Exploring better target for shadow detection
Knowledge-Based Systems(2023)
摘要
Shadow detection aims to identify shadow regions from images, which plays a significant role in scene understanding. Existing approaches tend to ignore the annotation noises in ground truths, which will be overfitted in the later training phase and potentially degrade detection performance. To alleviate the impact of such noisy labels, this work proposes a framework for robust shadow detection (RSD) by locating and correcting them. Specifically, we first introduce a noise-rate blind sample selection scheme based on the prediction-level stability to identify the reliable parts from all pixel-level samples. Next, we design a label correction strategy based on the graph convolutional network, which can propagate the label information between reliable and unreliable parts. Finally, we enable subsequent robust learning by using a new training target with fewer noisy labels for each image. Experimental results on public benchmarks (i.e., SBU, ISTD, UCF and CUHK-Shadow) show that our method can be favorable against SOTAs. Our source code is available at https://github.com/wuwen1994/RSD.
更多查看译文
关键词
Image segmentation,Shadow detection,Noisy label,Robust learning,Graph convolutional network
AI 理解论文
溯源树
样例
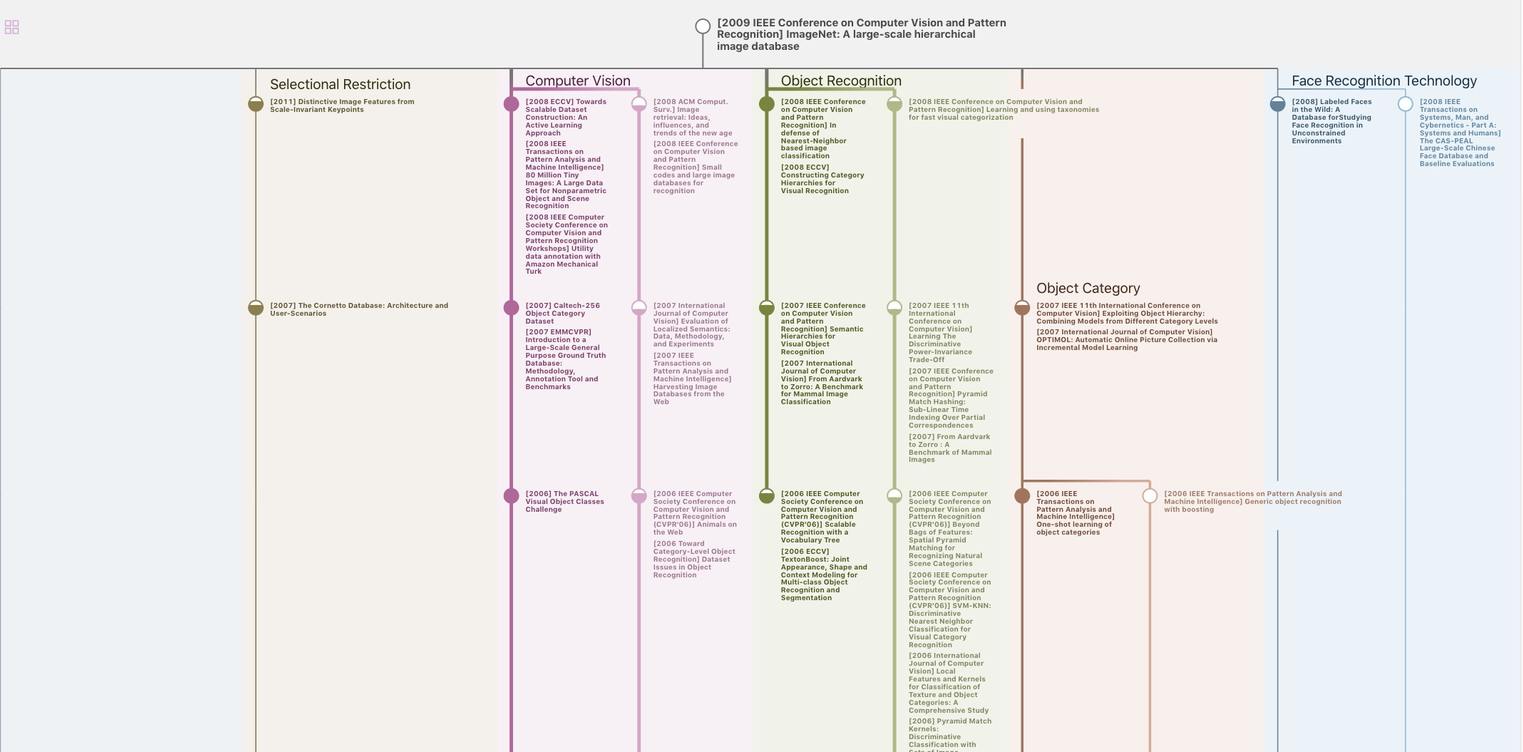
生成溯源树,研究论文发展脉络
Chat Paper
正在生成论文摘要