A Novel Hybrid Artificial Bee Colony-Based Deep Convolutional Neural Network to Improve the Detection Performance of Backscatter Communication Systems
ELECTRONICS(2023)
摘要
Backscatter communication (BC) is a promising technology for low-power and low-data-rate applications, though the signal detection performance is limited since the backscattered signal is usually much weaker than the original signal. When the detection performance is poor, the backscatter device (BD) may not be able to accurately detect and interpret the incoming signal, leading to errors and degraded communication quality. This can result in data loss, slow data transfer rates, and reduced reliability of the communication link. This paper proposes a novel approach to improve the detection performance of backscatter communication systems using evolutionary deep learning. In particular, we focus on training deep convolutional neural networks (DCNNs) to improve the detection performance of BC. We first develop a novel hybrid algorithm based on artificial bee colony (ABC), biogeography-based optimization (BBO), and particle swarm optimization (PSO) to optimize the architecture of the DCNN, followed by training using a large set of benchmark datasets. To develop the hybrid ABC, the migration operator of the BBO is used to improve the exploitation. Moving towards the global best of PSO is also proposed to improve the exploration of the ABC. Then, we take advantage of the proposed deep architecture to improve the bit-error rate (BER) performance of the studied BC system. The simulation results demonstrate that the proposed algorithm has the best performance in training the benchmark datasets. The results also show that the proposed approach significantly improves the detection performance of backscattered signals compared to existing works.
更多查看译文
关键词
backscatter communication,detection performance,bit-error rate,deep convolutional neural network,hybrid artificial bee colony
AI 理解论文
溯源树
样例
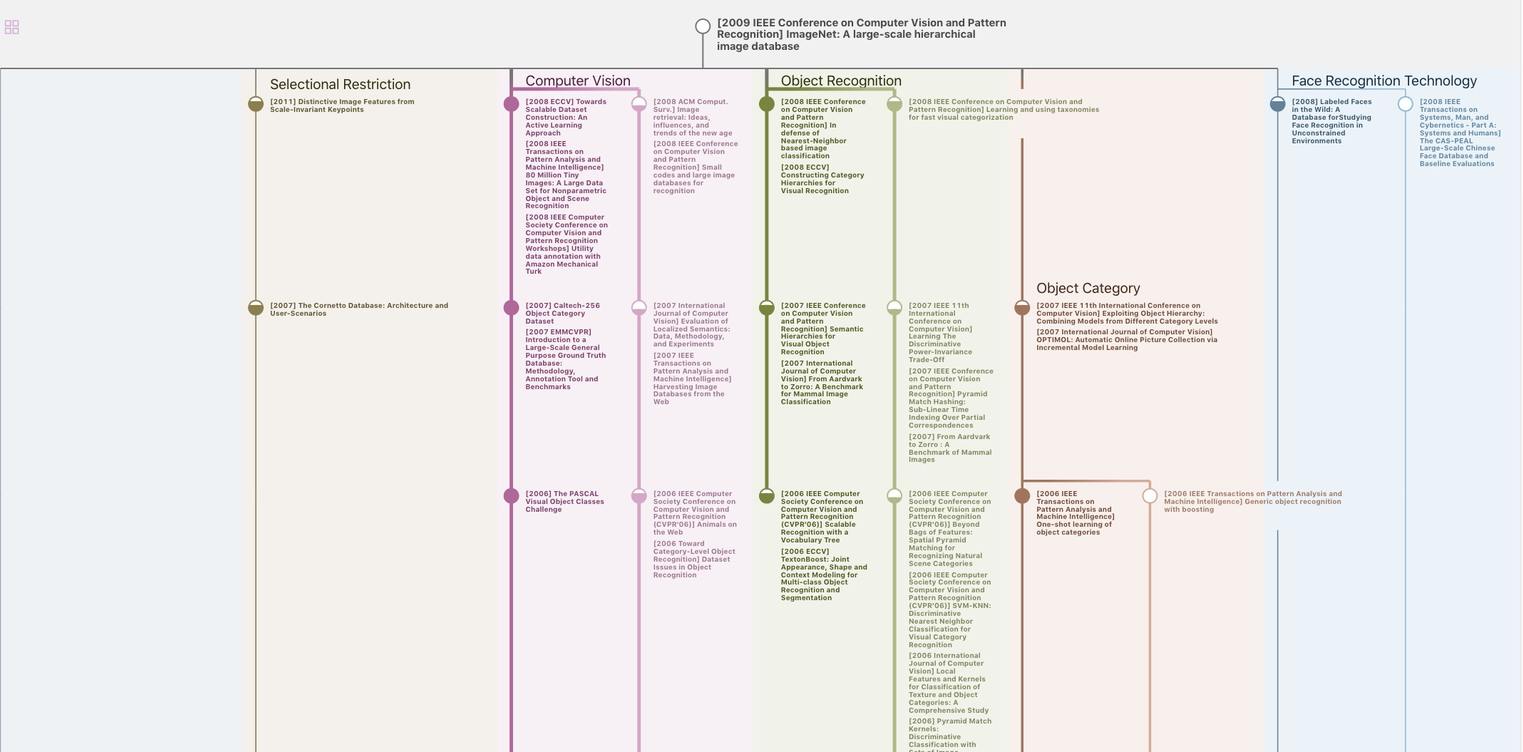
生成溯源树,研究论文发展脉络
Chat Paper
正在生成论文摘要