Optimising Robot Swarm Formations by Using Surrogate Models and Simulations
APPLIED SCIENCES-BASEL(2023)
摘要
Optimising a swarm of many robots can be computationally demanding, especially when accurate simulations are required to evaluate the proposed robot configurations. Consequentially, the size of the instances and swarms must be limited, reducing the number of problems that can be addressed. In this article, we study the viability of using surrogate models based on Gaussian processes and artificial neural networks as predictors of the robots' behaviour when arranged in formations surrounding a central point of interest. We have trained the surrogate models and tested them in terms of accuracy and execution time on five different case studies comprising three, five, ten, fifteen, and thirty robots. Then, the best performing predictors combined with ARGoS simulations have been used to obtain optimal configurations for the robot swarm by using our proposed hybrid evolutionary algorithm, based on a genetic algorithm and a local search. Finally, the best swarm configurations obtained have been tested on a number of unseen scenarios comprising different initial robot positions to evaluate the robustness and stability of the achieved robot formations. The best performing predictors exhibited speed increases of up to 3604 with respect to the ARGoS simulations. The optimisation algorithm converged in 91% of runs and stable robot formations were achieved in 79% of the unseen testing scenarios.
更多查看译文
关键词
robot swarm formations,surrogate models,simulations
AI 理解论文
溯源树
样例
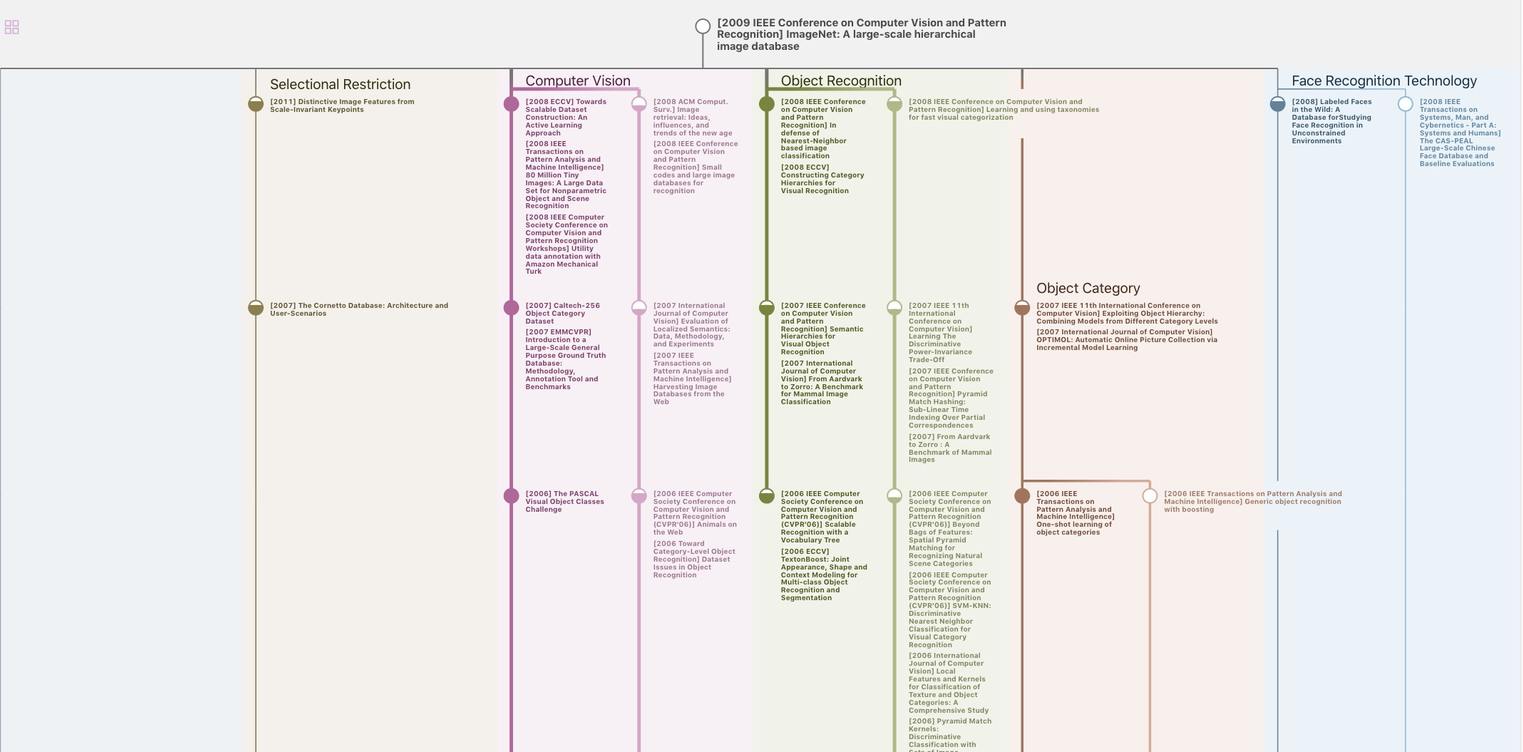
生成溯源树,研究论文发展脉络
Chat Paper
正在生成论文摘要