A multi-task learning method for multi-energy load forecasting based on synthesis correlation analysis and load participation factor
Applied Energy(2023)
摘要
Multi-energy load forecasting is the prerequisite for energy management and optimal scheduling of integrated energy systems (IES). Considering the randomness and coupling of multi-energy load demands, this paper proposes an IES multi-task learning (MTL) method for multi-energy load forecasting based on synthesis correlation analysis (SCA) and load participation factor (LPF). Firstly, SCA is proposed to screen multi-level features to maximize the key information in strong features and remove the prediction noise in weak features. Secondly, the LPF is proposed to describe the involvement of different loads in the total load, and the LPF application criteria of the factor are analyzed. Lastly, a MTL-LSTM integrated model considering total load forecasting is designed to deeply explore the hidden dynamic coupling information among different types of loads. The prediction model was tested on four seasons with data obtained from real-world scenarios and compared with existing prediction methods. The results show that the forecasting method constructed in this paper exhibits superior performance relative to other methods, and the average prediction accuracy achieve 97.18% for electricity, cooling, and heat loads, and 97.85% for the total load.
更多查看译文
关键词
Integrated energy systems,Load forecasting,Multi-task learning,Load participation factor,Synthesis correlation analysis
AI 理解论文
溯源树
样例
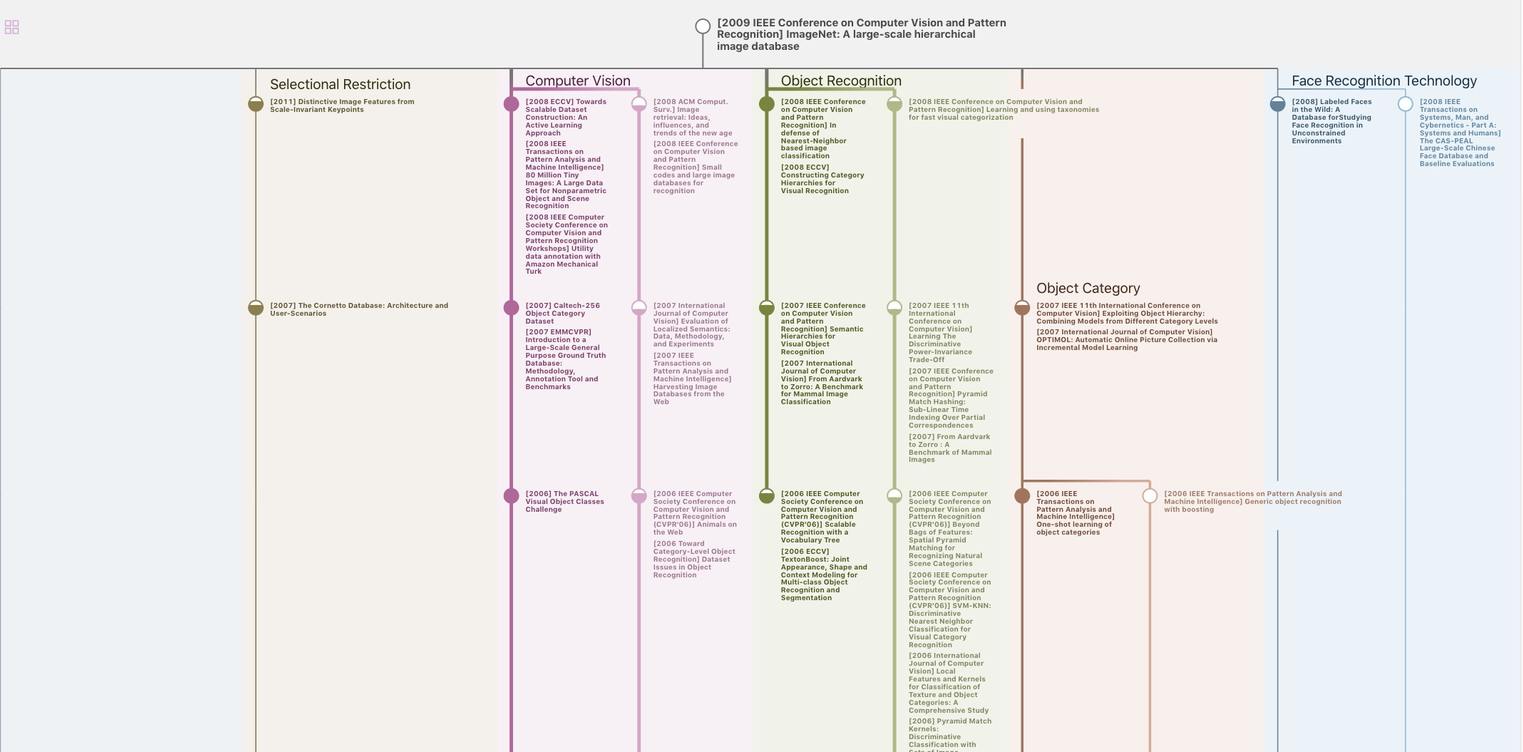
生成溯源树,研究论文发展脉络
Chat Paper
正在生成论文摘要