A study of variation in dynamical behavior of fractional complex Ginzburg-Landau model for different fractional operators
PATTERN RECOGNITION LETTERS(2023)
摘要
Recently, the Transformers have shown great potential in computer vision tasks, such as classification detection, segmentation , image synthesis, etc. The success of Transformers has been long attributed to the attention-based token mixer. However, the computational complexity of the attention-based token mixer module is quadratic to the number of tokens to be mixed. Therefore, the attention-based token mixer module requires more parameters , will cause a very large amount of computation. As far as image synthesis task is concerned, the attention-based token mixer module increases the computation amount of generative adversarial networks (GANs) based on Transformers. To address this problem, we propose the PFGAN method. The motivation is based on our observation that the computational com-plexity of pooling is linear to the sequence length, without any other learnable parameters. Based on this observation, we use pooling rather than self-attention as the token mixer. Experimental results on CelebA, CIFAR-10 and LSUN datasets demonstrate that our proposed method has fewer parameters and fewer computational complexity. (c) 2023 Elsevier B.V. All rights reserved.
更多查看译文
关键词
Transformers,Generative adversarial networks,Token mixer,Pooling
AI 理解论文
溯源树
样例
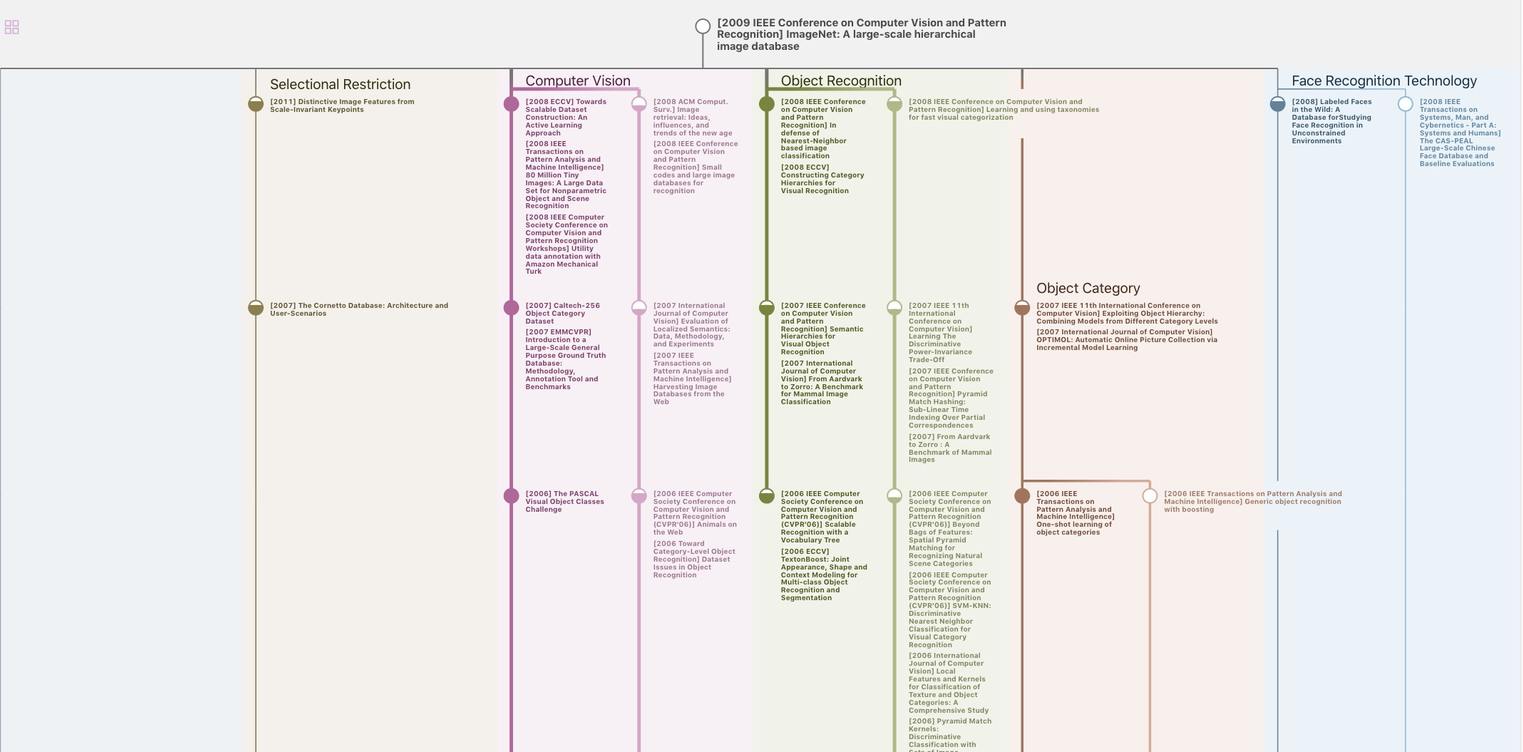
生成溯源树,研究论文发展脉络
Chat Paper
正在生成论文摘要