Automated valuation models: improving model performance by choosing the optimal spatial training level
JOURNAL OF PROPERTY RESEARCH(2023)
摘要
The academic community has discussed using Automated Valuation Models (AVMs) in the context of traditional real estate valuations and their performance for several decades. Most studies focus on finding the best method for estimating property values. One aspect that has not yet to be studied scientifically is the appropriate choice of the spatial training level. The published research on AVMs usually deals with a manually defined region and fails to test the methods used on different spatial levels. Our research aims to investigate the impact of training AVM algorithms at different spatial levels regarding valuation accuracy. We use a dataset with 1.2 million residential properties from Germany and test four methods: Ordinary Least Square, Generalised Additive Models, eXtreme Gradient Boosting and Deep Neural Network. Our results show that the right choice of spatial training level can significantly impact the model performance, and that this impact varies across the different methods.
更多查看译文
关键词
optimal spatial training level,models performance
AI 理解论文
溯源树
样例
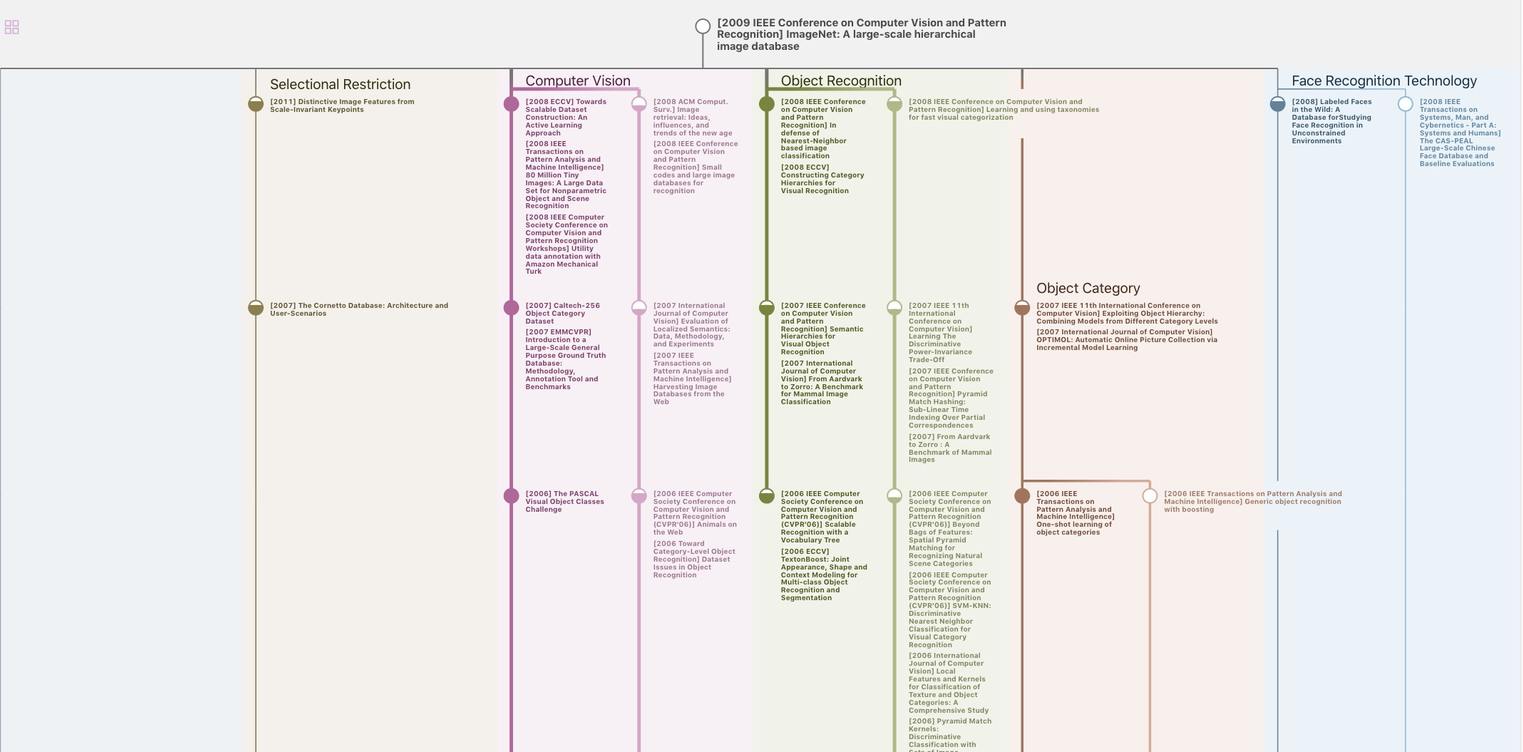
生成溯源树,研究论文发展脉络
Chat Paper
正在生成论文摘要