Multiple kernel clustering with structure-preserving and block diagonal property
MULTIMEDIA TOOLS AND APPLICATIONS(2024)
摘要
It is well known that graph-based multiple kernel clustering (GMKC) methods improve the clustering performance by integrating multiple kernel learning and graph-based clustering. However, existing GMKC methods either do not consider the global and local structure of data in kernel space simultaneously, or ignore block diagonal property of the affinity matrix, thus impairing the final clustering performance greatly. To address this issue, in this paper we propose a novel method named multiple kernel clustering with structure-preserving and block diagonal property (SBDMKC) by combining GMKC and block diagonal regularizer. Typically, the local structure-preserving regularization term is an accurate measurement for the similarity between data in kernel space, rather than original space. Furthermore, the affinity matrix is encouraged to be block diagonal by a soft regularizer, which helps to achieve good data clustering. In addition, a simple kernel weight strategy is given, which can automatically weight each base kernel to find an optimal consensus kernel. Experimental results on the ten benchmark data sets show that our method outperforms the nine state-of-the-art clustering methods.
更多查看译文
关键词
Multiple kernel clustering,Block diagonal representation,Structure preserving
AI 理解论文
溯源树
样例
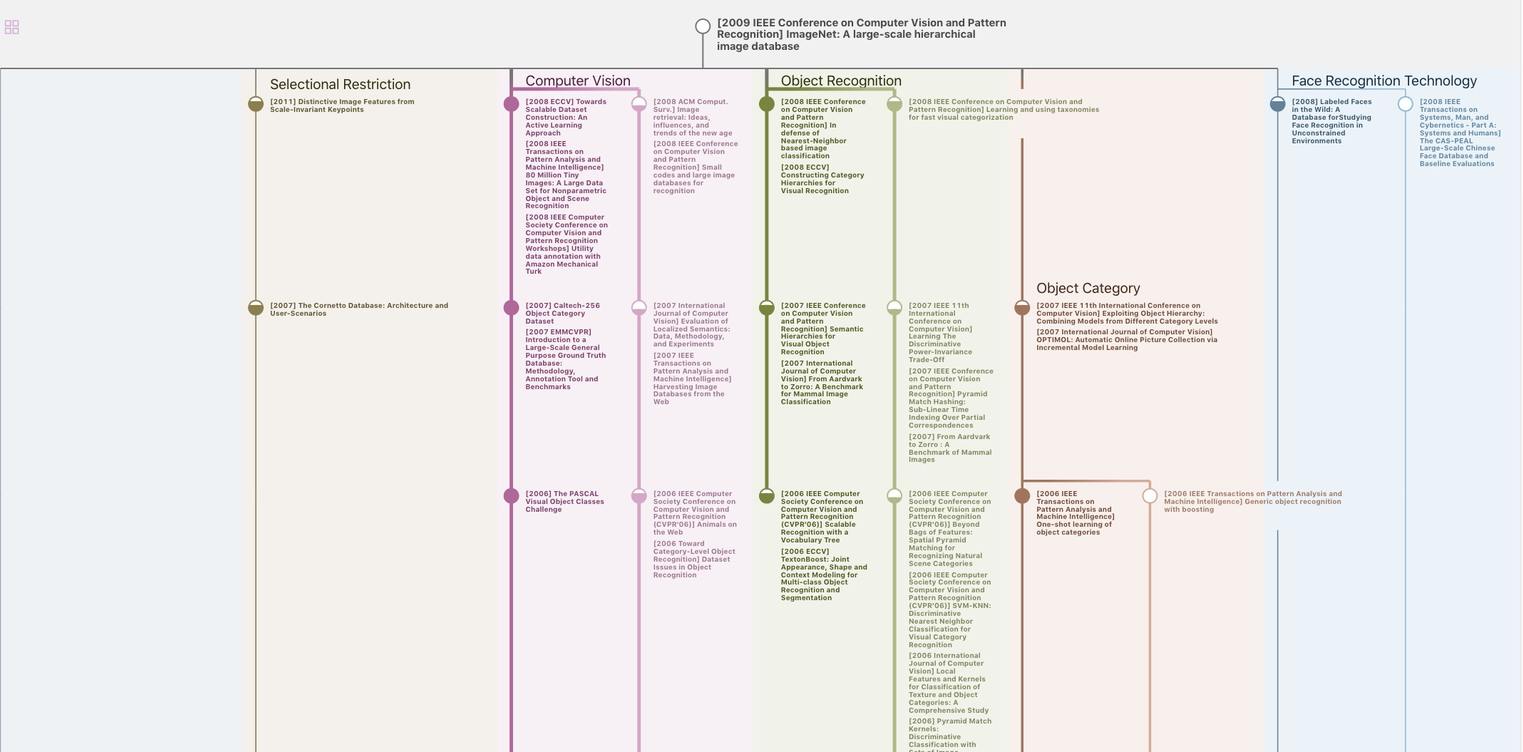
生成溯源树,研究论文发展脉络
Chat Paper
正在生成论文摘要