A deep learning approach for detecting covert timing channel attacks using sequential data
CLUSTER COMPUTING-THE JOURNAL OF NETWORKS SOFTWARE TOOLS AND APPLICATIONS(2024)
摘要
The advanced development of communication technologies has made covert communications quite challenging to be recognized. By altering an entity's timing behavior during overt network communication, a covert timing channel (CTC) provides a way to leak sensitive data. This is becoming a serious threat due to the ability to transmit hidden messages without being detected by traditional security systems such as proxies and firewalls. In this paper, we explore the automatic classification and identification of covert timing channels using deep neural networks, namely, Long Short-Term Memory (LSTM), 1D-Convolutional Neural networks (1D-CNN), and a hybrid of LSTM and 1D-CNN. These classifiers have been trained and tested using sequence real inter-arrival times datasets labeled with covert or legitimate. The stream length differs for each dataset; (32, 64, 128, 256, and 512 bytes). Traditional machine-learning models have also been developed for comparisons and evaluation. The evaluation results showed that the hybrid of LSTM and CNN model outperforms other models either developed by deep neural networks or traditional machine learning with an accuracy of %97.5.
更多查看译文
关键词
Covert timing channels,Cybersecurity,Deep neural networks,Machine learning
AI 理解论文
溯源树
样例
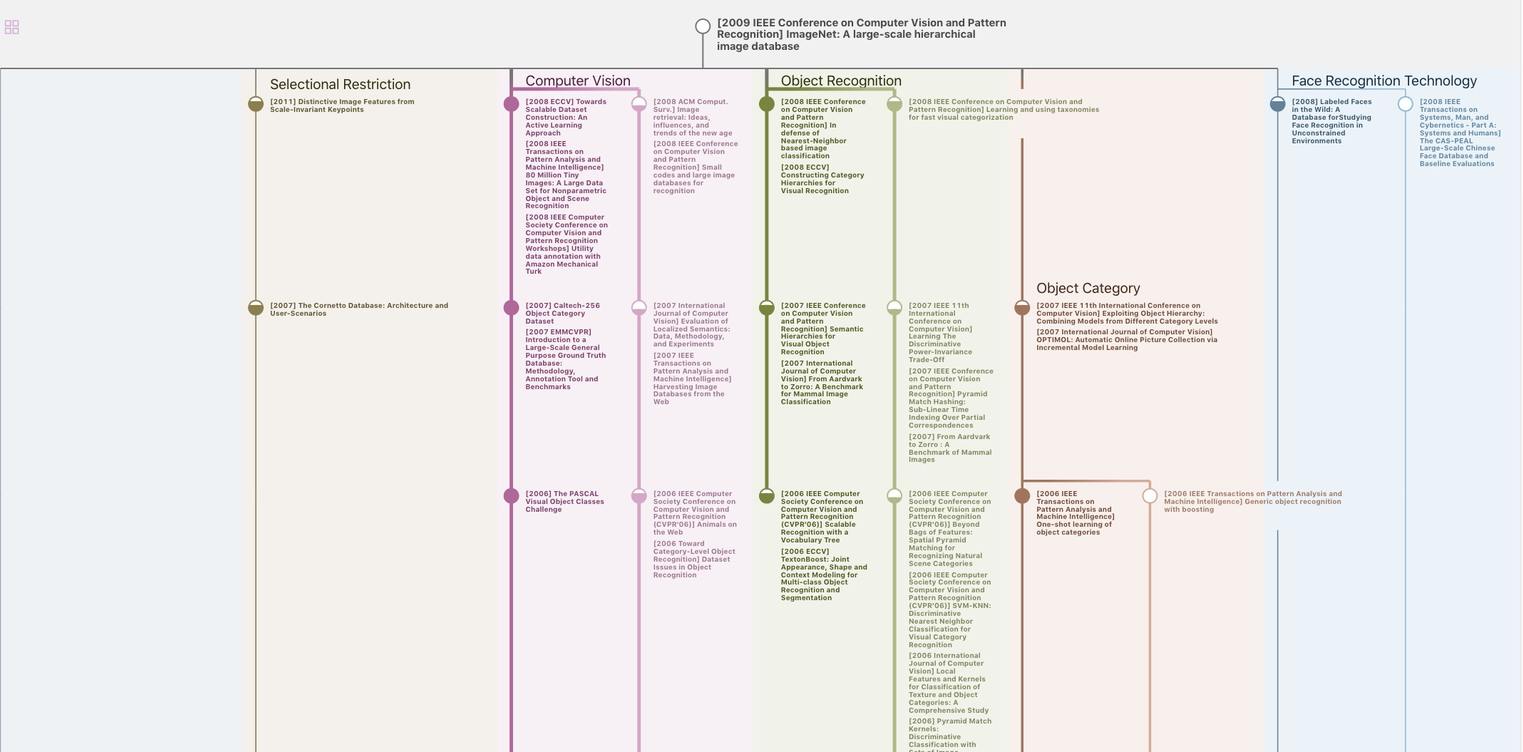
生成溯源树,研究论文发展脉络
Chat Paper
正在生成论文摘要