving systems. In contrast to the widely studied video prediction, only a few literatures have explored LiDAR point cloud prediction. To achieve future point cloud generation, most existing methods are based on free-form 3D scene flow prediction. However, these simple scene flow prediction-based methods may cause distortions since the motions of 3D scenes can be seen as a combination of rigid (static background) and flow (dynamic foreground) motions. To address this issue, we propose a simple but effective rigid constrained point cloud prediction network named RPP-Net. The key component of the proposed method is the hybrid motion decoder, which generates motion masks and combines flow motions and rigid motions to produce hybrid motions without additional annotations and sensors. Besides, to reduce running time, we provide a hierarchical cell, which uses a feature encoder to extract deep features and an RPP-RNN module to capture temporal correlations across frames. To evaluate the effectiveness of our RPP-Net, we have conducted extensive experiments on both KITTI dataset and Argoverse dataset and the results show that our RPP-Net significantly outperforms existing methods and achieves a new state-of-the-art.
更多查看译文
关键词
Point cloud,prediction,autonomous driving
AI 理解论文
溯源树
样例
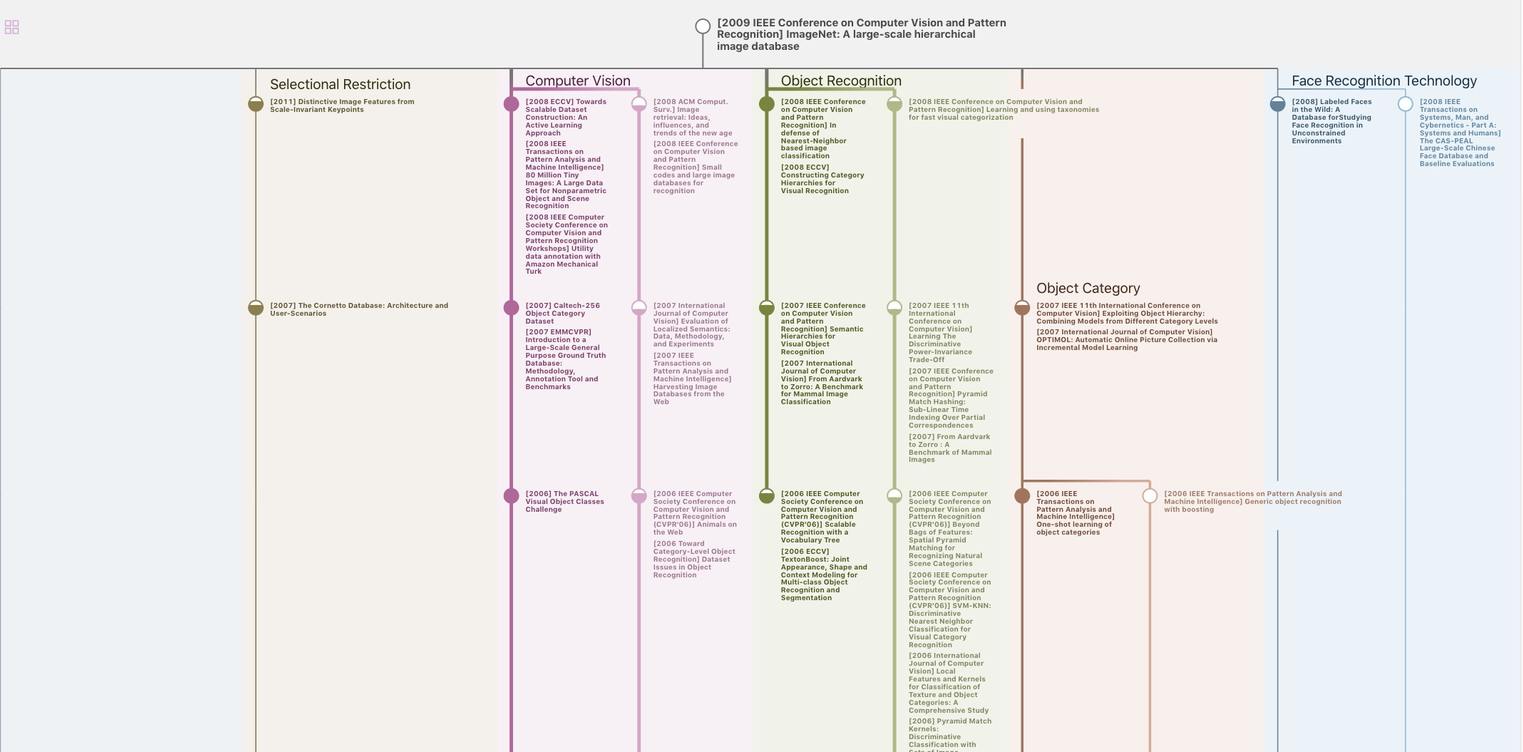
生成溯源树,研究论文发展脉络
Chat Paper
正在生成论文摘要