Remote sensing monitoring of wheat leaf rust based on UAV multispectral imagery and the BPNN method
FOOD AND ENERGY SECURITY(2023)
摘要
Wheat (Triticum aestivum L.) leaf rust is the most common and widely distributed wheat disease. Non-destructive and real-time methods for monitoring wheat leaf rust can help prevent and control plant diseases in agricultural production. In this study, we obtained multispectral imagery of the wheat canopy acquired by an unmanned aerial vehicle, selected the vegetation index using the K-means algorithm (KA) and genetic algorithm (GA), and established a wheat leaf rust monitoring model based on the backpropagation neural network (BPNN) method. The results showed that the R-2 and RMSE of the KA-BPNN model were 0.902% and 5.45% for the modeling set, respectively, and 0.784% and 4.76% for the validation set, respectively; and the R-2 and RMSE of the GA-BPNN model was 0.922% and 4.88% for the modeling set, respectively, and 0.780% and 4.28% for the validation set, respectively. The prediction model after optimizing the variables using KA and GA had higher accuracy than the BPNN model, implying that using variable dimensionality reduction methods and complex machine learning algorithms to construct estimation models can improve model accuracy significantly. These models accurately monitored leaf rust in winter wheat, providing a theoretical basis and technical support for assessing plant diseases and screening disease-resistant wheat varieties.
更多查看译文
关键词
backpropagation neural network, multispectral imagery, spectral reflectance, vegetation index, wheat leaf rust
AI 理解论文
溯源树
样例
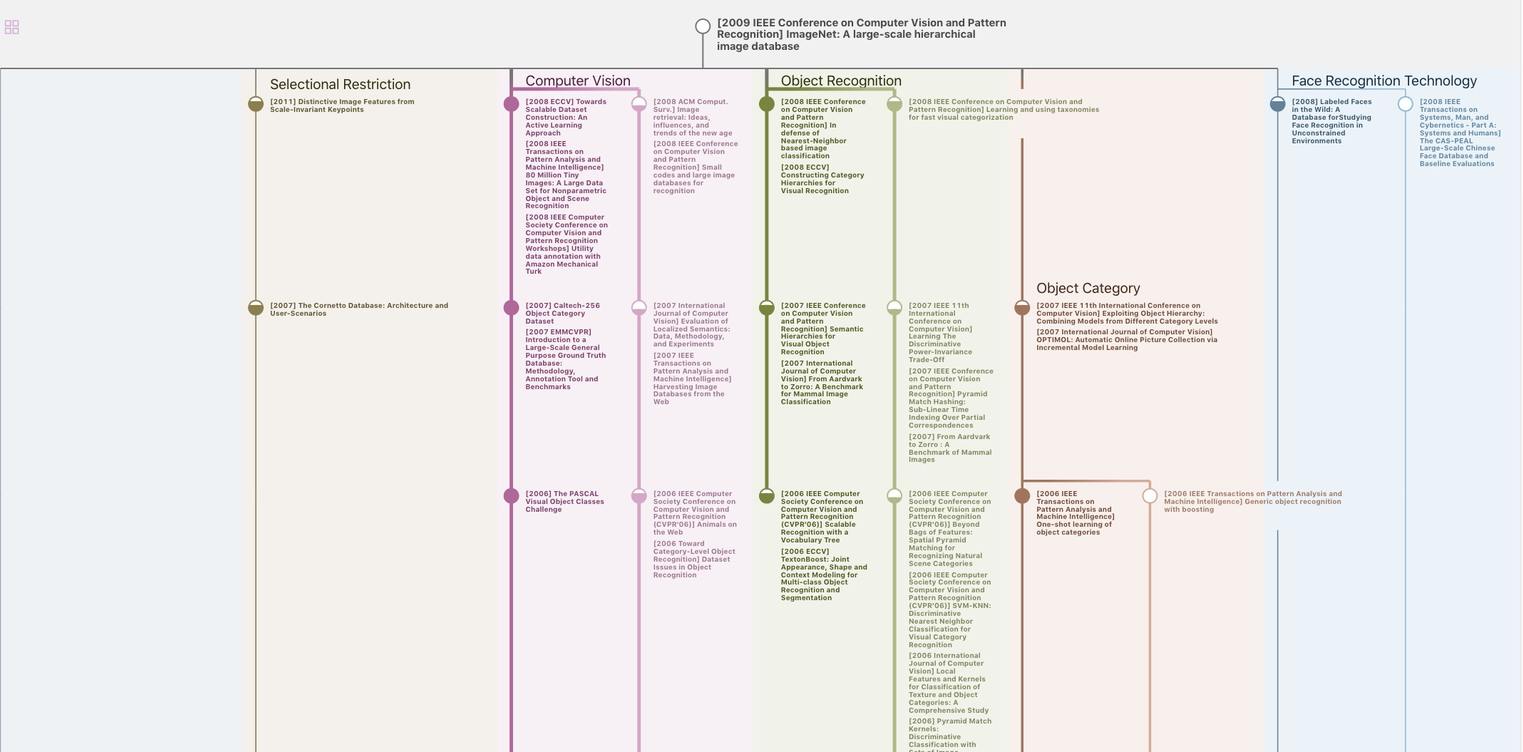
生成溯源树,研究论文发展脉络
Chat Paper
正在生成论文摘要